SQ Lower Bounds for Non-Gaussian Component Analysis with Weaker Assumptions.
NeurIPS(2023)
摘要
We study the complexity of Non-Gaussian Component Analysis (NGCA) in the Statistical Query (SQ) model.
Prior work developed a methodology to prove SQ lower bounds for NGCA that have been applicable to a wide range of contexts.
In particular, it was known that for any univariate distribution $A$ satisfying certain conditions,
distinguishing between a standard multivariate Gaussian and a distribution that behaves like $A$ in a random hidden direction and like a standard Gaussian in the orthogonal complement, is SQ-hard.
The required conditions were that (1) $A$ matches many low-order moments with a standard Gaussian,
and (2) the chi-squared norm of $A$ with respect to the standard Gaussian is finite.
While the moment-matching condition is clearly necessary for hardness, the chi-squared condition was only required for technical reasons.
In this work, we establish that the latter condition is indeed not necessary.
In particular, we prove near-optimal SQ lower bounds for NGCA under the moment-matching condition only.
更多查看译文
AI 理解论文
溯源树
样例
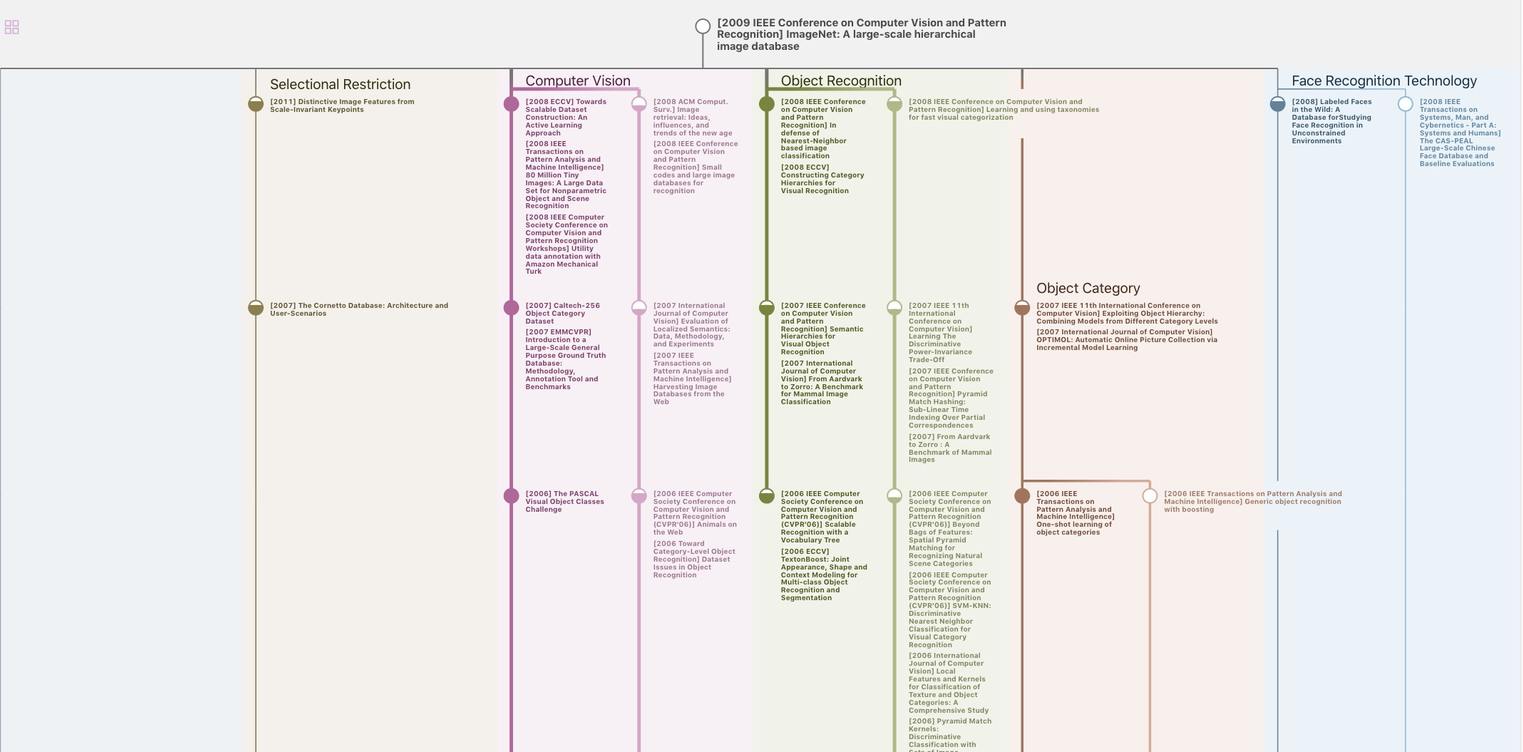
生成溯源树,研究论文发展脉络
Chat Paper
正在生成论文摘要