Comprehension from Chaos: Towards Informed Consent for Private Computation
PROCEEDINGS OF THE 2023 ACM SIGSAC CONFERENCE ON COMPUTER AND COMMUNICATIONS SECURITY, CCS 2023(2023)
摘要
Private computation, which includes techniques like multi-party computation and private query execution, holds great promise for enabling organizations to analyze data they and their partners hold while maintaining data subjects' privacy. Despite recent interest in communicating about differential privacy, end users' perspectives on private computation have not previously been studied. To fill this gap, we conducted 22 semi-structured interviews investigating users' understanding of, and expectations for, private computation over data about them. Interviews centered on four concrete data-analysis scenarios (e.g., ad conversion analysis), each with a variant that did not use private computation and another that did. While participants struggled with abstract definitions of private computation, they found the concrete scenarios enlightening and plausible even though we did not explain the complex cryptographic underpinnings. Private computation increased participants' acceptance of data sharing, but not unconditionally; the purpose of data sharing and analysis was the primary driver of their attitudes. Through collective activities, participants emphasized the importance of detailing the purpose of a computation and clarifying that inputs to private computation are not shared across organizations when describing private computation to end users.
更多查看译文
关键词
Private Computation,Secure Multi-Party Computation,User Study
AI 理解论文
溯源树
样例
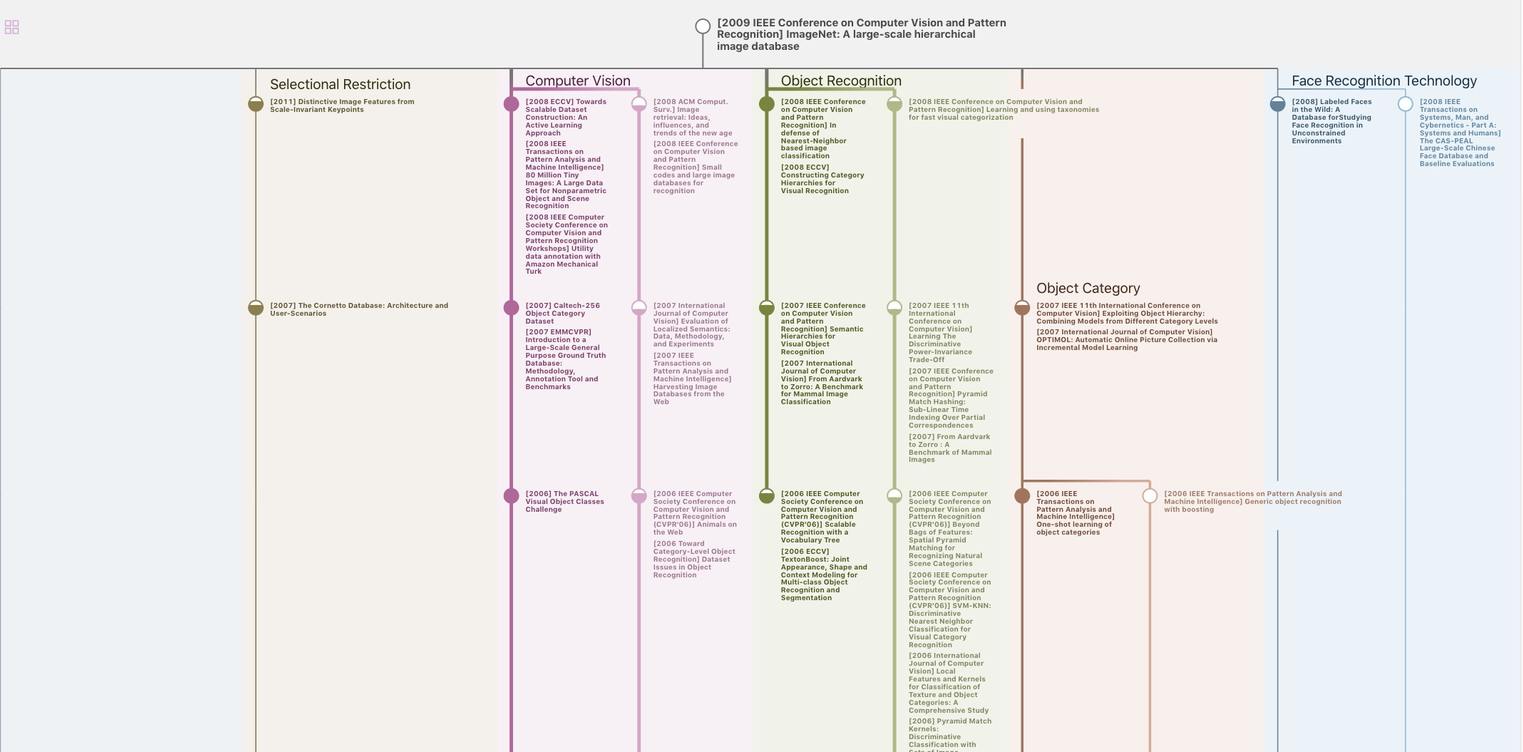
生成溯源树,研究论文发展脉络
Chat Paper
正在生成论文摘要