MDE and Learning for flexible Planning and optimized Execution of Multi-Robot Choreographies.
ETFA(2023)
摘要
Multi-Robot systems in automotive are safety-critical systems that consist of collaborating-aware robots and components that interact with external components, the environment, or humans at run-time. This implies a significant complexity for the system engineer to design, model, validate the system, and optimize the cycle time, including considering unexpected events at run-time. This paper addresses this challenge by describing a model-driven engineering approach that formally designs the system under the consideration of uncertainties and at run-time optimizes the system actions using learning-based approaches. We implemented this approach in an industrial-inspired case study of a spot-welding multi-robot cell. Based on the system requirements, we generate valid system strategies that consider unexpected events such as robot interruptions and failures. Considering movement and interruption time models, we implemented a reinforcement learning method to optimize system actions at run-time. We show that via simulations and learning, our approach can be used to synthesize time-efficient schedules for robot task assignments that improve the overall cycle time.
更多查看译文
关键词
Motion planning,Task planning,Choreography planning,Learning-based scheduling under uncertainty
AI 理解论文
溯源树
样例
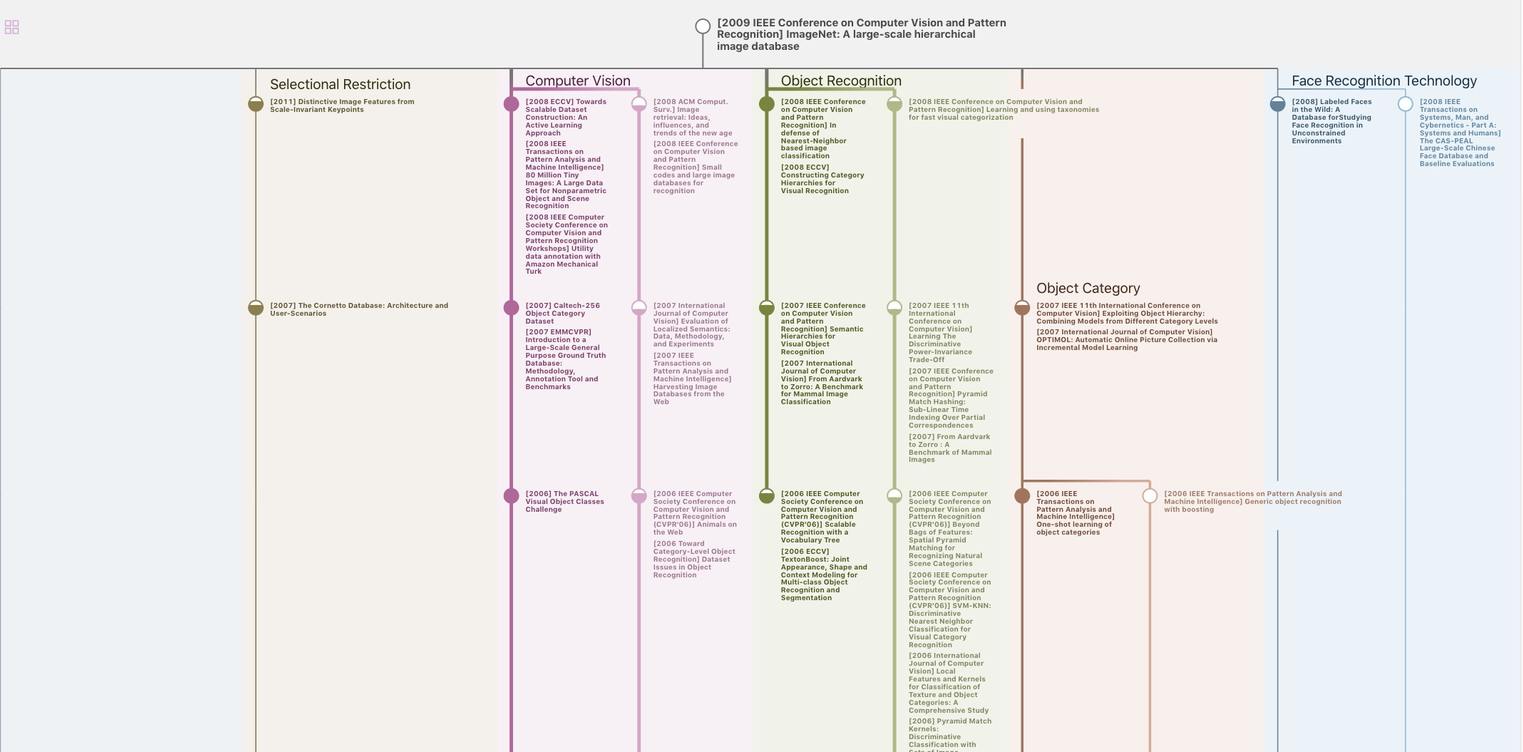
生成溯源树,研究论文发展脉络
Chat Paper
正在生成论文摘要