Attentive Deep Canonical Correlation Analysis for Diagnosing Alzheimer's Disease Using Multimodal Imaging Genetics
MEDICAL IMAGE COMPUTING AND COMPUTER ASSISTED INTERVENTION, MICCAI 2023, PT II(2023)
摘要
Integration of imaging genetics data provides unprecedented opportunities for revealing biological mechanisms underpinning diseases and certain phenotypes. In this paper, a new model called attentive deep canonical correlation analysis (ADCCA) is proposed for the diagnosis of Alzheimer's disease using multimodal brain imaging genetics data. ADCCA combines the strengths of deep neural networks, attention mechanisms, and canonical correlation analysis to integrate and exploit the complementary information from multiple data modalities. This leads to improved interpretability and strong multimodal feature learning ability. The ADCCA model is evaluated using the ADNI database with three imaging modalities (VBM-MRI, FDG-PET, and AV45-PET) and genetic SNP data. The results indicate that this approach can achieve outstanding performance and identify meaningful biomarkers for Alzheimer's disease diagnosis. To promote reproducibility, the code has been made publicly available at https://github.com/rongzhou7/ADCCA.
更多查看译文
关键词
Brain imaging genetics,Canonical correlation analysis,Self-attention,Alzheimer's disease
AI 理解论文
溯源树
样例
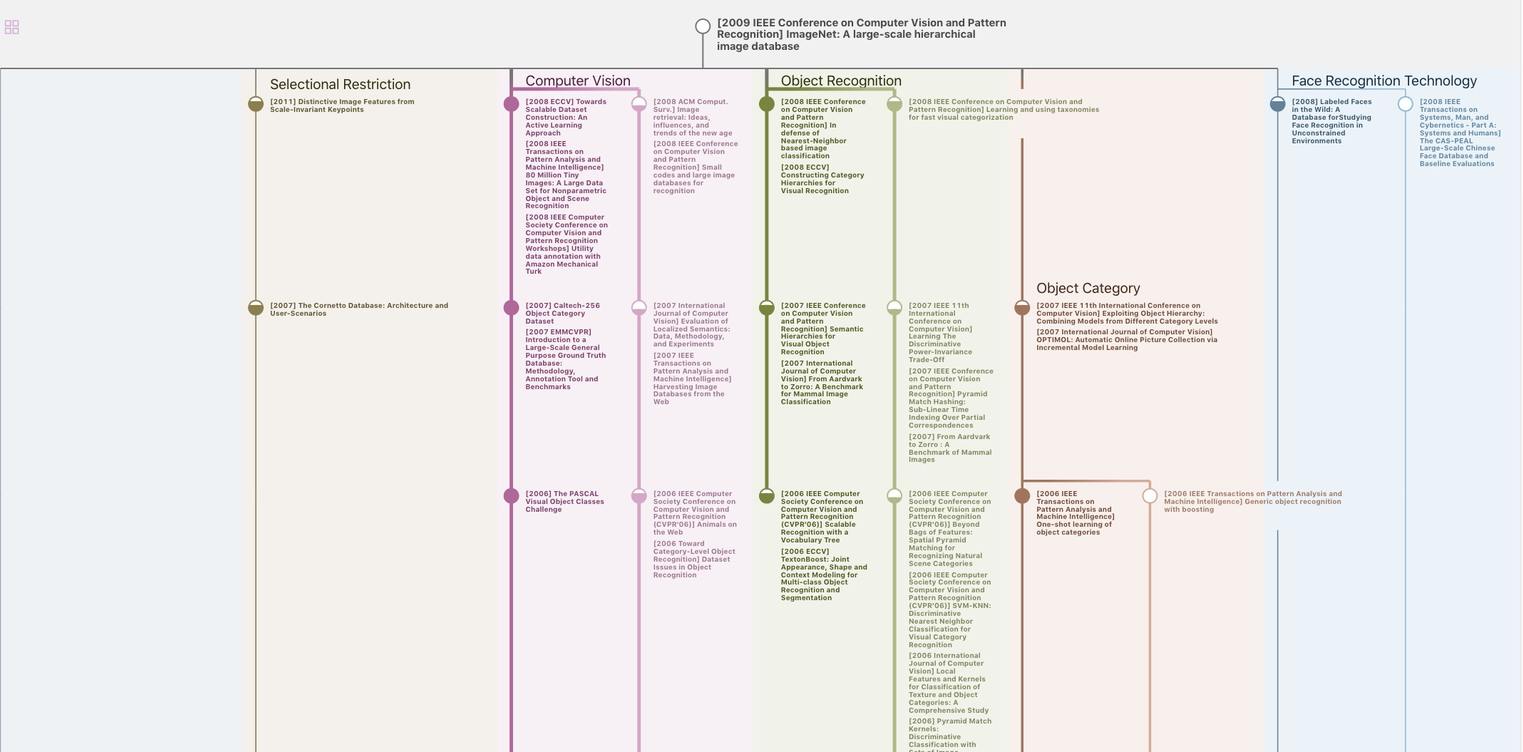
生成溯源树,研究论文发展脉络
Chat Paper
正在生成论文摘要