Understanding Any Time Series Classifier with a Subsequence-based Explainer
ACM TRANSACTIONS ON KNOWLEDGE DISCOVERY FROM DATA(2024)
摘要
The growing availability of time series data has increased the usage of classifiers for this data type. Unfortunately, state-of-the-art time series classifiers are black-box models and, therefore, not usable in critical domains such as healthcare or finance, where explainability can be a crucial requirement. This paper presents a framework to explain the predictions of any black-box classifier for univariate and multivariate time series. The provided explanation is composed of three parts. First, a saliency map highlighting the most important parts of the time series for the classification. Second, an instance-based explanation exemplifies the black-box's decision by providing a set of prototypical and counterfactual time series. Third, a factual and counterfactual rule-based explanation, revealing the reasons for the classification through logical conditions based on subsequences that must, or must not, be contained in the time series. Experiments and benchmarks show that the proposed method provides faithful, meaningful, stable, and interpretable explanations.
更多查看译文
关键词
Explainable AI,time series classification,subsequence-based rules,prototypes and counterfactuals
AI 理解论文
溯源树
样例
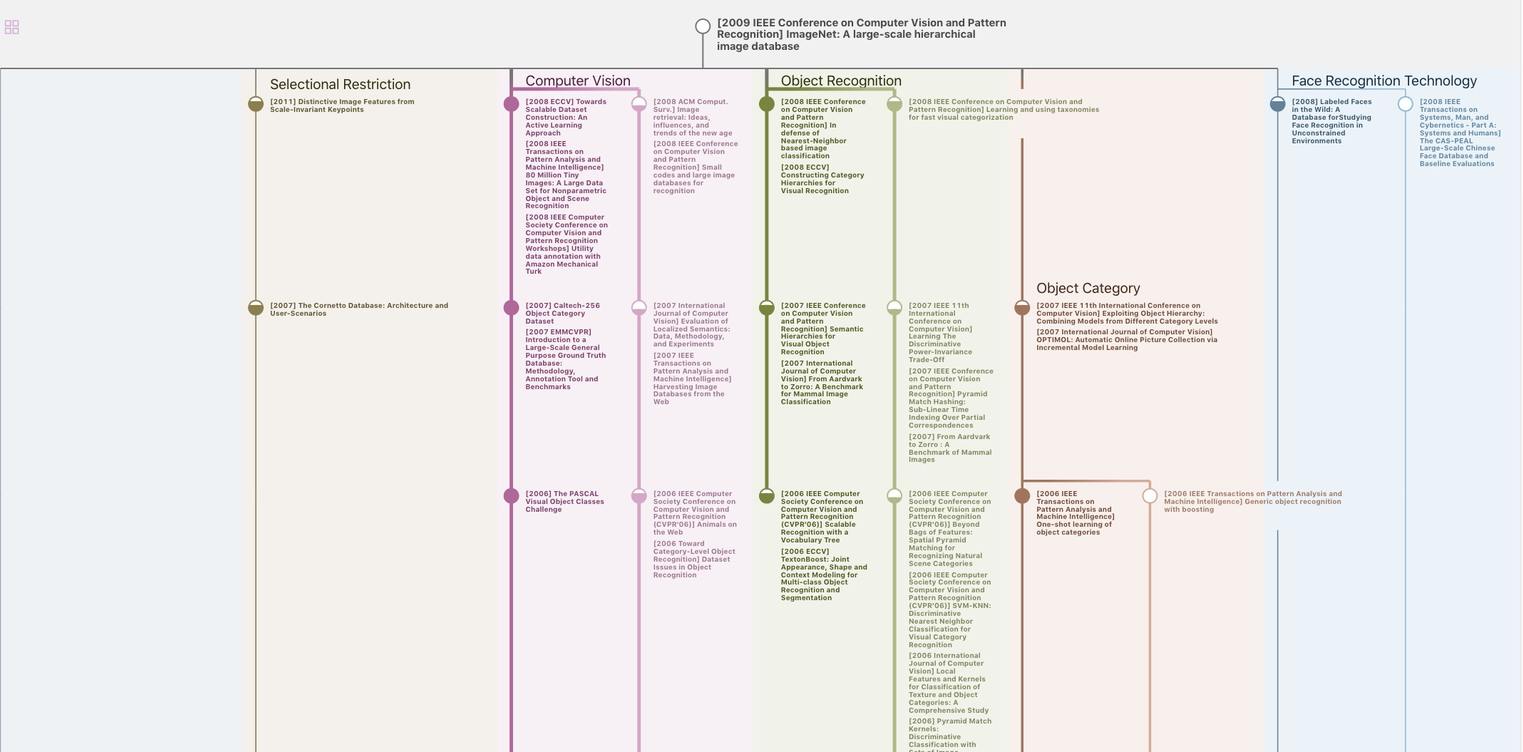
生成溯源树,研究论文发展脉络
Chat Paper
正在生成论文摘要