Direct Amortized Likelihood Ratio Estimation
AAAI 2024(2024)
摘要
We introduce a new amortized likelihood ratio estimator for likelihood-free simulation-based inference (SBI). Our estimator is simple to train and estimates the likelihood ratio using a single forward pass of the neural estimator. Our approach directly computes the likelihood ratio between two competing parameter sets which is different from the previous approach of comparing two neural network output values. We refer to our model as the direct neural ratio estimator (DNRE). As part of introducing the DNRE, we derive a corresponding Monte Carlo estimate of the posterior. We benchmark our new ratio estimator and compare to previous ratio estimators in the literature. We show that our new ratio estimator often outperforms these previous approaches. As a further contribution, we introduce a new derivative estimator for likelihood ratio estimators that enables us to compare likelihood-free Hamiltonian Monte Carlo (HMC) with random-walk Metropolis-Hastings (MH). We show that HMC is equally competitive, which has not been previously shown. Finally, we include a novel real-world application of SBI by using our neural ratio estimator to design a quadcopter. Code is available at https://github.com/SRI-CSL/dnre.
更多查看译文
关键词
RU: Probabilistic Inference,ML: Bayesian Learning
AI 理解论文
溯源树
样例
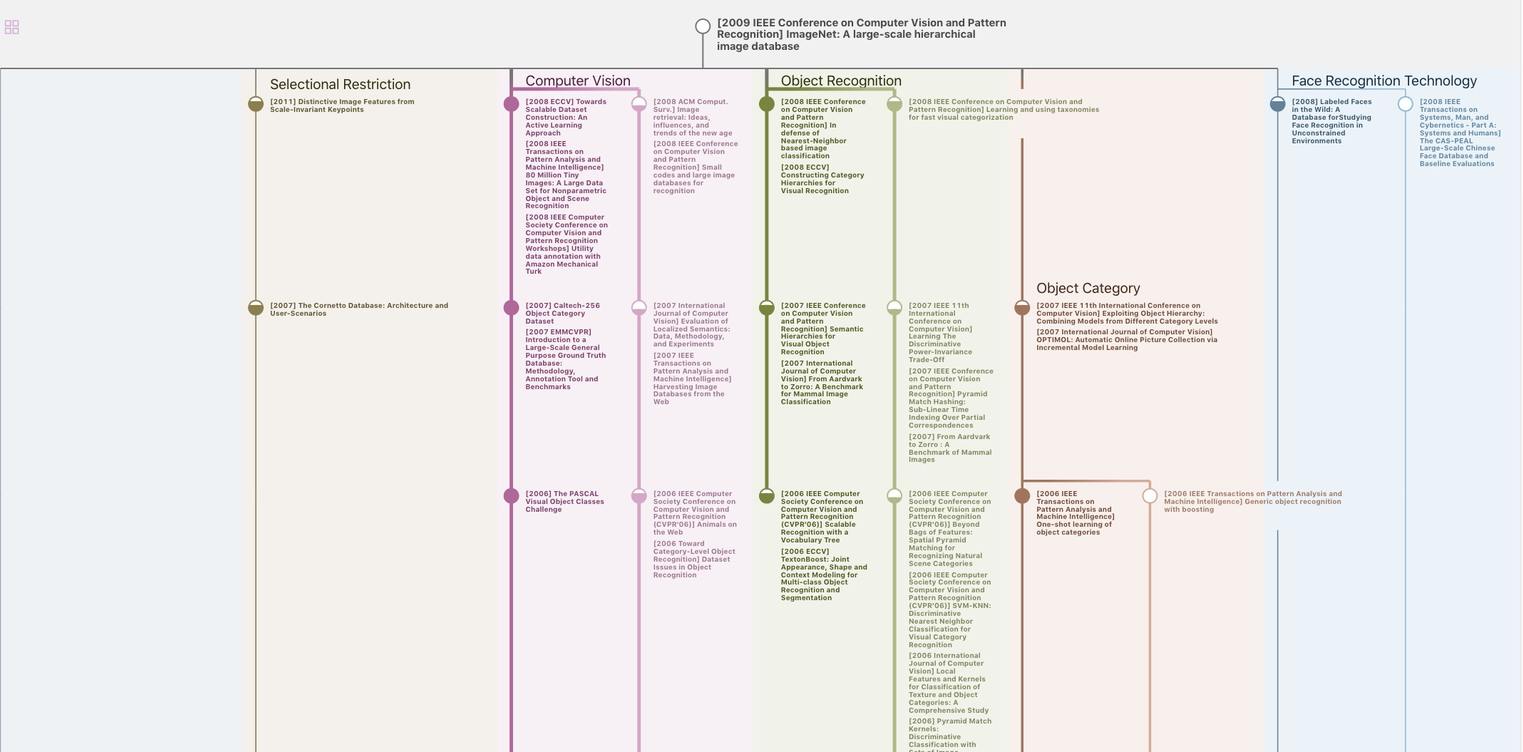
生成溯源树,研究论文发展脉络
Chat Paper
正在生成论文摘要