A Deep Learning-Based Novel Class Discovery Approach for Automatic Modulation Classification
IEEE COMMUNICATIONS LETTERS(2023)
摘要
The existing Automatic Modulation Classification (AMC) methods require the training and testing datasets share a common set of modulation categories. However, the AMC model may encounter the need to discriminate novel classes in non-cooperative environments. To the best of our knowledge, the research reporting AMC in the class-disjoint environment has not been addressed yet. In this letter, a novel class discovery method is proposed for AMC leveraging the information contained in the labeled training dataset. Specifically, a 3-stage deep learning method is introduced to recognize samples of the known classes and cluster samples of novel classes. The extracted features and the pairwise similarity relationship are considered as the common knowledge between the two class-disjoint datasets and are utilized to help the construction and training of the classifier for novel classes. The simulation results validate the effectiveness and performance of the proposed method.
更多查看译文
关键词
Automatic modulation classification,novel class discovery,deep learning
AI 理解论文
溯源树
样例
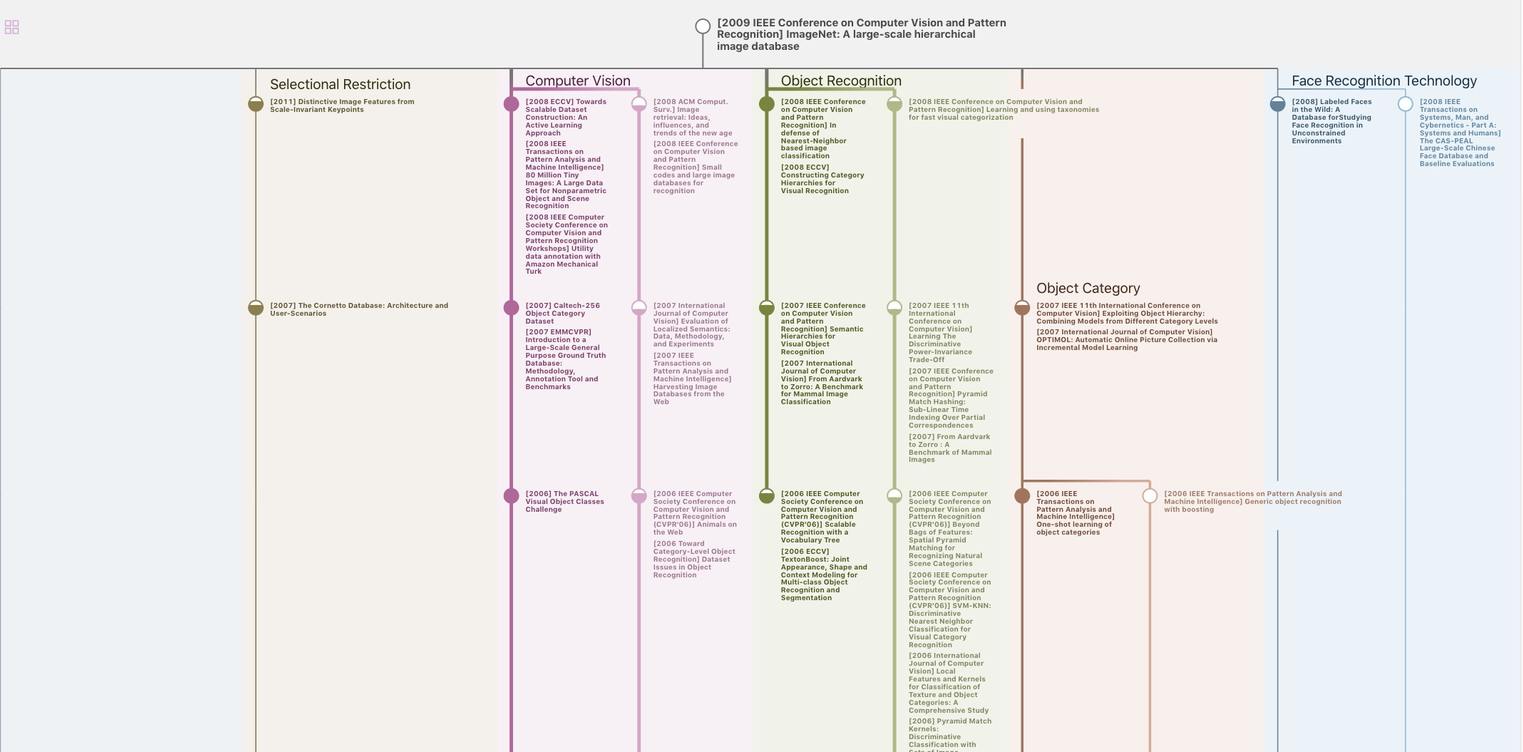
生成溯源树,研究论文发展脉络
Chat Paper
正在生成论文摘要