A Lightweight Deep Learning Solution for mmWave Human Activity Recognition in Smart Health based on Discrete Fourier Transformation
ICC 2023 - IEEE INTERNATIONAL CONFERENCE ON COMMUNICATIONS(2023)
摘要
Millimeter wave (mmWave) based human activity recognition is important in smart health in terms of studying user lifestyle. In practical health IoT scenarios, fast and accurate human activity recognition is critically important. In this work, we design and implement a lightweight deep learning solution for human activity recognition based on discrete Fourier transformation. The model has a fairly small number of model parameters while offering high accuracy in activity recognition. The core of the solution is a discrete Fourier transform module inside a neural network, which converts the temporal features of mmWave radar activity data into frequency features before activity recognition is performed by a simple classifier. We have extensively evaluated this solution against other traditional deep learning models in mmWave human activity recognition. The evaluation demonstrates that the DFT-based network can achieve the same accuracy as other traditional neural network models, but with a very small computational load.
更多查看译文
AI 理解论文
溯源树
样例
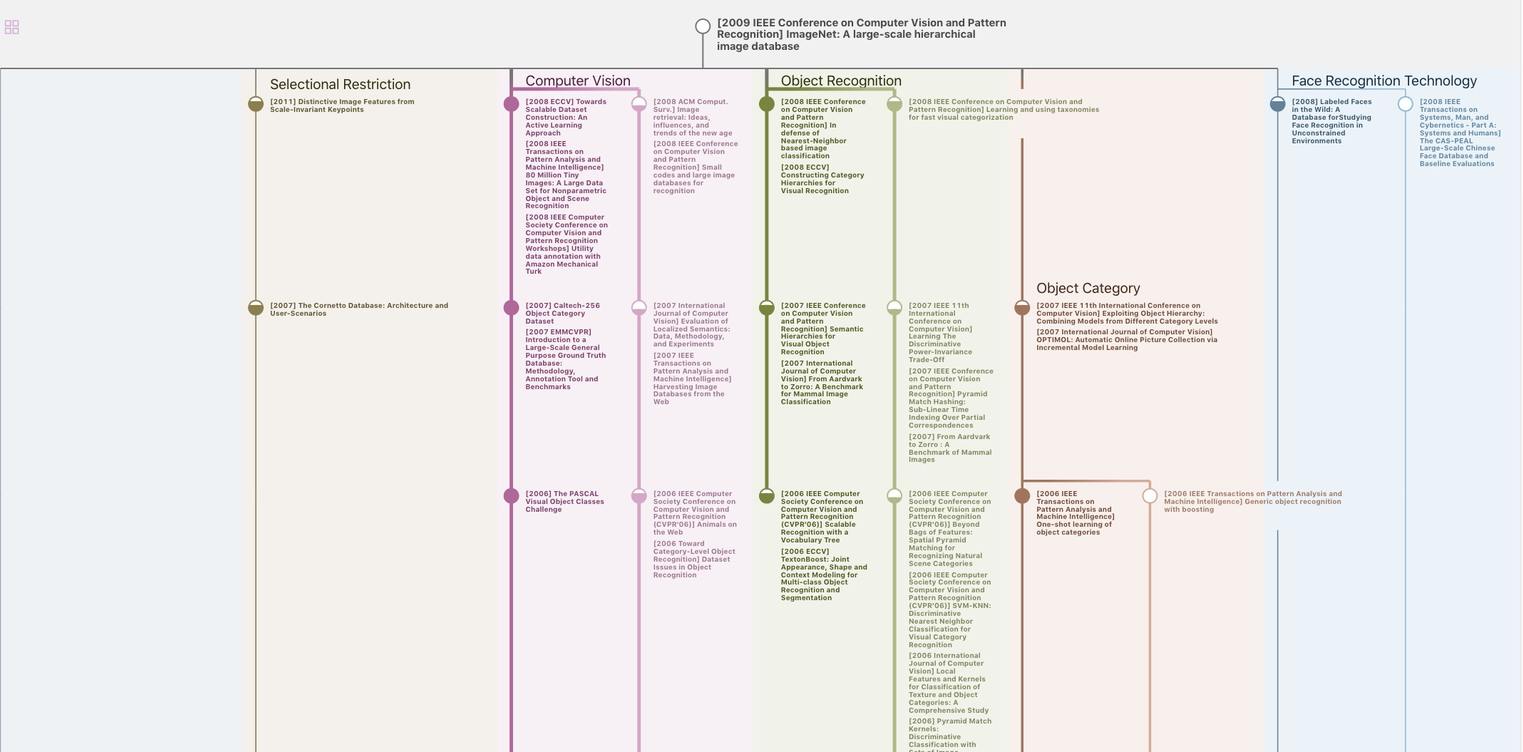
生成溯源树,研究论文发展脉络
Chat Paper
正在生成论文摘要