Vulnerability Intelligence Alignment via Masked Graph Attention Networks
PROCEEDINGS OF THE 2023 ACM SIGSAC CONFERENCE ON COMPUTER AND COMMUNICATIONS SECURITY, CCS 2023(2023)
摘要
Cybersecurity vulnerability information is often sourced from multiple channels, such as government vulnerability repositories, individually maintained vulnerability-gathering platforms, or vulnerability-disclosure email lists and forums. Integrating vulnerability information from different channels enables comprehensive threat assessment and quick deployment to various security mechanisms. However, automatic integration of vulnerability information, especially those lacking decisive information (e.g., CVE-ID), is hindered by the limitations of today's entity alignment techniques. In our study, we annotate and release the first cybersecurity-domain vulnerability alignment dataset, and highlight the unique characteristics of security entities, including the inconsistent vulnerability artifacts of identical vulnerability (e.g., impact and affected version) in different vulnerability repositories. Based on these characteristics, we propose an entity alignment model, CEAM, for integrating vulnerability information from multiple sources. CEAM equips graph neural network-based entity alignment techniques with two application-driven mechanisms: asymmetric masked aggregation and partitioned attention. These techniques selectively aggregate vulnerability artifacts to learn the semantic embeddings for vulnerabilities by an asymmetric mask, while ensuring that the artifacts critical to the vulnerability identification are always taken more consideration. Experimental results on vulnerability alignment datasets demonstrate that CEAM significantly outperforms state-of-the-art entity alignment methods.
更多查看译文
关键词
Entity Alignment,Vulnerability Intelligence,Knowledge Graph Alignment,Graph Attention Networks,Vulnerability Repository Inconsistency
AI 理解论文
溯源树
样例
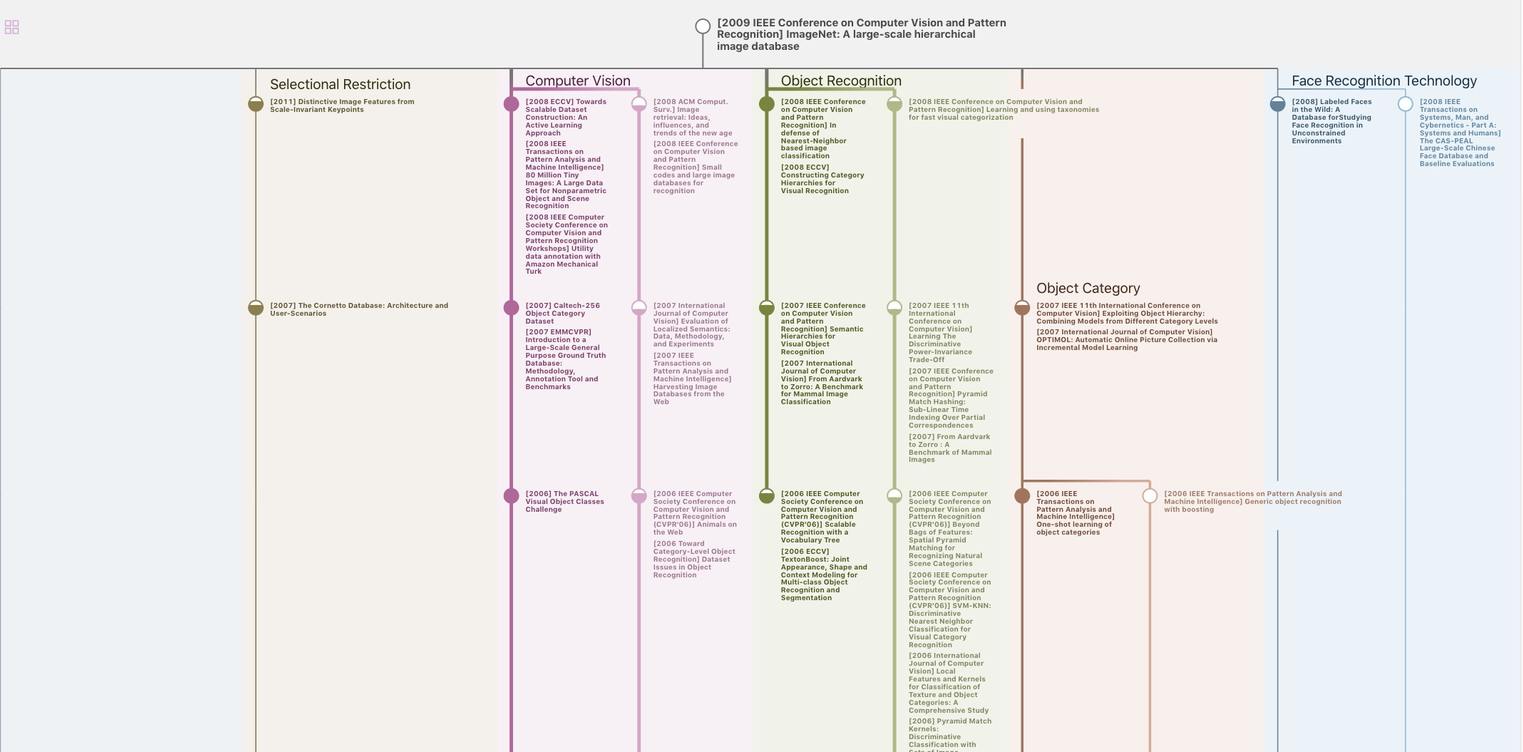
生成溯源树,研究论文发展脉络
Chat Paper
正在生成论文摘要