TREAD-M3D: Temperature-Aware DNN Accelerators for Monolithic 3-D Mobile Systems
IEEE TRANSACTIONS ON COMPUTER-AIDED DESIGN OF INTEGRATED CIRCUITS AND SYSTEMS(2023)
摘要
Monolithic 3-D (MONO3 D) integration provides performance and power efficiency benefits over 2-D circuits and, thus, is a potent technology for the design of deep neural network (DNN) accelerators with enhanced energy efficiency. However, high IC temperatures are major challenges for the design of MONO3 D systems. To this end, this article focuses on designing temperature-aware MONO3 D DNN accelerators. We propose a new automated method, called TREAD- M3 D, that provides a near-optimal MONO3 D DNN accelerator architecture in terms of systolic array size, SRAM organization, partition across 3-D layers, and operating frequency, for a given DNN, optimization goal, and temperature constraint. TREAD- M3 D incorporates circuit- and architecture-level models to evaluate the power and performance characteristics of different partitions. Our method reveals valuable insights and enables tradeoff analysis for achieving high energy efficiency in MONO3 D systolic arrays. In comparison to recent works that adopt a fixed partition choice to design MONO3 D DNN systems, TREAD- M3 D yields up to 22 % higher energy efficiency. Using TREAD- M3 D, we further demonstrate that temperature unawareness not only leads to infeasible configurations due to temperature violations but also over-estimates energy-delay-product benefits by up to 24 %.
更多查看译文
关键词
Systolic arrays,Energy efficiency,Three-dimensional displays,Optimization,Integrated circuits,Resistive RAM,Organizations,Deep neural networks (DNNs),energy efficiency,monolithic 3-D (Mono3D),systolic arrays,temperature optimization
AI 理解论文
溯源树
样例
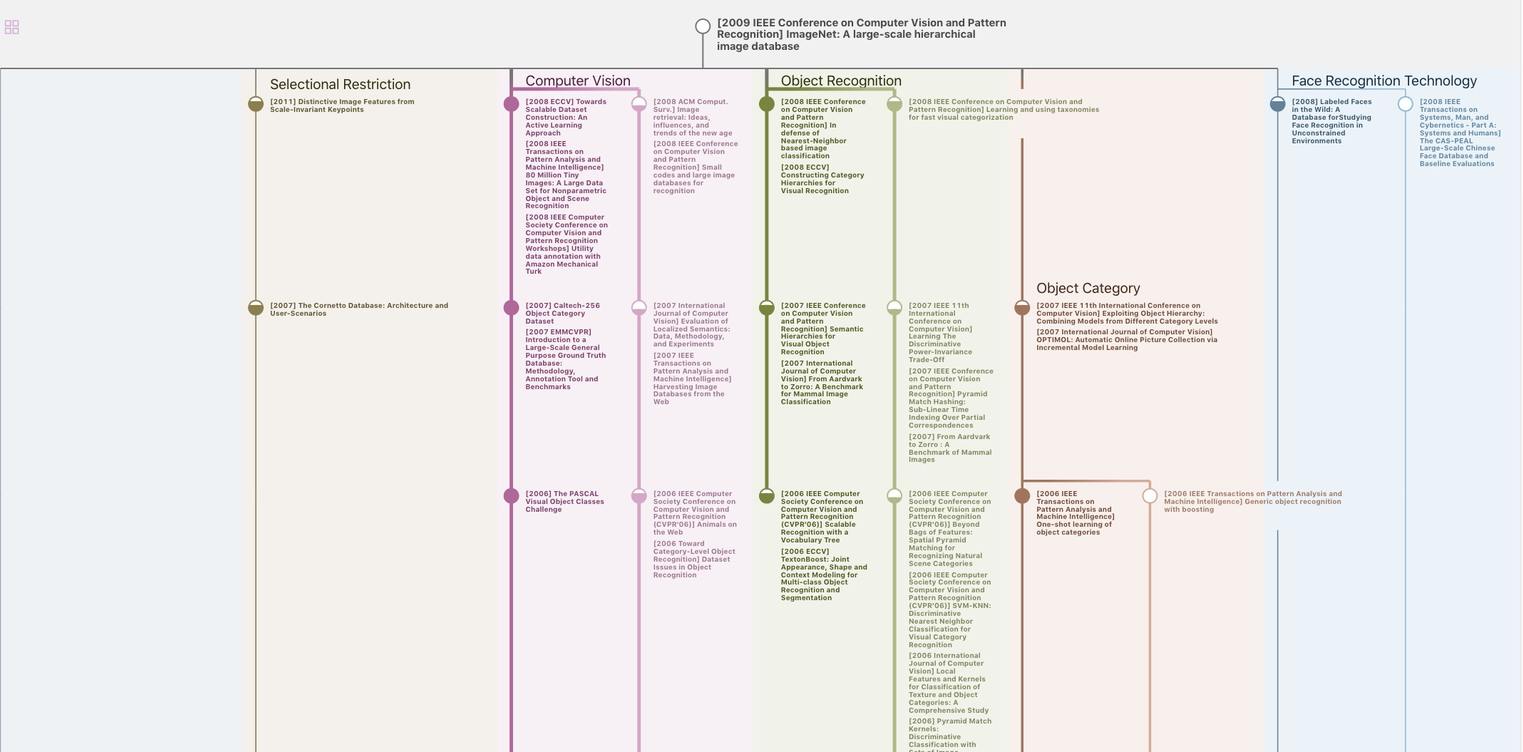
生成溯源树,研究论文发展脉络
Chat Paper
正在生成论文摘要