Maximum Fairness-Aware (k, r)-Core Identification in Large Graphs
DATABASES THEORY AND APPLICATIONS, ADC 2023(2024)
摘要
Cohesive subgraph mining is a fundamental problem in attributed graph analysis. The k-core model has been widely used in many studies to measure the cohesiveness of subgraphs. However, none of them considers the fairness of attributes in communities. To address this limitation, we propose a novel model, named fair (k, r)-core, to assess fairness equity within communities captured in attributed graphs. The model not only focuses on cohesive community structure but also eliminates vertices that contribute to unfairness. We prove that the problem of identifying the maximum fair (k, r)-core is NP-hard. To scale for large graphs, we introduce innovative pruning techniques and search methods. Comprehensive experiments are conducted on 6 real-world datasets to evaluate the efficiency and effectiveness of the proposed model and solutions.
更多查看译文
关键词
Community detection,k-core,fairness-aware
AI 理解论文
溯源树
样例
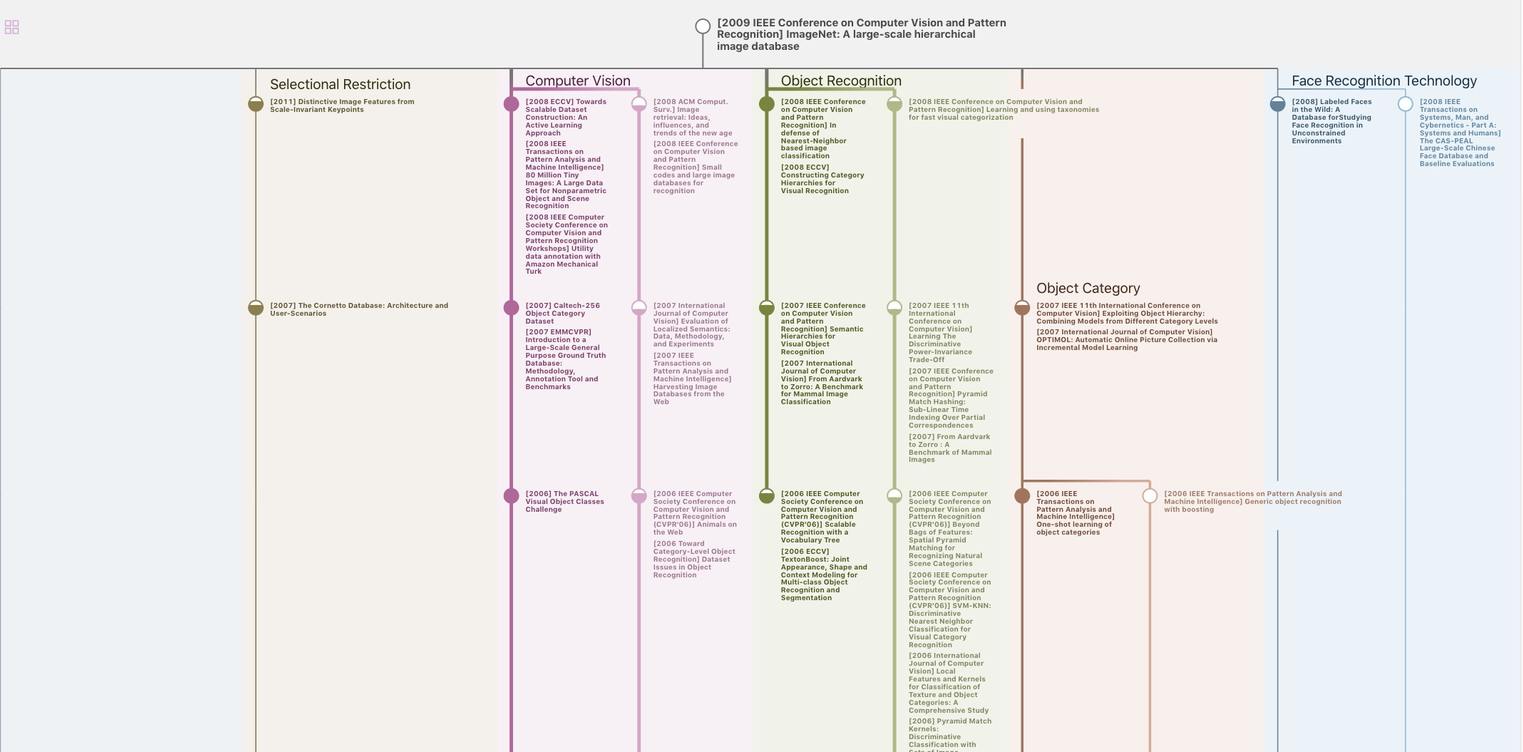
生成溯源树,研究论文发展脉络
Chat Paper
正在生成论文摘要