Online Learning-Based Beamforming for Rate-Splitting Multiple Access: A Constrained Bandit Approach
ICC 2023 - IEEE INTERNATIONAL CONFERENCE ON COMMUNICATIONS(2023)
摘要
Rate-splitting multiple access (RSMA) has emerged as a potential non-orthogonal transmission strategy and powerful interference management scheme for 6G. Most of the existing works on RSMA beamforming design assume instantaneous or statistical channel state information (CSI) is available at the transmitter. Such an assumption however is impractical especially in massive multiple-input multiple-output (MIMO) due to the dynamic wireless environments and the challenges in channel estimation. In this work, we propose a novel beamforming design framework based on online learning and online control to adaptively learn the best precoding action for a RSMA-aided downlink massive MIMO without explicit CSI feedback. In particular, we first formulate the precoder selection problem that maximizes the ergodic sum-rate subject to a long-term transmit power constraint as a constrained combinatorial multi-armed bandit (CMAB) problem. Then we propose a precoder selection with bandit learning algorithm for RSMA (PBR). Our theoretical analysis shows that PBR achieves a sublinear regret bound with a long-term power constraint guarantee. Through experimental results, we not only verify our theoretical analysis but also demonstrate the outperformance of PBR in terms of sum-rate and power consumption compared with the conventional transmission schemes without using RSMA.
更多查看译文
AI 理解论文
溯源树
样例
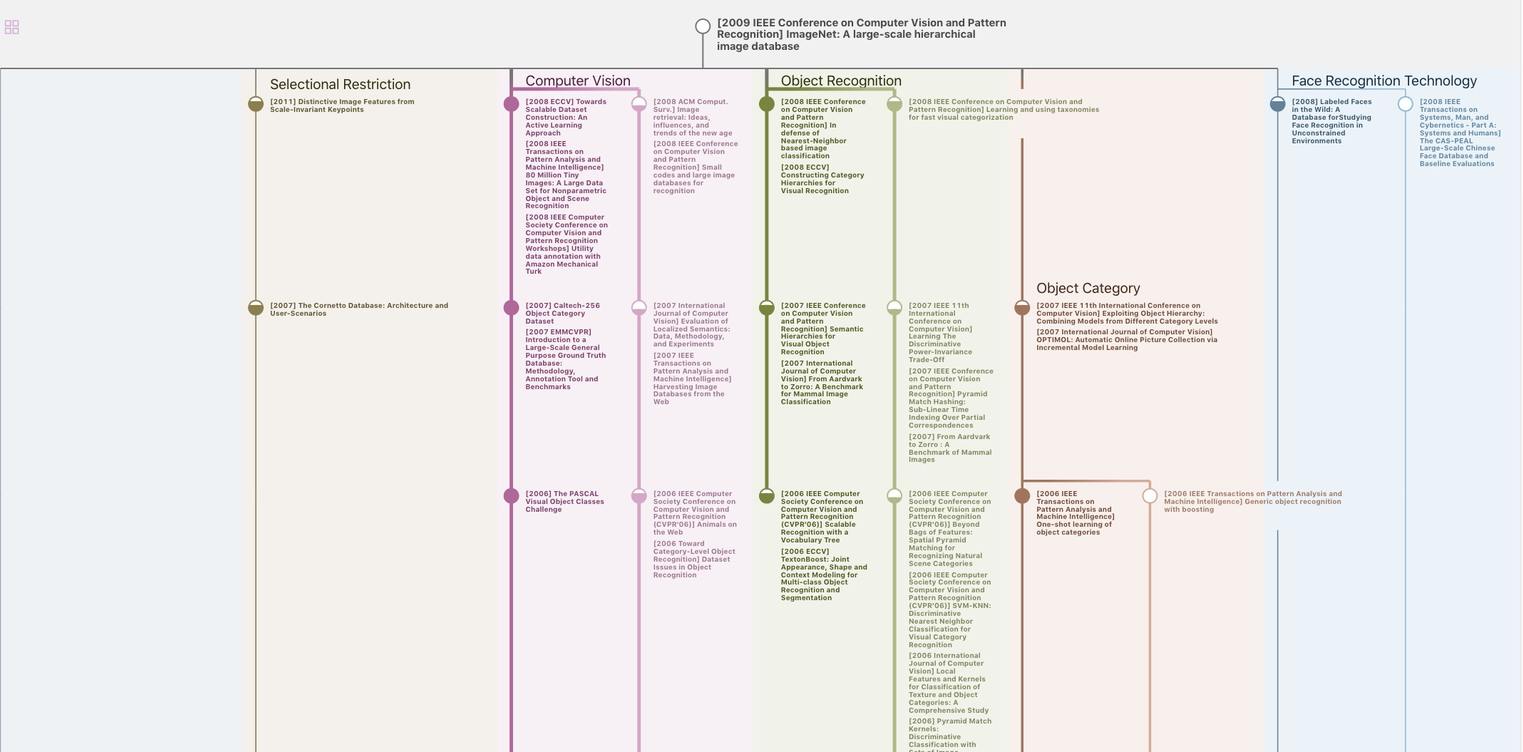
生成溯源树,研究论文发展脉络
Chat Paper
正在生成论文摘要