A Self-Adaptive Robustness Optimization Method With Evolutionary Multi-Agent for IoT Topology
IEEE-ACM TRANSACTIONS ON NETWORKING(2024)
摘要
Topology robustness is critical to the connectivity and lifetime of large-scale Internet-of-Things (IoT) applications. To improve robustness while reducing the execution cost, the existing robustness optimization methods utilize neural learning schemes, including neural networks, deep learning, and reinforcement learning. However, insufficient exploration of reinforcement learning agents for topological environments is likely to yield local optima. Moreover, convergence speed is influenced by the sparse reward problem generated while exploring topological environments. To address these problems, this study proposes a self-adaptive robustness optimization method with an evolutionary multi-agent for IoT topology (ROMEM). ROMEM introduces a new multi-agent co-evolution scheme that leverages a non-deterministic strategy to extend the exploration in multi-directions, enabling the reinforcement learning agent to transcend local optima. Furthermore, ROMEM presents a novel distributed training mechanism for multiple agents to accelerate convergence. Experimental results demonstrate that ROMEM can achieve multi-directional collaborative training and outperform other state-of-the-art learning-based robustness optimization methods in terms of convergence efficiency and robustness.
更多查看译文
关键词
Internet of Things,network topology,robustness optimization,soft actor-critic,evolutionary reinforcement learning
AI 理解论文
溯源树
样例
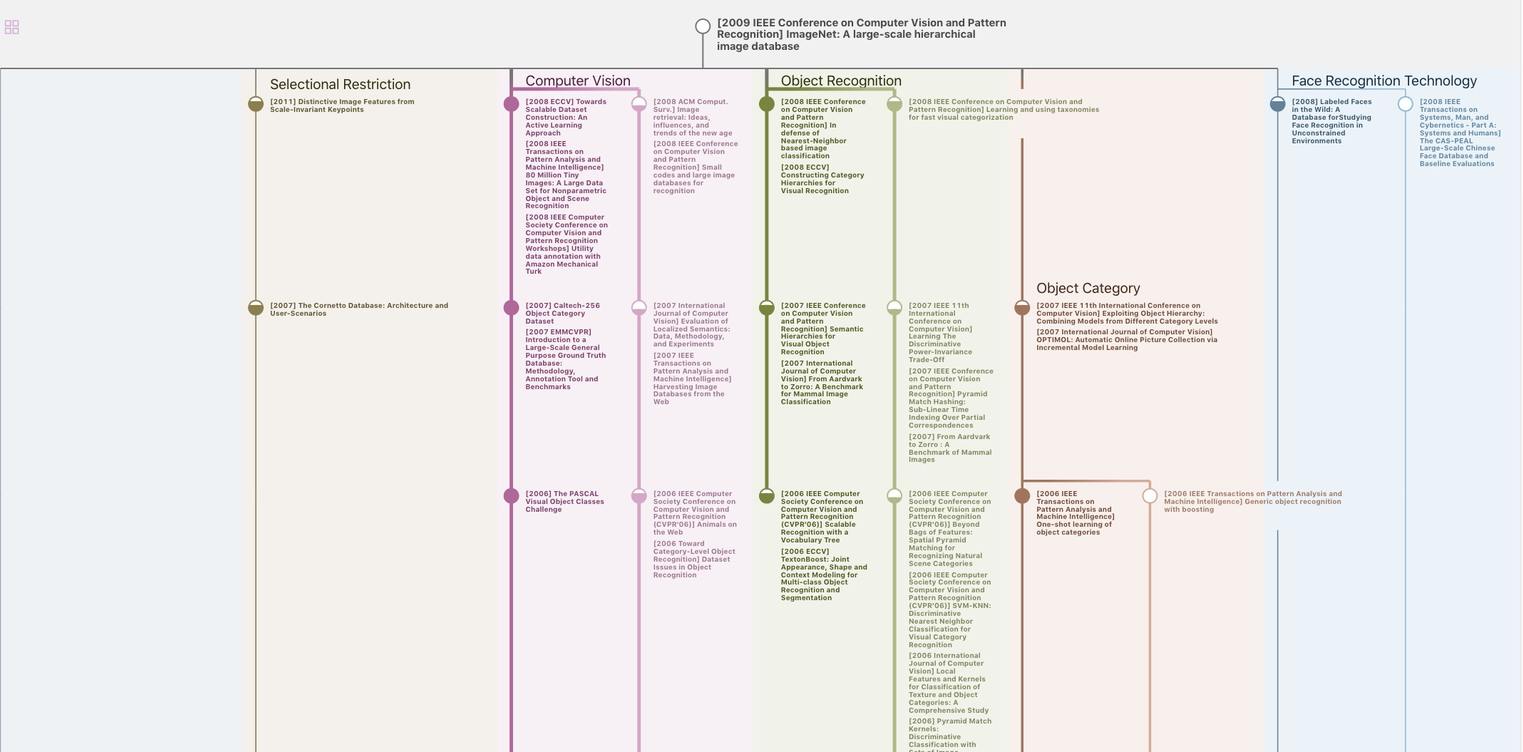
生成溯源树,研究论文发展脉络
Chat Paper
正在生成论文摘要