Ignore This Title and HackAPrompt: Exposing Systemic Vulnerabilities of LLMs through a Global Scale Prompt Hacking Competition
arxiv(2023)
摘要
Large Language Models (LLMs) are deployed in interactive contexts with direct
user engagement, such as chatbots and writing assistants. These deployments are
vulnerable to prompt injection and jailbreaking (collectively, prompt hacking),
in which models are manipulated to ignore their original instructions and
follow potentially malicious ones. Although widely acknowledged as a
significant security threat, there is a dearth of large-scale resources and
quantitative studies on prompt hacking. To address this lacuna, we launch a
global prompt hacking competition, which allows for free-form human input
attacks. We elicit 600K+ adversarial prompts against three state-of-the-art
LLMs. We describe the dataset, which empirically verifies that current LLMs can
indeed be manipulated via prompt hacking. We also present a comprehensive
taxonomical ontology of the types of adversarial prompts.
更多查看译文
AI 理解论文
溯源树
样例
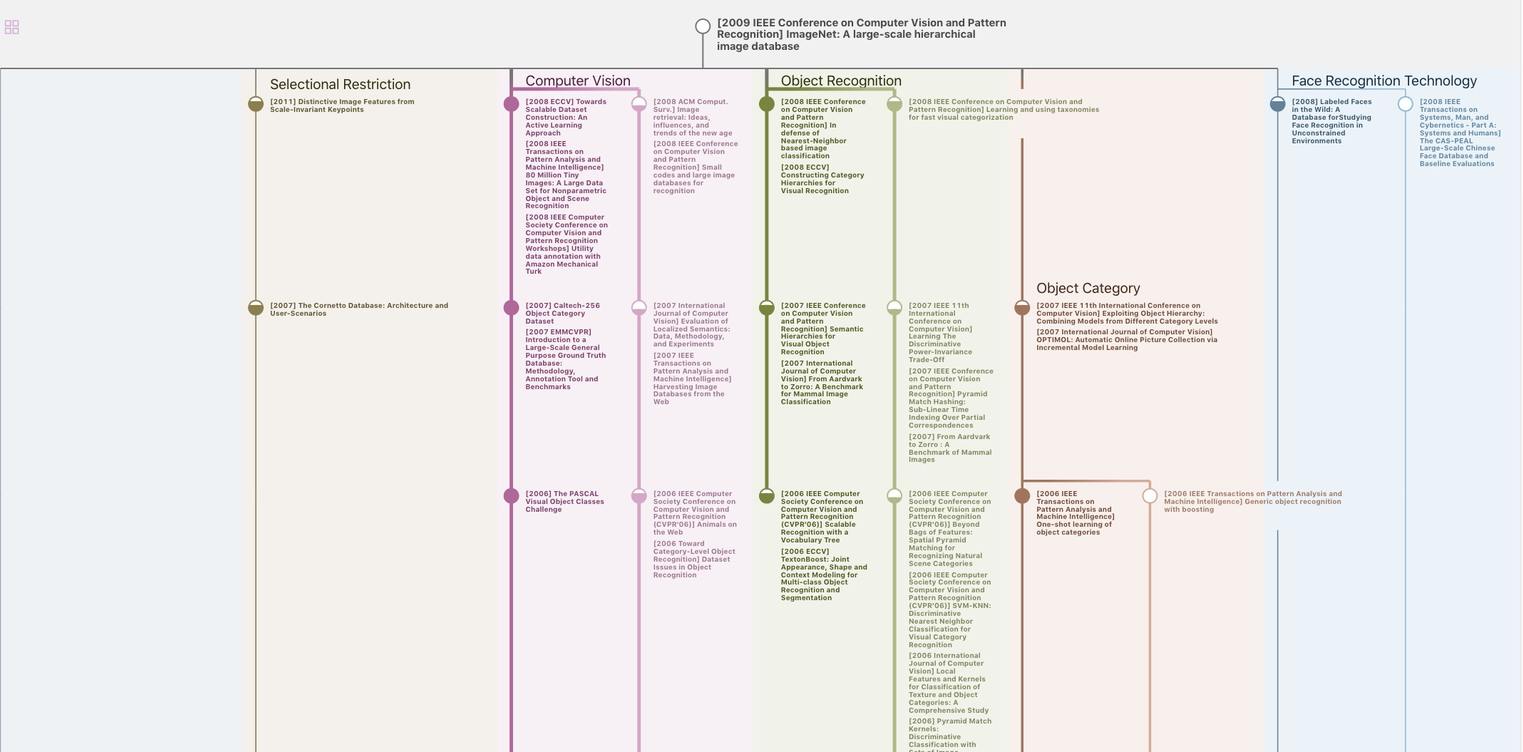
生成溯源树,研究论文发展脉络
Chat Paper
正在生成论文摘要