Domain Disentangled Meta-Learning.
SDM(2023)
摘要
A key challenge with supervised learning (e.g., image classification) is the shift of data distribution and domain from training to testing datasets, so-called “domain shift” (or “distribution shift”), which usually leads to a reduction of model accuracy. Various meta-learning approaches have been proposed to prevent the accuracy loss by learning an adaptable model with training data, and adapting it to test time data from a new data domain. However, when the domain shift occurs in multiple domain dimensions (e.g., images may be transformed by rotations, transitions, and expansions), the average predictive power of the adapted model will deteriorate. To tackle this problem, we propose a domain disentangled meta-learning (DDML) framework. DDML disentangles the data domain by dimensions, learns the representations of domain dimensions independently, and adapts to the domain of test time data. We evaluate our DDML on image classification problems using three datasets with distribution shifts over multiple domain dimensions. Comparing to various baselines in meta-learning and empirical risk minimization, our DDML approach achieves consistently higher classification accuracy with the test time data. These results demonstrate that domain disentanglement reduces the complexity of the model adaptation, thus increases the model generalizability, and prevents it from overfitting.
更多查看译文
关键词
domain,meta-learning
AI 理解论文
溯源树
样例
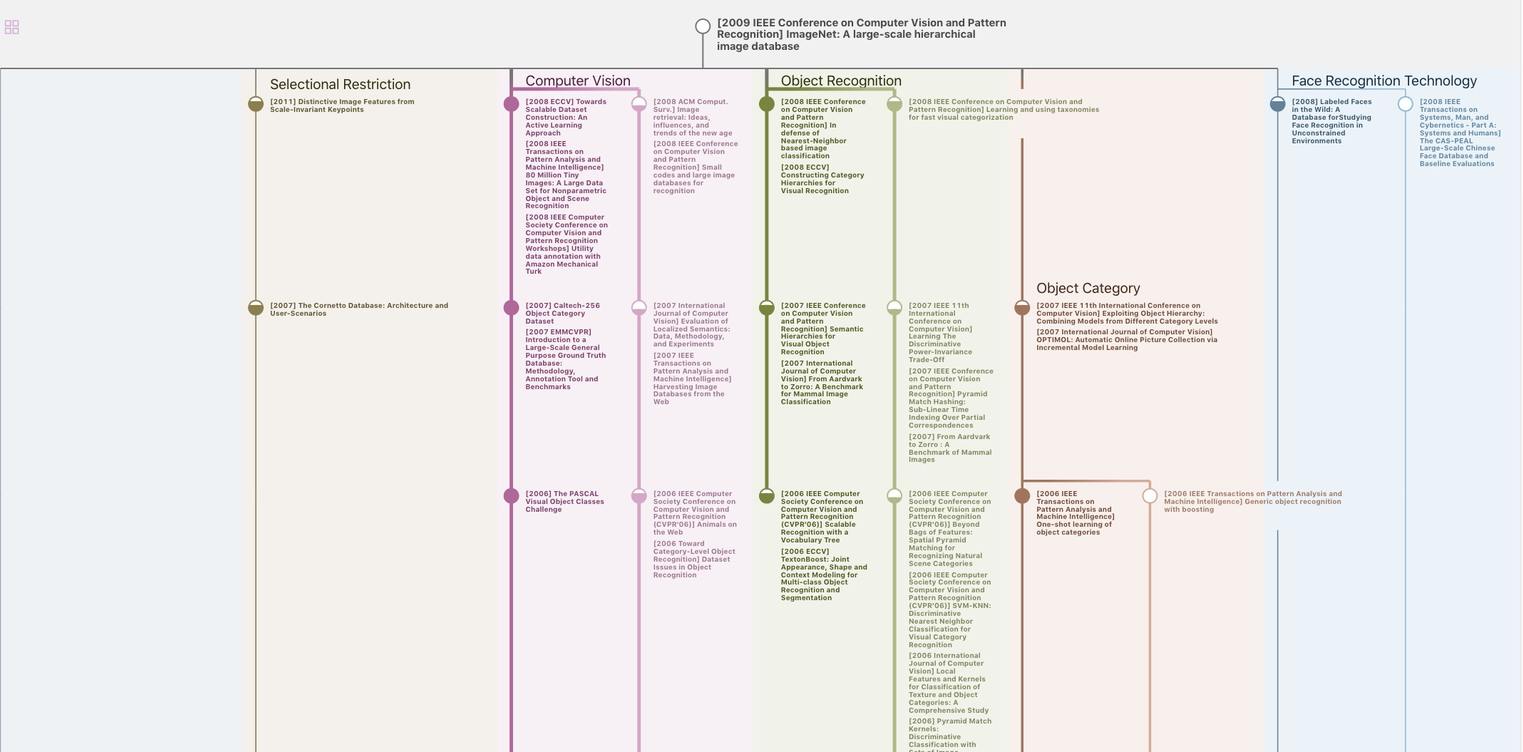
生成溯源树,研究论文发展脉络
Chat Paper
正在生成论文摘要