Towards Globalised Models for Exercise Classification using Inertial Measurement Units
2023 IEEE 19th International Conference on Body Sensor Networks (BSN)(2023)
摘要
Wearable sensors are becoming a popular method of objectively evaluating motor performance in various exercise tasks. A challenge in working with this motion capture data is the personalised nature of the data where one individual's data may be different to that of others due to factors such as differences in exercise form. Hence global models generally perform poorly in this domain. The aim of this study is to investigate a technique to push personalised classification models into global models. In this research, a dataset of Inertial Measurement Unit data consisting of fatigued and non-fatigued running is used to employ a clustering strategy where participants are grouped together using a hierarchical clustering methodology. These clusters are used to form semi-globalised models. The investigation shows that this strategy can improve the global model performance by up to 20%.
更多查看译文
关键词
Wearable Sensors,Time Series Classification,Fatigue Prediction
AI 理解论文
溯源树
样例
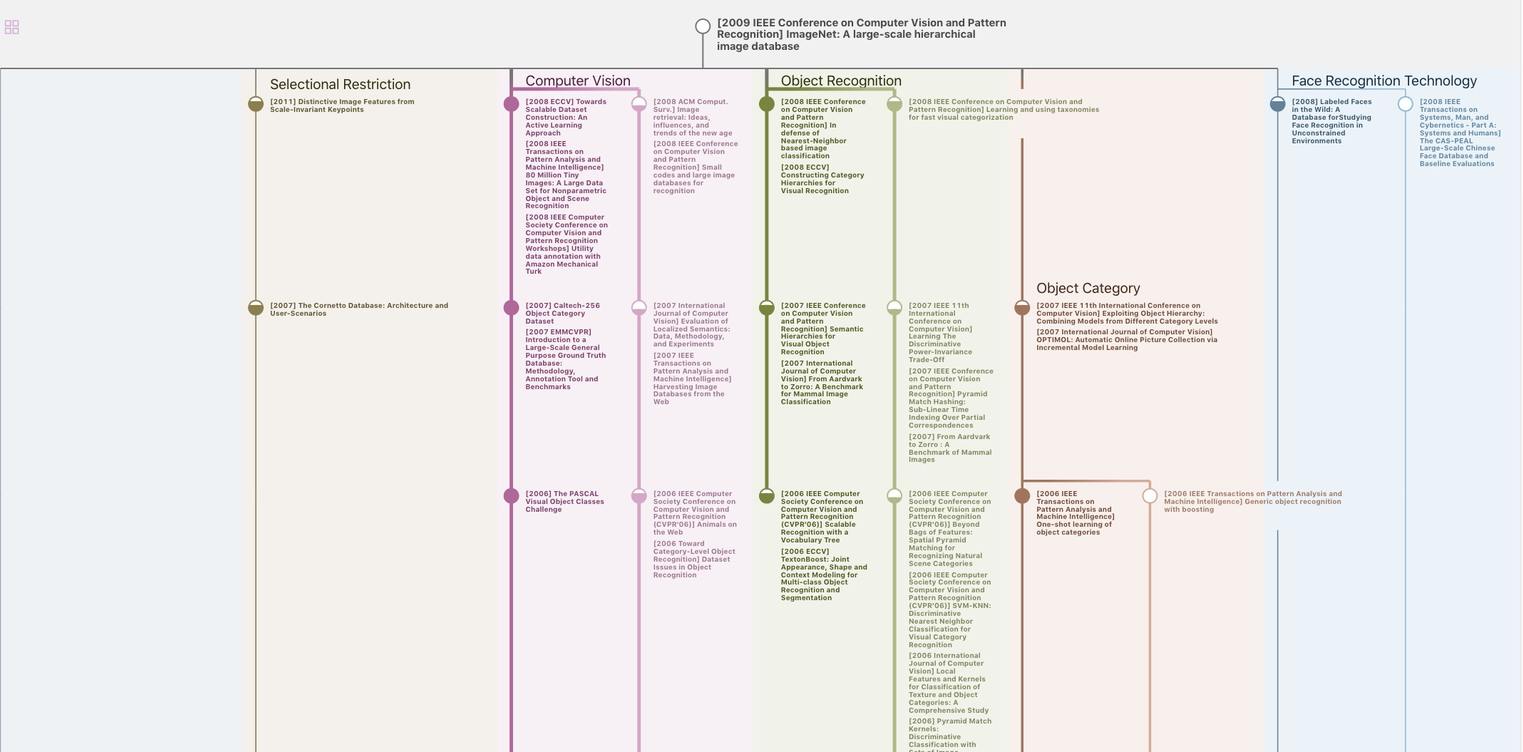
生成溯源树,研究论文发展脉络
Chat Paper
正在生成论文摘要