A coupled model to improve river water quality prediction towards addressing non-stationarity and data limitation
WATER RESEARCH(2024)
摘要
Accurate predictions of river water quality are vital for sustainable water management. However, even the powerful deep learning model, i.e., long short-term memory (LSTM), has difficulty in accurately predicting water quality dynamics owing to the high non-stationarity and data limitation in a changing environment. To wiggle out of quagmires, wavelet analysis (WA) and transfer learning (TL) techniques were introduced in this study to assist LSTM modeling, termed WA-LSTM-TL. Total phosphorus, total nitrogen, ammonia nitrogen, and permanganate index were predicted in a 4 h step within 49 water quality monitoring sites in a coastal province of China. We selected suitable source domains for each target domain using an innovatively proposed regionalization approach that included 20 attributes to improve the prediction efficiency of WA-LSTM-TL. The coupled WA-LSTM facilitated capturing non-stationary patterns of water quality dynamics and improved the performance by 53 % during testing phase compared to conventional LSTM. The WA-LSTM-TL, aided by the knowledge of source domain, obtained a 17 % higher performance compared to locally trained WA-LSTM, and such improvement was more impressive when local data was limited (+66 %). The benefit of TL-based modeling diminished as data quantity increased; however, it outperformed locally direct modeling regardless of whether target domain data was limited or sufficient. This study demonstrates the reasoning for coupling WA and TL techniques with LSTM models and provides a newly coupled modeling approach for improving short-term prediction of river water quality from the perspectives of non-stationarity and data limitation.
更多查看译文
关键词
River water quality,Deep learning,Wavelet analysis,Transfer learning,Non-stationarity,Data limitation
AI 理解论文
溯源树
样例
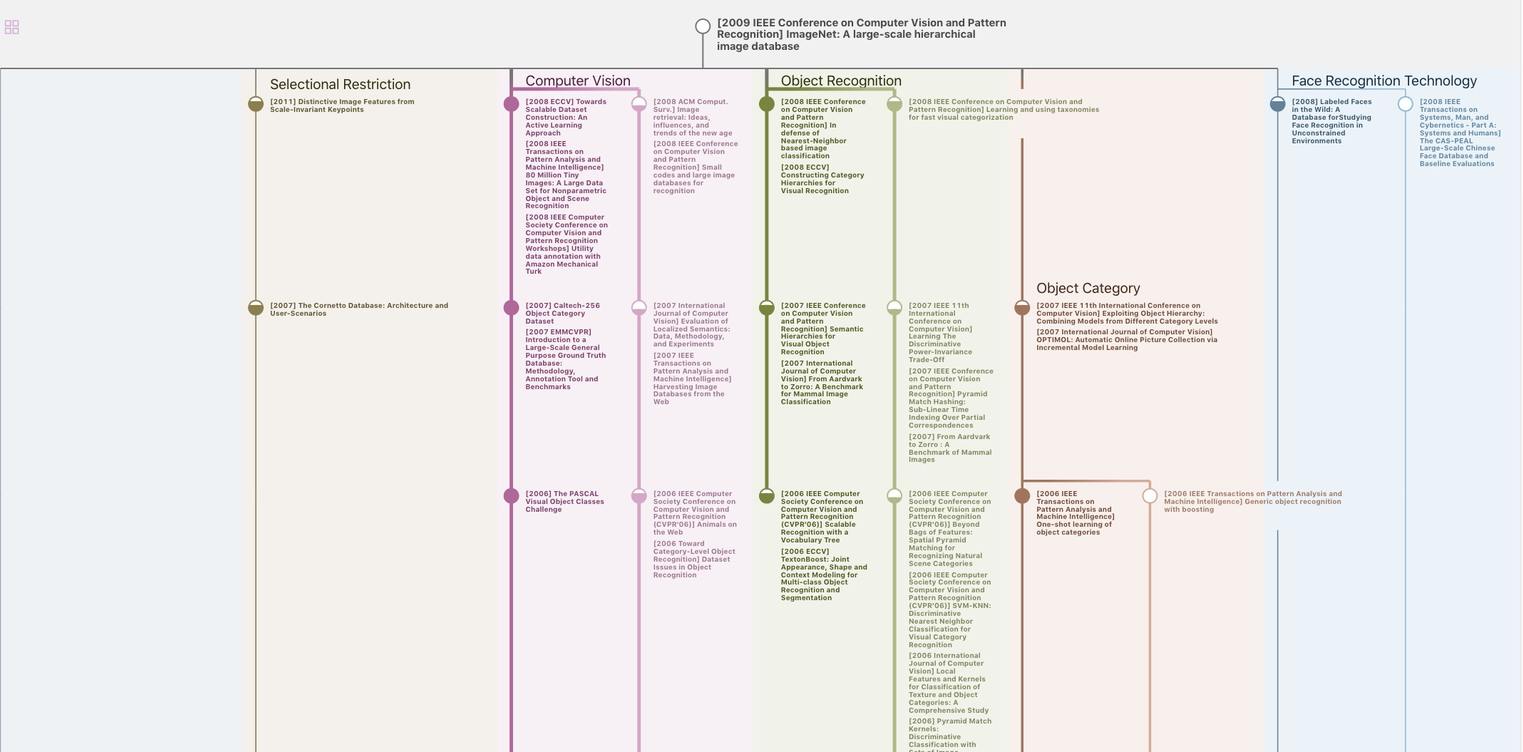
生成溯源树,研究论文发展脉络
Chat Paper
正在生成论文摘要