AFALog: A General Augmentation Framework for Log-based Anomaly Detection with Active Learning
2023 IEEE 34th International Symposium on Software Reliability Engineering (ISSRE)(2023)
摘要
Log-based anomaly detection is becoming more and more important for maintaining the availability of modern microservice systems. Existing supervised/semi-supervised log anomaly detection models require a large amount of human-labeled logs for training which are hard to collect in real-world systems. Unsupervised models often perform poorly without explicit anomaly labels. To improve the performance of unsupervised models, in this paper, we first make an empirical study of existing unsupervised models to tackle the reason why they often produce unsatisfied results. We find that anomaly detection results produced by existing unsupervised models are significantly affected by two key problems including Not-Cover (NC) problem and Suspicious-Noise (SN) problem. To solve these problems, we propose a novel augmentation framework called AFALog. AFALog leverages the idea of active learning to incorporate human knowledge so as to augment data quality. It can support almost all existing unsupervised models and improve their performance. Our experiments on two open datasets and one dataset collected from a real-world microservice system demonstrate that DALog improves the F1-score by an average of 6.61%, with only 5.9% labeled training data.
更多查看译文
关键词
Anomaly Detection,Augmentation,Log Analysis,Active Learning,Deep Learning
AI 理解论文
溯源树
样例
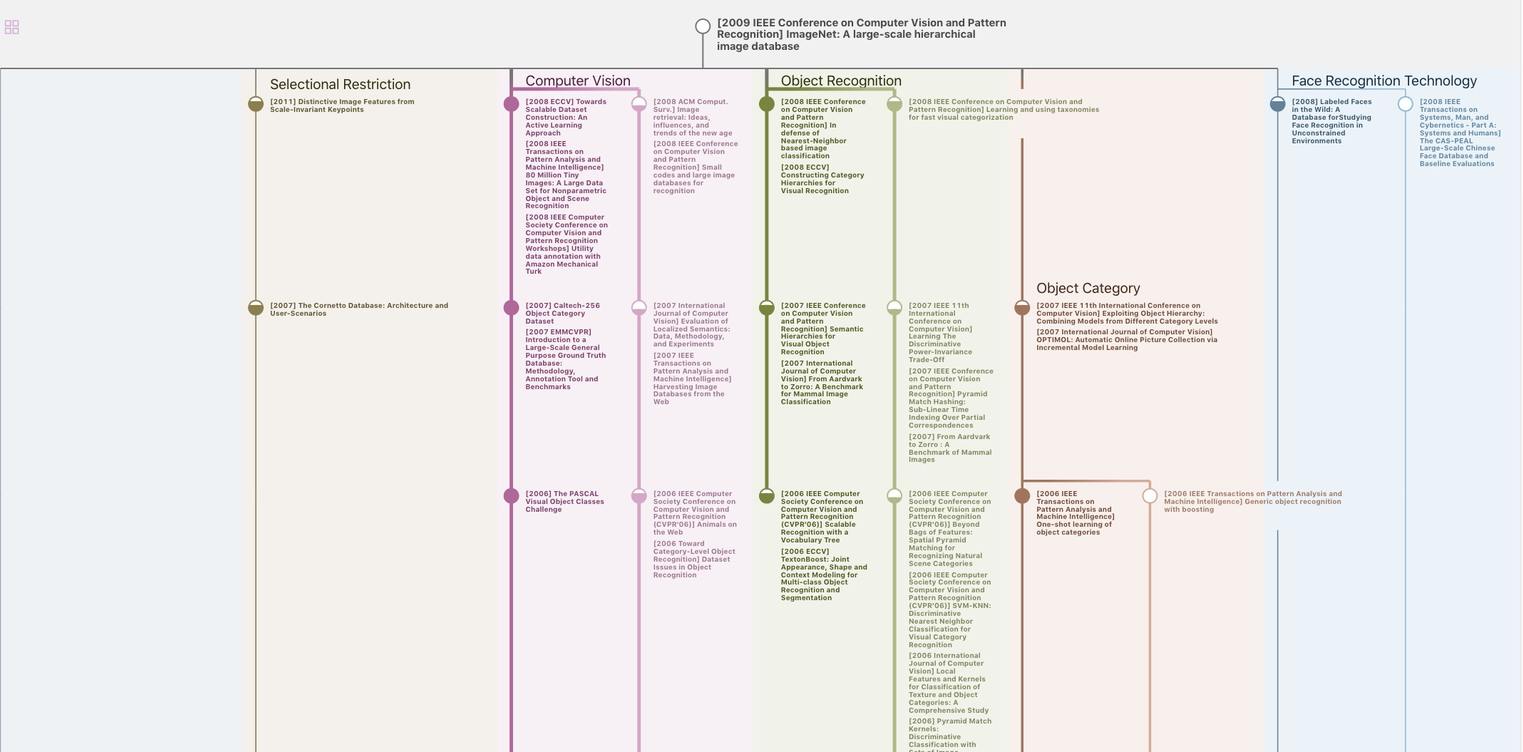
生成溯源树,研究论文发展脉络
Chat Paper
正在生成论文摘要