MetaMix: Meta-State Precision Searcher for Mixed-Precision Activation Quantization
AAAI 2024(2024)
摘要
Mixed-precision quantization of efficient networks often suffer from activation instability encountered in the exploration of bit selections. To address this problem, we propose a novel method called MetaMix which consists of bit selection and weight training phases. The bit selection phase iterates two steps, (1) the mixed-precision-aware weight update, and (2) the bit-search training with the fixed mixed-precision-aware weights, both of which combined reduce activation instability in mixed-precision quantization and contribute to fast and high-quality bit selection. The weight training phase exploits the weights and step sizes trained in the bit selection phase and fine-tunes them thereby offering fast training. Our experiments with efficient and hard-to-quantize networks, i.e., MobileNet v2 and v3, and ResNet-18 on ImageNet show that our proposed method pushes the boundary of mixed-precision quantization, in terms of accuracy vs. operations, by outperforming both mixed- and single-precision SOTA methods.
更多查看译文
关键词
ML: Optimization
AI 理解论文
溯源树
样例
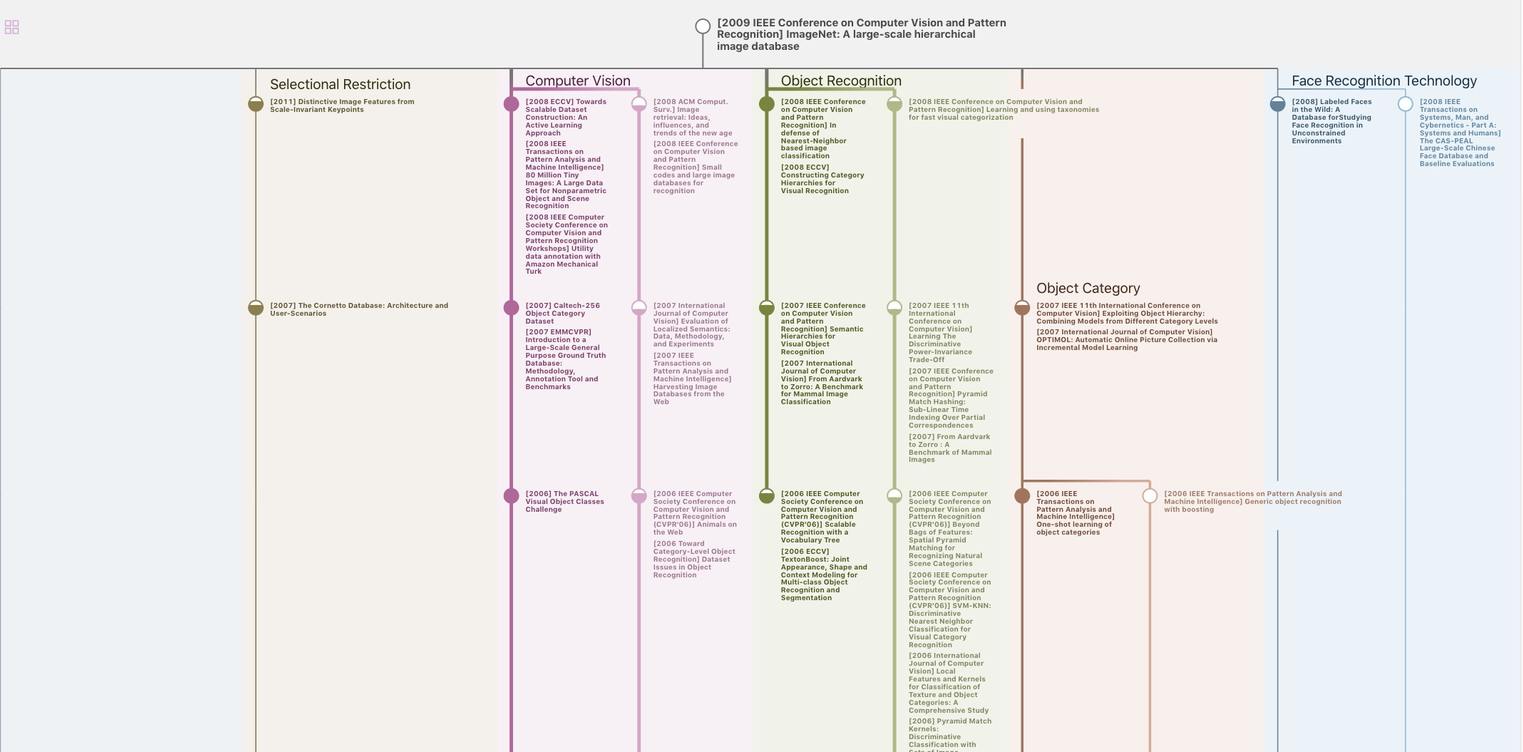
生成溯源树,研究论文发展脉络
Chat Paper
正在生成论文摘要