On the adversarial robustness of full integer quantized TinyML models at the edge.
MiddleWEdge '23: Proceedings of the 2nd International Workshop on Middleware for the Edge(2023)
摘要
The recent surge in deploying machine learning (ML) models at the edge has revolutionized various industries by enabling real-time decision-making on resource-constrained devices, such as TinyML models on microcontrollers. However, this trend brings forth a critical concern - the vulnerability of these models to adversarial examples. ML at the edge offers tremendous potential but demands heightened vigilance in the realm of cybersecurity. Our research has shown that any adversarial robustness attained in standard TensorFlow models through adversarial training can be completely nullified during post-training full integer quantization to address resource constraints of edge devices. This finding raises crucial questions about the adversarial robustness of TinyML models on microcontrollers limited to integer-only operations. As edge computing continues to proliferate, addressing these vulnerabilities and developing lightweight defenses tailored to resource-constrained environments becomes imperative for ensuring the security and trustworthiness of edge-deployed ML models.
更多查看译文
AI 理解论文
溯源树
样例
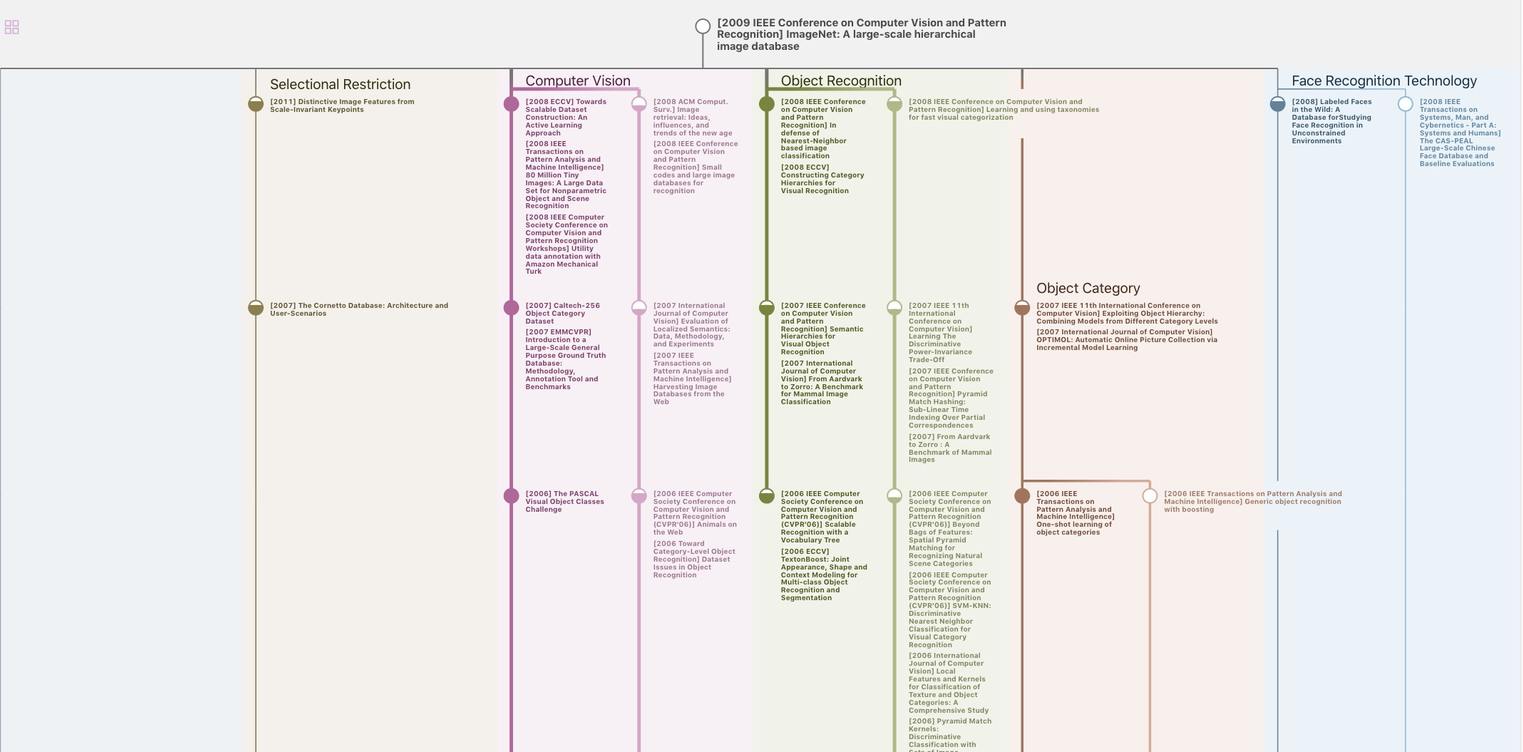
生成溯源树,研究论文发展脉络
Chat Paper
正在生成论文摘要