Query by Activity Video in the Wild.
CoRR(2023)
摘要
This paper focuses on activity retrieval from a video query in an imbalanced
scenario. In current query-by-activity-video literature, a common assumption is
that all activities have sufficient labelled examples when learning an
embedding. This assumption does however practically not hold, as only a portion
of activities have many examples, while other activities are only described by
few examples. In this paper, we propose a visual-semantic embedding network
that explicitly deals with the imbalanced scenario for activity retrieval. Our
network contains two novel modules. The visual alignment module performs a
global alignment between the input video and fixed-sized visual bank
representations for all activities. The semantic module performs an alignment
between the input video and fixed-sized semantic activity representations. By
matching videos with both visual and semantic activity representations that are
of equal size over all activities, we no longer ignore infrequent activities
during retrieval. Experiments on a new imbalanced activity retrieval benchmark
show the effectiveness of our approach for all types of activities.
更多查看译文
关键词
activity video search,imbalance learning
AI 理解论文
溯源树
样例
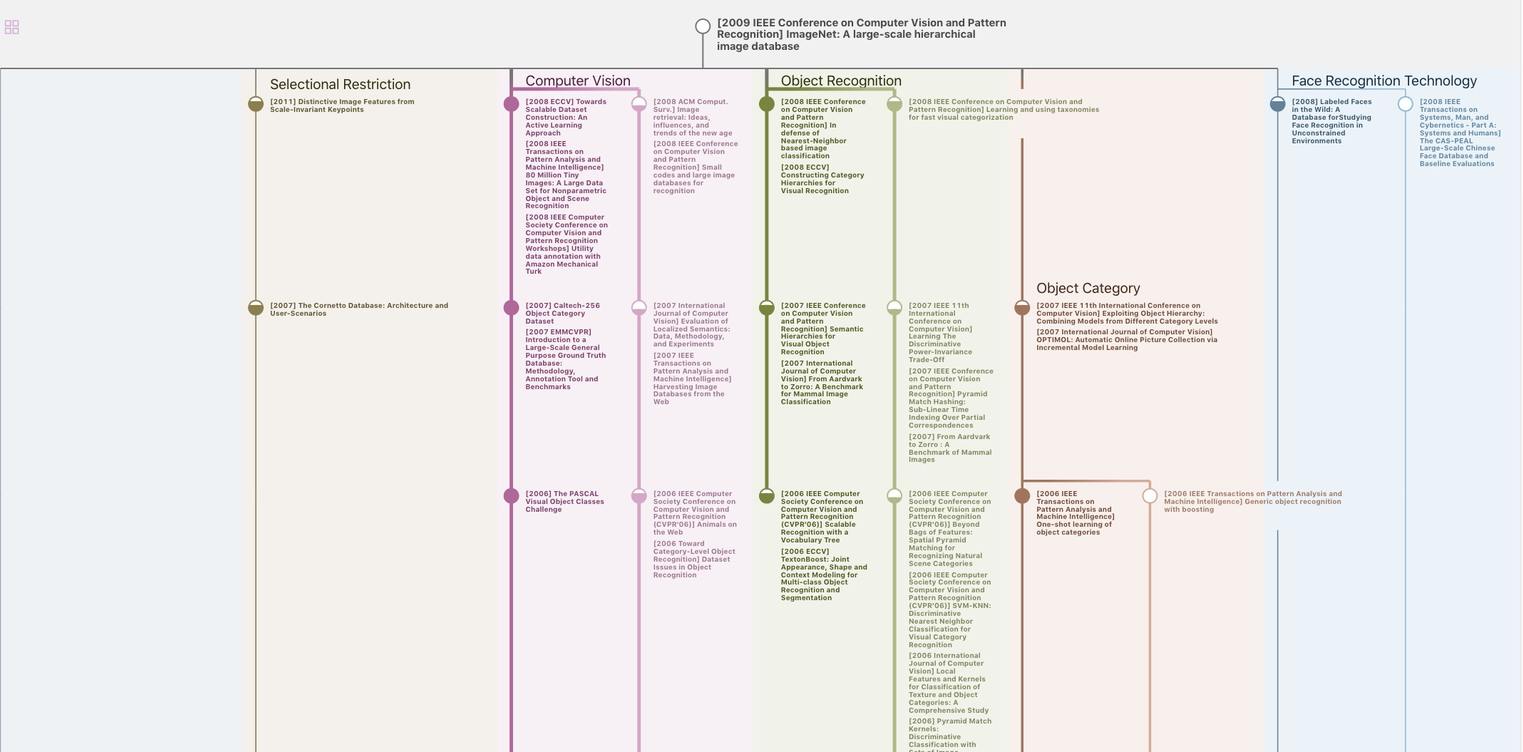
生成溯源树,研究论文发展脉络
Chat Paper
正在生成论文摘要