Scene-Driven Multimodal Knowledge Graph Construction for Embodied AI
arXiv (Cornell University)(2023)
摘要
Embodied AI is one of the most popular studies in artificial intelligence and robotics, which can effectively improve the intelligence of real-world agents (i.e. robots) serving human beings. Scene knowledge is important for an agent to understand the surroundings and make correct decisions in the varied open world. Currently, knowledge base for embodied tasks is missing and most existing work use general knowledge base or pre-trained models to enhance the intelligence of an agent. For conventional knowledge base, it is sparse, insufficient in capacity and cost in data collection. For pre-trained models, they face the uncertainty of knowledge and hard maintenance. To overcome the challenges of scene knowledge, we propose a scene-driven multimodal knowledge graph (Scene-MMKG) construction method combining conventional knowledge engineering and large language models. A unified scene knowledge injection framework is introduced for knowledge representation. To evaluate the advantages of our proposed method, we instantiate Scene-MMKG considering typical indoor robotic functionalities (Manipulation and Mobility), named ManipMob-MMKG. Comparisons in characteristics indicate our instantiated ManipMob-MMKG has broad superiority in data-collection efficiency and knowledge quality. Experimental results on typical embodied tasks show that knowledge-enhanced methods using our instantiated ManipMob-MMKG can improve the performance obviously without re-designing model structures complexly. Our project can be found at https://sites.google.com/view/manipmob-mmkg
更多查看译文
关键词
multimodal knowledge graph construction,knowledge graph,ai,scene-driven
AI 理解论文
溯源树
样例
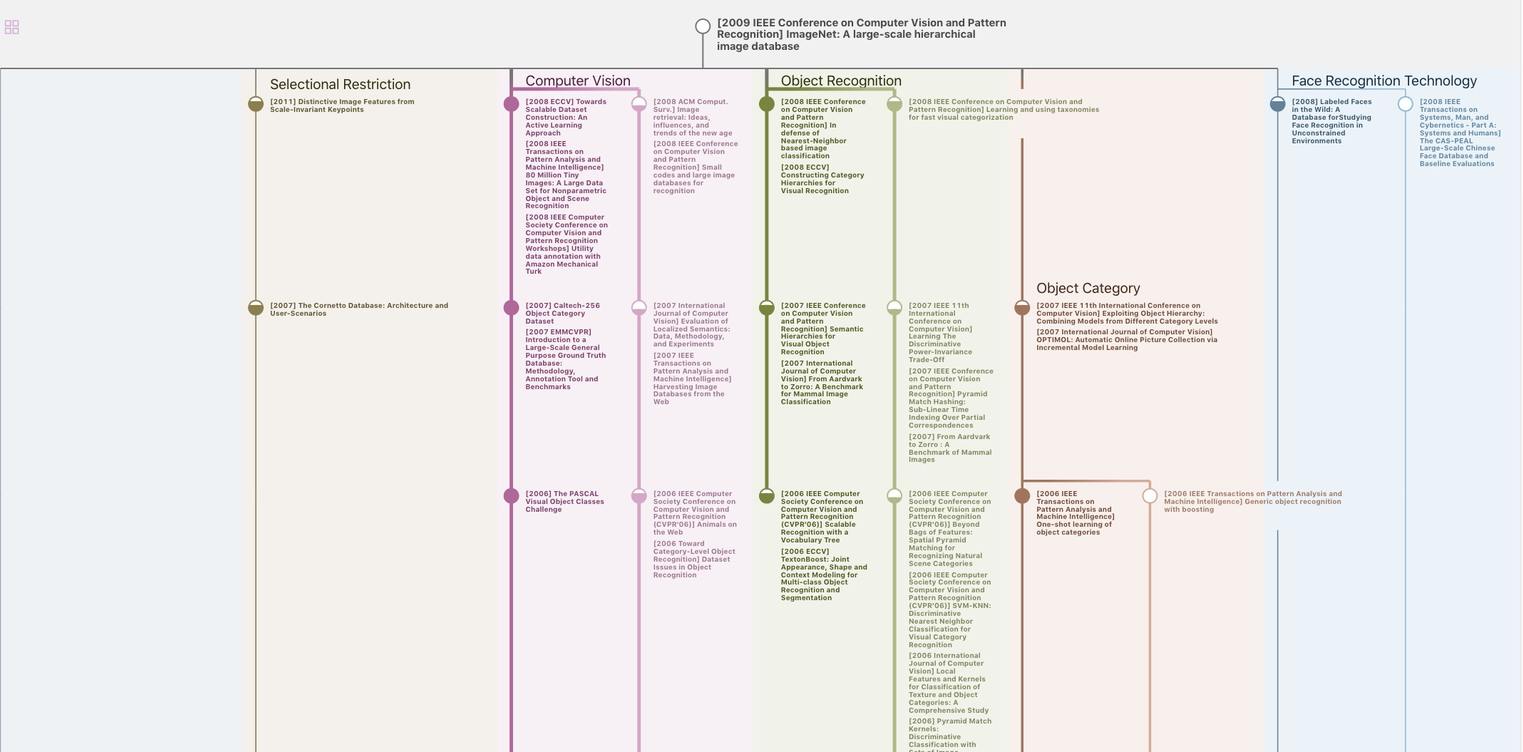
生成溯源树,研究论文发展脉络
Chat Paper
正在生成论文摘要