Full uncertainty analysis for Bayesian nonparametric mixture models
COMPUTATIONAL STATISTICS & DATA ANALYSIS(2024)
摘要
A full posterior analysis for nonparametric mixture models using Gibbs-type prior distributions is presented. This includes the well known Dirichlet process mixture (DPM) model. The random mixing distribution is removed enabling a simple-to-implement Markov chain Monte Carlo (MCMC) algorithm. The removal procedure takes away some of the posterior uncertainty and how it is replaced forms a novel aspect to the work. The removal, MCMC algorithm and replacement of the uncertainty only require the probabilities of a new or an old value associated with the corresponding Gibbs-type exchangeable sequence. Consequently, no explicit representations of the prior or posterior are required and instead only knowledge of the exchangeable sequence is needed. This allows the implementation of mixture models with full posterior uncertainty, not previously possible, including one introduced by Gnedin. Numerous illustrations are presented, as is an R-package called CopRe which implements the methodology, and other supplemental material.(c) 2023 Elsevier B.V. All rights reserved.
更多查看译文
关键词
Exchangeability,Gibbs -type model,Mixture model
AI 理解论文
溯源树
样例
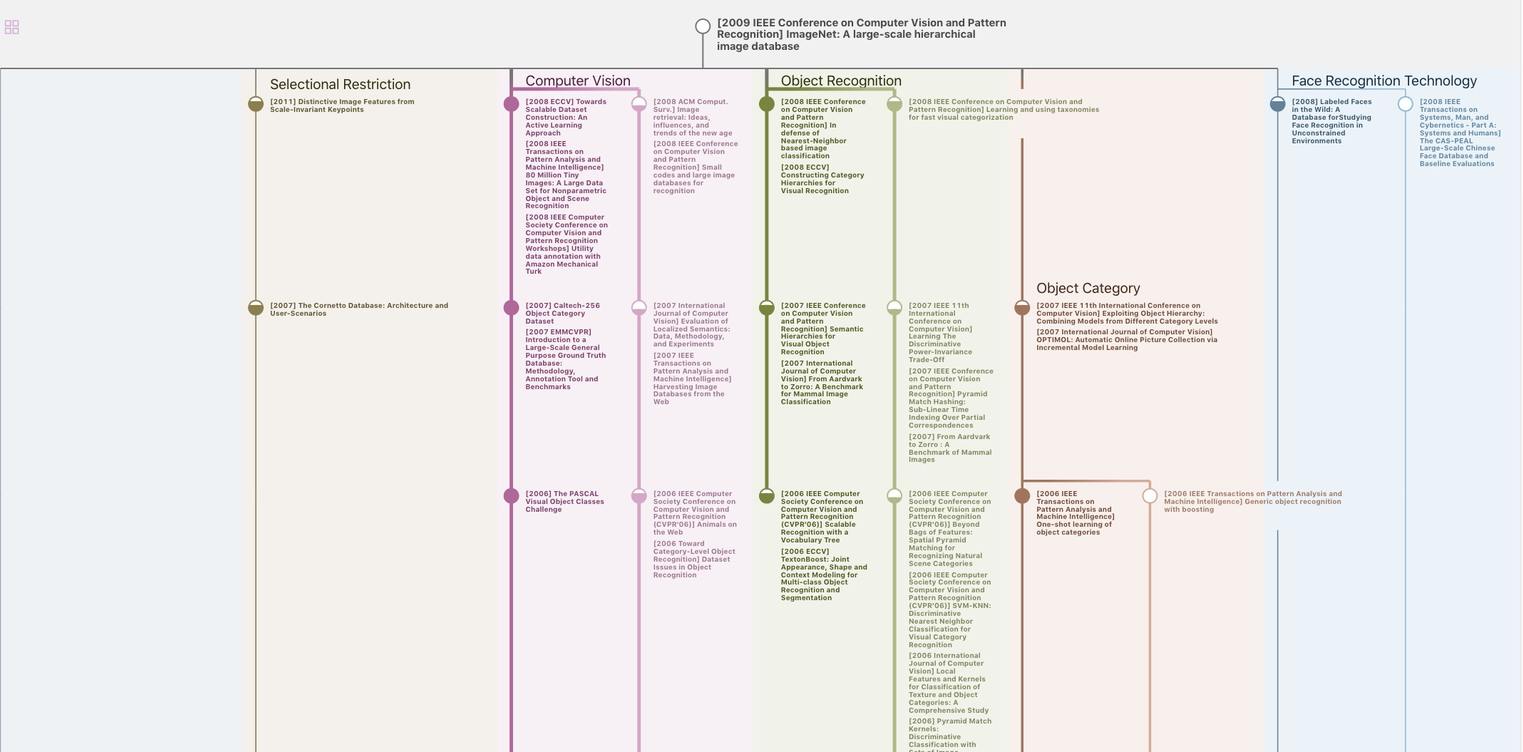
生成溯源树,研究论文发展脉络
Chat Paper
正在生成论文摘要