Multiagent Deep Reinforcement Learning for Automated Truck Platooning Control
IEEE INTELLIGENT TRANSPORTATION SYSTEMS MAGAZINE(2024)
摘要
Human-leading automated truck platooning has been an effective technique to improve traffic capacity and fuel economy and eliminate uncertainties of the traffic environment. Aiming for a tradeoff between the dynamic response of car following and energy-efficient platooning control, a predictive information multiagent soft actor-critic (PI-MASAC) control framework is proposed for a human-leading automated heavy-duty-truck platoon. In this framework, predictive information of environmental dynamics is modeled as the state representation of a deep reinforcement learning algorithm to address the uncertainties of a partially observable environment. In the truck model, the impact of intraplatoon aerodynamic interactions is modeled, which is used to design a constant spacing policy for platooning control. We demonstrate the effectiveness of our approach by testing the human-leading truck platoon under multiple scenarios compared to proximal policy optimization, an intelligent driver model, and linear-based cooperative adaptive cruise control. Our results show that the PI-MASAC learns a novel car-following strategy of peak shaving and valley filling and therefore significantly enhances energy savings by reducing high-intensity accelerations and decelerations. In addition, the PI-MASAC demonstrates its adaptability to various initial scenarios and exhibits good generalization to a larger platoon size.
更多查看译文
关键词
Aerodynamics,Atmospheric modeling,Vehicle dynamics,Drag,Adaptation models,Uncertainty,Stability analysis
AI 理解论文
溯源树
样例
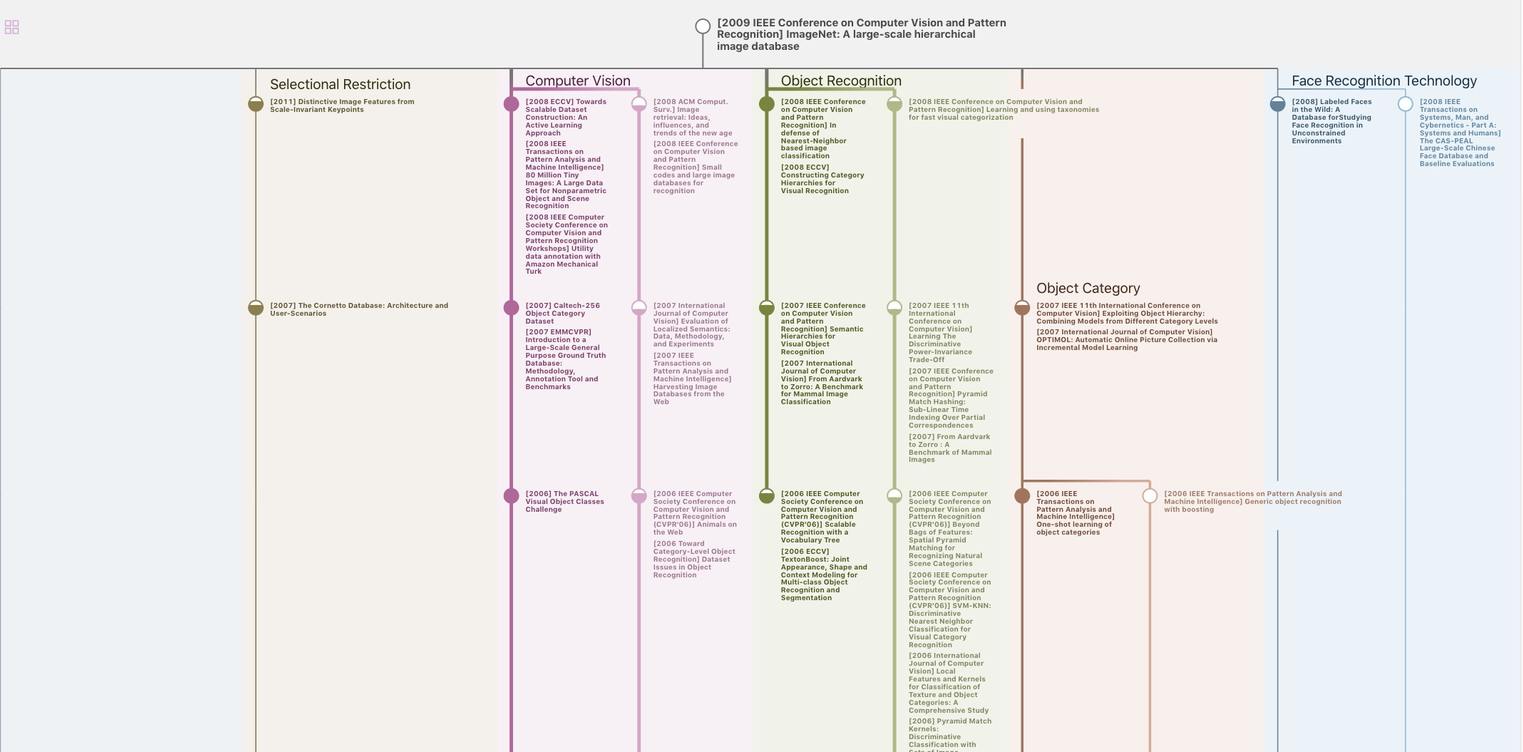
生成溯源树,研究论文发展脉络
Chat Paper
正在生成论文摘要