Medical Image Segmentation Approach via Transformer Knowledge Distillation
CVIPPR '23: Proceedings of the 2023 Asia Conference on Computer Vision, Image Processing and Pattern Recognition(2023)
摘要
Numerous transformer-based medical image segmentation methods have been proposed and achieved good segmentation results. However, it is still a challenge to train and deploy transformer networks to mobile medical devices due to a large number of model parameters. To resolve the training and model parameter problems, in this paper, we propose a Transformer-based network for Medical Image Segmentation using Knowledge Distillation named MISTKD. The MISTKD consists of a teacher network and a student network. It achieves comparable performance to state-of-the-art transformer works using fewer parameters by employing the teacher network to train the student network. The training can be implemented by extracting the sequence in the teacher and student encoder networks during the training procedure. The losses between sequences are further calculated, thus the student network can learn from the teacher network. The experimental results on Synapse show that the proposed work achieves competitive performance using only one-eighth parameters.
更多查看译文
AI 理解论文
溯源树
样例
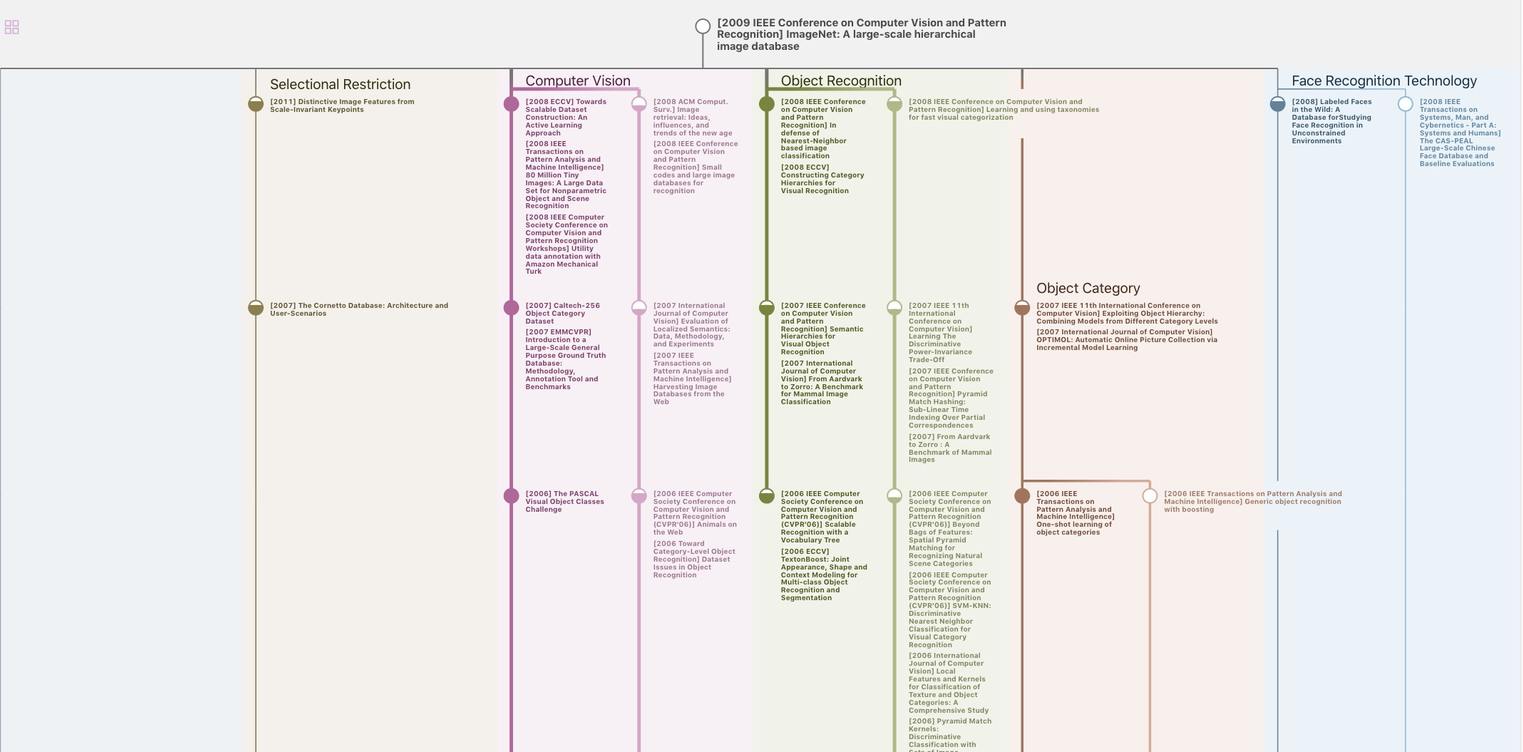
生成溯源树,研究论文发展脉络
Chat Paper
正在生成论文摘要