FaceReader: Unobtrusively Mining Vital Signs and Vital Sign Embedded Sensitive Info via AR/VR Motion Sensors.
CCS '23: Proceedings of the 2023 ACM SIGSAC Conference on Computer and Communications Security(2023)
摘要
The market size of augmented reality and virtual reality (AR/VR) has been expanding rapidly in recent years, with the use of face-mounted headsets extending beyond gaming to various application sectors, such as education, healthcare, and the military. Despite the rapid growth, the understanding of information leakage through sensor-rich headsets remains in its infancy. Some of the headset's built-in sensors do not require users' permission to access, and any apps and websites can acquire their readings. While theseunrestricted sensors are generally considered free of privacy risks, we find that an adversary could uncover private information by scrutinizing sensor readings, making existing AR/VR apps and websites potential eavesdroppers. In this work, we investigate a novel, unobtrusive privacy attack called FaceReader, which reconstructs high-quality vital sign signals (breathing and heartbeat patterns) based on unrestricted AR/VR motion sensors. FaceReader is built on the key insight that the headset is closely mounted on the user's face, allowing the motion sensors to detect subtle facial vibrations produced by users' breathing and heartbeats. Based on the reconstructed vital signs, we further investigate three more advanced attacks, including gender recognition, user re-identification, and body fat ratio estimation. Such attacks pose severe privacy concerns, as an adversary may obtain users' sensitive demographic/physiological traits and potentially uncover their real-world identities. Compared to prior privacy attacks relying on speeches and activities, FaceReader targets spontaneous breathing and heartbeat activities that are naturally produced by the human body and are unobtrusive to victims. In particular, we design an adaptive filter to dynamically mitigate the impacts of body motions. We further employ advanced deep-learning techniques to reconstruct vital sign signals, achieving signal qualities comparable to those of dedicated medical instruments, as well as deriving sensitive gender, identity, and body fat information. We conduct extensive experiments involving 35 users on three types of mainstream AR/VR headsets across 3 months. The results reveal that FaceReader can reconstruct vital signs with low mean errors and accurately detect gender (over 93.33%). The attack can also link/re-identify users across different apps, websites, and longitudinal sessions with over 97.83% accuracy. Furthermore, we present the first successful attempt at revealing body fat information from motion sensor data, achieving a remarkably low estimation error of 4.43%.
更多查看译文
AI 理解论文
溯源树
样例
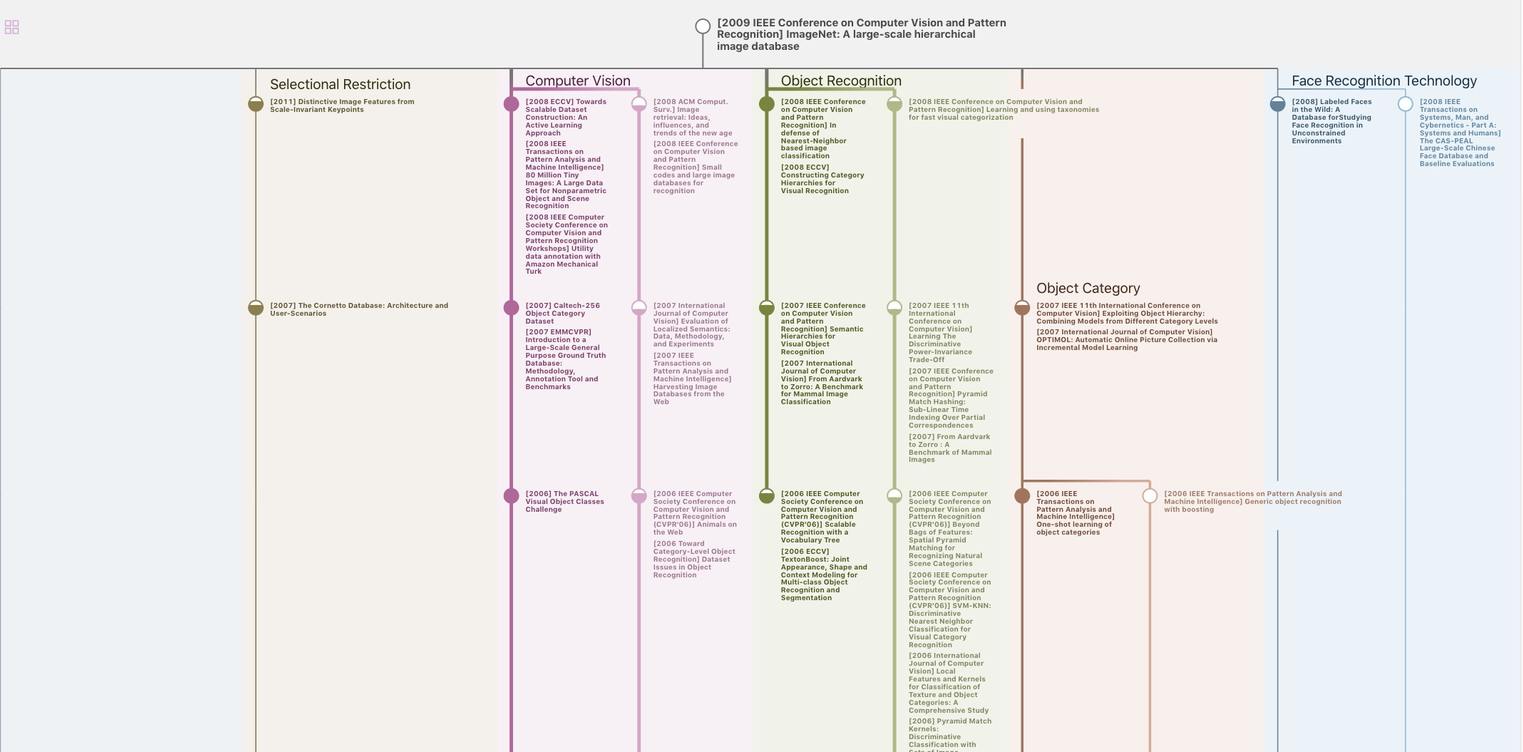
生成溯源树,研究论文发展脉络
Chat Paper
正在生成论文摘要