Exploiting Multi-modal Fusion for Robust Face Representation Learning with Missing Modality
ARTIFICIAL NEURAL NETWORKS AND MACHINE LEARNING, ICANN 2023, PT II(2023)
摘要
Current RGB-D-T face recognition methods are able to alleviate the sensitivity to facial variations, posture, occlusions, and illumination by incorporating complementary information, while they rely heavily on the availability of complete modalities. Given the likelihood of missing modalities in real-world scenarios and the fact that current multi-modal recognition models perform poorly when faced with incomplete data, robust multi-modal models for face recognition that can handle missing modalities are highly desirable. To this end, we propose a multi-modal fusion framework for robustly learning face representations in the presence of missing modalities, using a combination of RGB, depth, and thermal modalities. Our approach effectively blends these modalities together while also alleviating the semantic gap among them. Specifically, we put forward a novel modality-missing loss function to learn the modality-specific features that are robust to missing-modality data conditions. To project various modality features to the same semantic space, we exploit a joint modality-invariant representation with a central moment discrepancy (CMD) based distance constraint training strategy. We conduct extensive experiments on several benchmark datasets, such as VAP RGBD-T and Lock3DFace, and the results demonstrate the effectiveness and robustness of the proposed approach under uncertain missing-modality conditions compared with all baseline algorithms.
更多查看译文
关键词
multi-modal fusion,face representation learning,missing modality
AI 理解论文
溯源树
样例
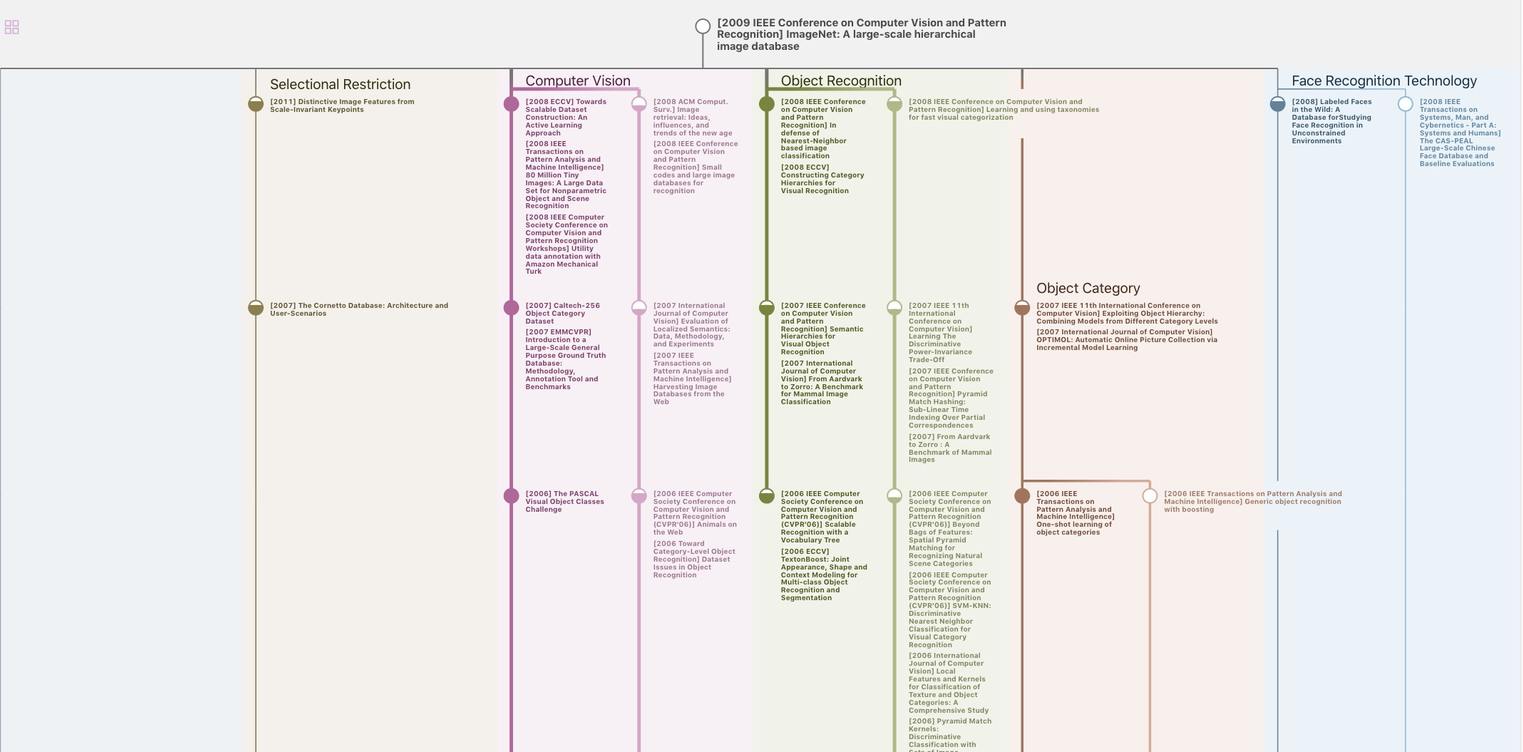
生成溯源树,研究论文发展脉络
Chat Paper
正在生成论文摘要