Attribute Simulation for Item Embedding Enhancement in Multi-interest Recommendation
PROCEEDINGS OF THE 17TH ACM INTERNATIONAL CONFERENCE ON WEB SEARCH AND DATA MINING, WSDM 2024(2024)
摘要
Our research reveals that multi-interest recommendation models in the matching stage tend to exhibit an under-clustered item embedding space, which leads to a low discernibility between items and hampers item retrieval. This highlights the necessity for item embedding enhancement. However, item attributes, which serve as effective side information for enhancement, are either unavailable or incomplete in many public datasets due to the labor-intensive nature of manual annotation tasks. This dilemma raises two meaningful questions: 1. Can we bypass manual annotation and directly simulate complete attribute information from the interaction data? And 2. If feasible, howcanwe simulate attributes with high accuracy and low complexity in the matching stage? In this paper, we first establish a theoretical feasibility that the item-attribute correlation matrix can be approximated through elementary transformations on the item co-occurrence matrix. Then, based on further formula derivation, we propose a simple yet effective module, SimEmb (Item Embedding Enhancement via Simulated Attribute), in the multi-interest recommendation of the matching stage. By simulating attributes with the co-occurrence matrix, SimEmb discards the ID-based item embedding and employs the attribute-weighted summation for item embedding enhancement. Comprehensive experiments on four benchmark datasets demonstrate that our approach notably enhances the clustering of item embedding and significantly outperforms SOTA models with an average improvement of 25.59% on Recall@20.
更多查看译文
关键词
Recommender Systems,Multi-interest Learning,Attribute Simulation,Item Embedding Enhancement
AI 理解论文
溯源树
样例
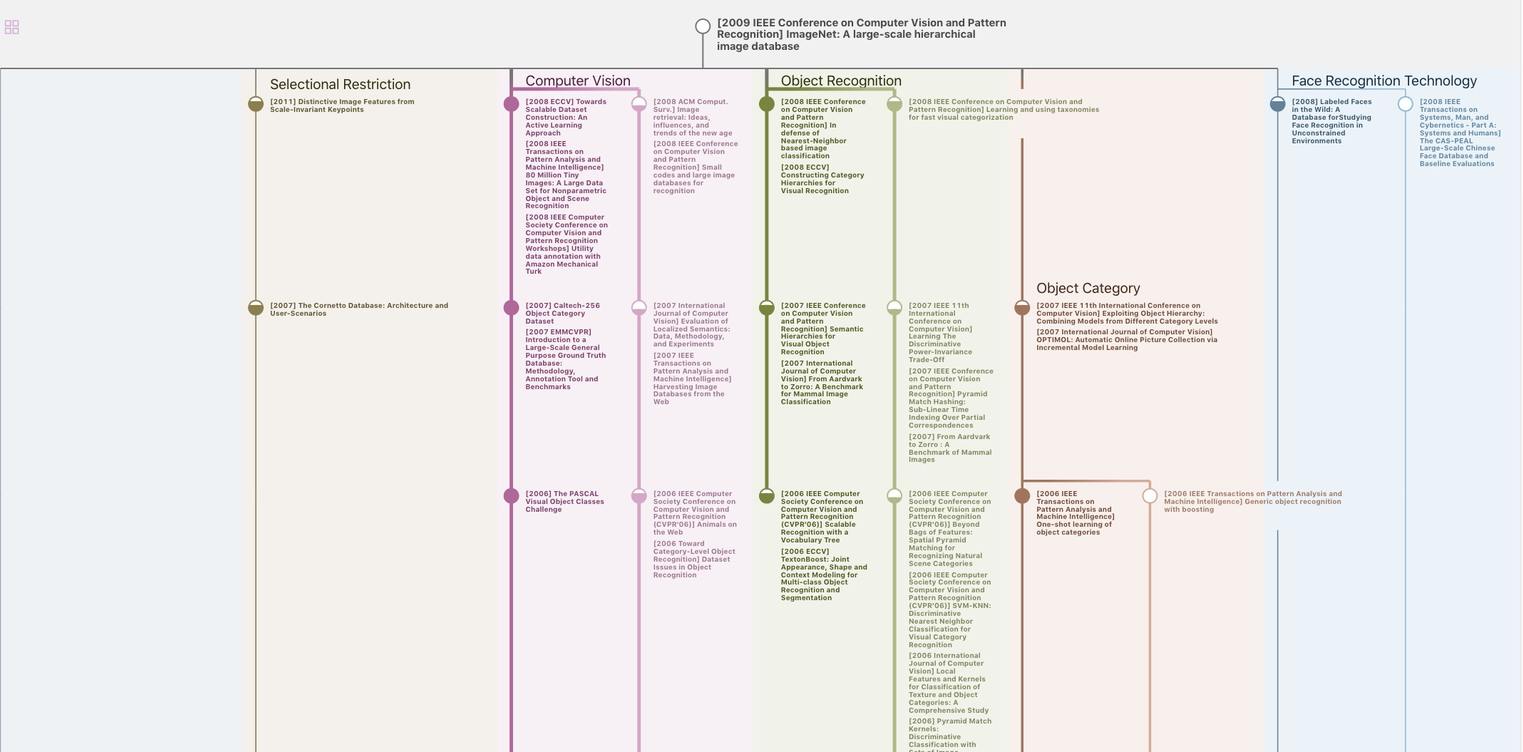
生成溯源树,研究论文发展脉络
Chat Paper
正在生成论文摘要