A Hierarchical Spatial Transformer for Massive Point Samples in\n Continuous Space
NeurIPS(2023)
摘要
Transformers are widely used deep learning architectures. Existing\ntransformers are mostly designed for sequences (texts or time series), images\nor videos, and graphs. This paper proposes a novel transformer model for\nmassive (up to a million) point samples in continuous space. Such data are\nubiquitous in environment sciences (e.g., sensor observations), numerical\nsimulations (e.g., particle-laden flow, astrophysics), and location-based\nservices (e.g., POIs and trajectories). However, designing a transformer for\nmassive spatial points is non-trivial due to several challenges, including\nimplicit long-range and multi-scale dependency on irregular points in\ncontinuous space, a non-uniform point distribution, the potential high\ncomputational costs of calculating all-pair attention across massive points,\nand the risks of over-confident predictions due to varying point density. To\naddress these challenges, we propose a new hierarchical spatial transformer\nmodel, which includes multi-resolution representation learning within a\nquad-tree hierarchy and efficient spatial attention via coarse approximation.\nWe also design an uncertainty quantification branch to estimate prediction\nconfidence related to input feature noise and point sparsity. We provide a\ntheoretical analysis of computational time complexity and memory costs.\nExtensive experiments on both real-world and synthetic datasets show that our\nmethod outperforms multiple baselines in prediction accuracy and our model can\nscale up to one million points on one NVIDIA A100 GPU. The code is available at\n\\url{https://github.com/spatialdatasciencegroup/HST}.
更多查看译文
AI 理解论文
溯源树
样例
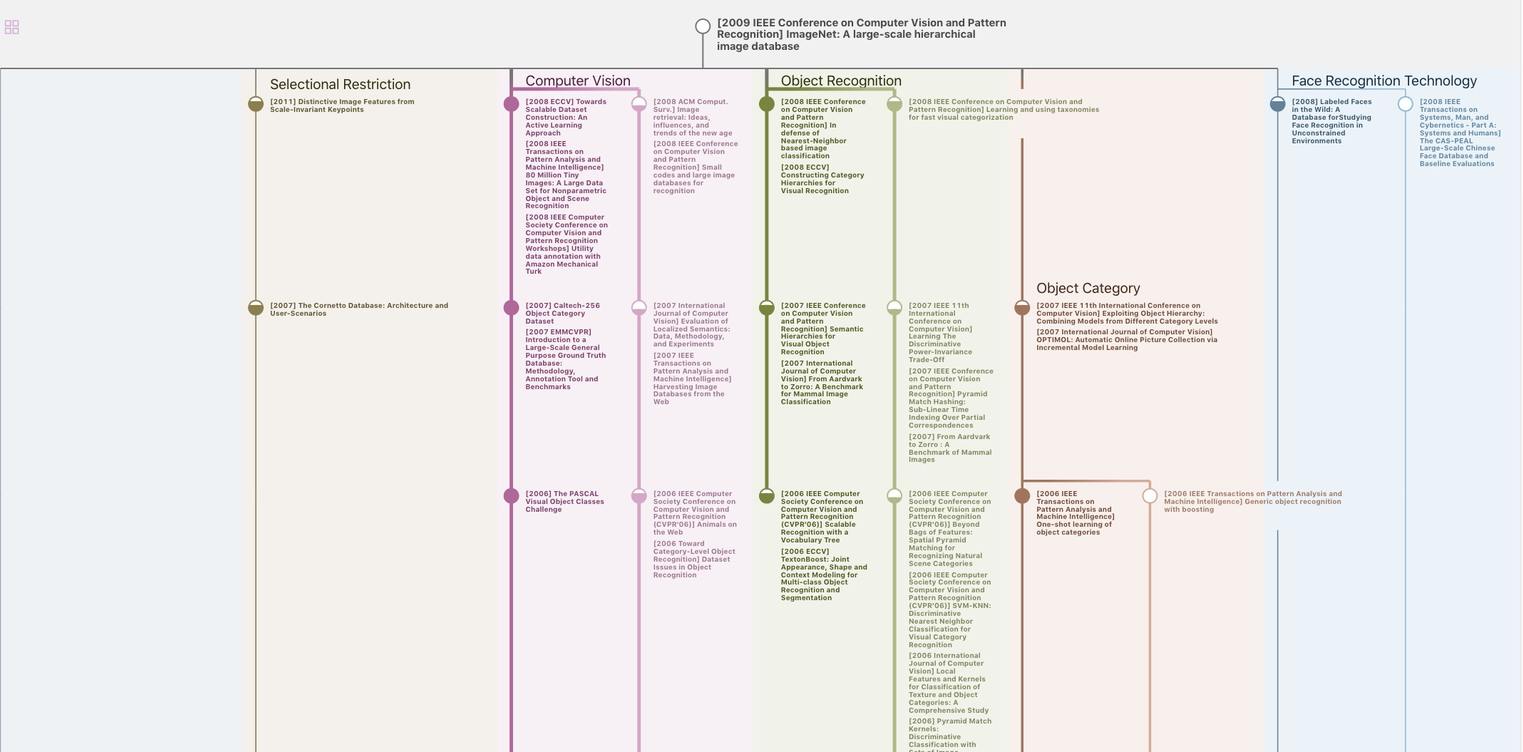
生成溯源树,研究论文发展脉络
Chat Paper
正在生成论文摘要