GII: A Unified Approach to Representation Learning in Open Set Recognition with Novel Category Discovery
ARTIFICIAL NEURAL NETWORKS AND MACHINE LEARNING, ICANN 2023, PT VIII(2023)
摘要
In this paper, we consider the problem of Novel Class Discovery (NCD) in Open Set Recognition (OSR). Given a labeled and an unlabeled set for training, NCD aims to discover the novel categories in the unlabeled set with prior knowledge learned from the labeled set. Existing approaches tackle the NCD problems under a close-set setting, where only the existing categories from the labeled set and the novel categories from the unlabeled set will occur during the inference. This paper considers a more realistic open-set scenario. In the open-set setting, in addition to the existing and novel categories, some unknown categories absent from the training could be present during inference. To address NCD in the open-set scenario, we propose the General Inter-Intra (GII) loss, a unified approach for learning representations from both labeled and unlabeled samples. The proposed approach discovers novel categories in the training set (NCD) meanwhile recognizes the unknown categories (OSR). We evaluate GII with image and graph datasets, and the results indicate that our proposed approach is more effective than other NCD and OSR approaches.
更多查看译文
关键词
Novel Category Discovery,Open Set Recognition,Representation Learning
AI 理解论文
溯源树
样例
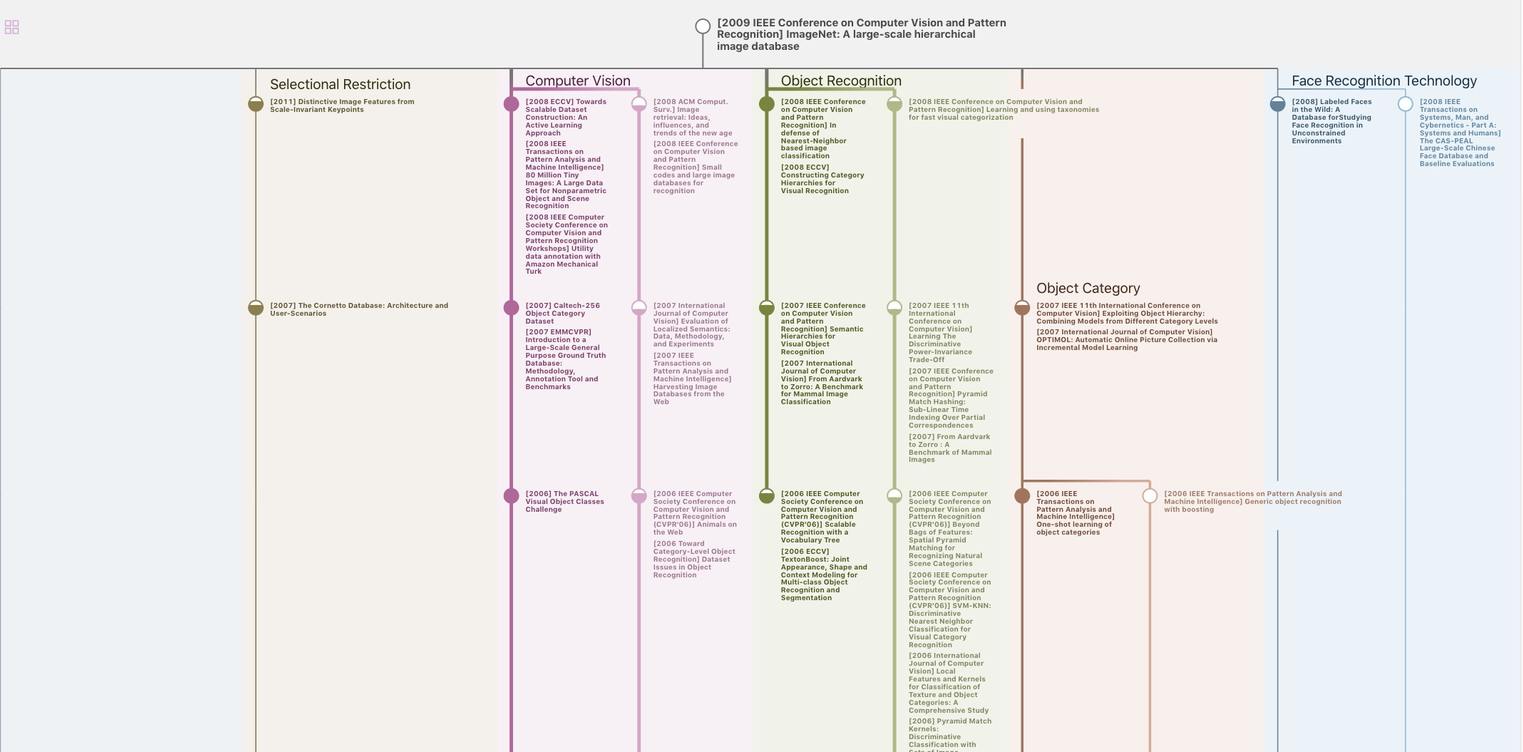
生成溯源树,研究论文发展脉络
Chat Paper
正在生成论文摘要