Corner-to-Center long-range context model for efficient learned image compression
JOURNAL OF VISUAL COMMUNICATION AND IMAGE REPRESENTATION(2024)
摘要
In the framework of learned image compression, the context model plays a pivotal role in capturing the de-pendencies among latent representations. To reduce the decoding time resulting from the serial autoregressive context model, the parallel context model has been proposed as an alternative that necessitates only two passes during the decoding phase, thus facilitating efficient image compression in real-world scenarios. However, performance degradation occurs due to its incomplete casual context. To tackle this issue, we conduct an in-depth analysis of the performance degradation observed in existing parallel context models, focusing on two aspects: the Quantity and Quality of information utilized for context prediction and decoding. Based on such analysis, we propose the Corner-to-Center transformer-based Context Model symbolscript designed to enhance context and latent predictions and improve rate-distortion performance. Specifically, we leverage the logarithmic-based prediction order to predict more context features from corner to center progressively. In addition, to enlarge the receptive field in the analysis and synthesis transformation, we use the Long-range Crossing Attention Module (LCAM) in the encoder/decoder to capture the long-range semantic information by assigning the different window shapes in different channels. Extensive experimental evaluations show that the proposed method is effective and outperforms the state-of-the-art parallel methods. Finally, according to the subjective analysis, we suggest that improving the detailed representation in transformer-based image compression is a promising direction to be explored.
更多查看译文
关键词
Learned image compression,Context model,Transformer
AI 理解论文
溯源树
样例
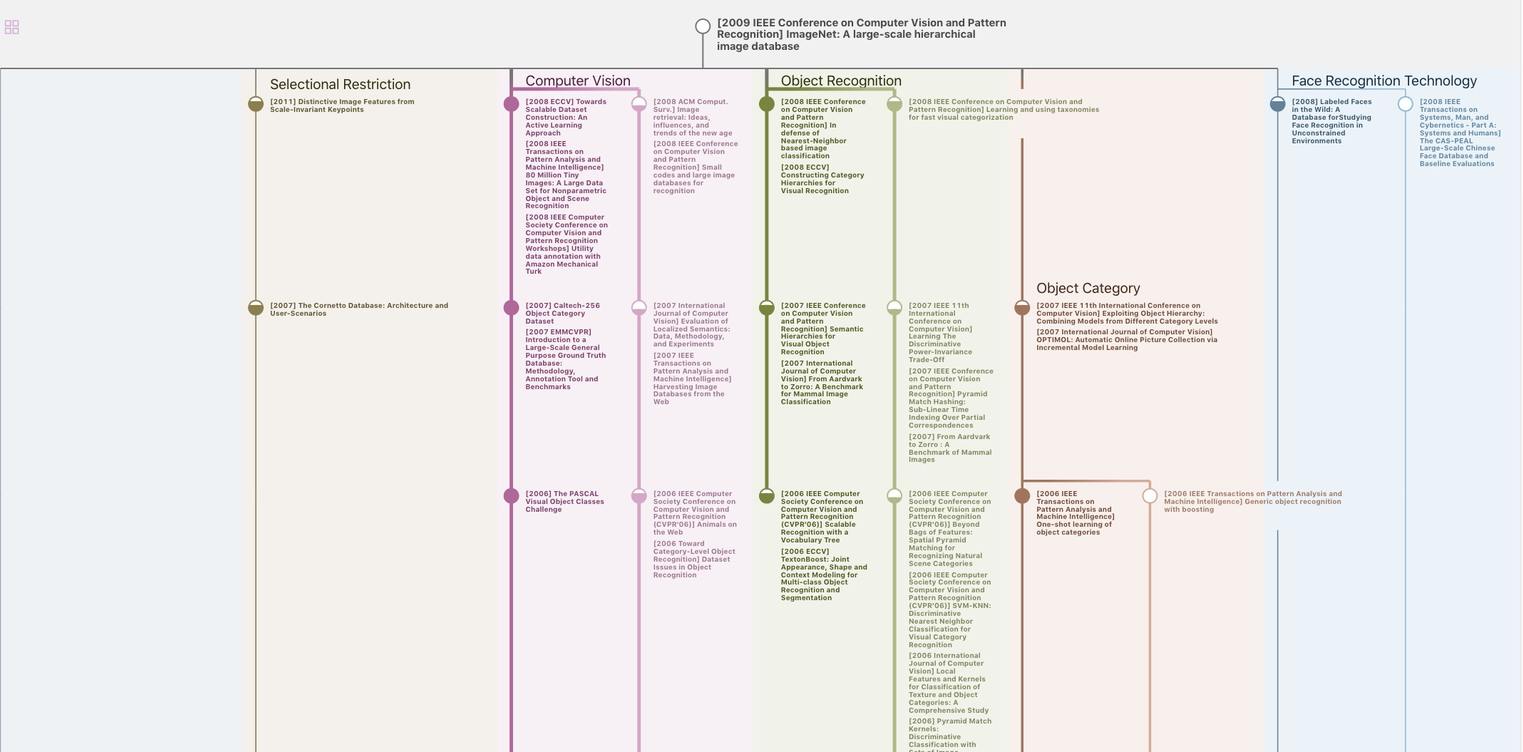
生成溯源树,研究论文发展脉络
Chat Paper
正在生成论文摘要