N-epitomizer: Enabling Semantic Offloading for Neural Network Inferences
2023 IEEE 20th International Conference on Mobile Ad Hoc and Smart Systems (MASS)(2023)
摘要
Offloading neural network Inferences from resource- constrained mobile devices to an edge server over wireless networks is becoming more crucial as the neural networks get heavier. To this end, recent studies have tried to make this offloading process more efficient. However, the most fundamental question on extracting and offloading the minimal amount of necessary information that does not degrade the inference accuracy has remained unanswered. We call such an ideal offloading semantic offloading and propose N-epitomizer, a new offloading framework that enables semantic offloading, thus achieving more reliable and timely inferences in highly-fluctuated or even low- bandwidth wireless networks. To realize N-epitomizer, we design an autoencoder-based scalable encoder trained to extract the most informative data and scale its output size to meet the latency and accuracy requirements of inferences over a network. We also accelerate N-epitomizer by exploiting light-weight knowledge distillation for the encoder design and decoder slimming for the decoder design, reducing its overall computation time significantly. Our evaluation shows that N-epitomizer achieves exceptionally high compression for images without compromising inference accuracy, which is 21×, 77×, and 192× higher than JPEG compression, and 20×, 55×, and 86× higher than the state-of-the-art DNN-aware image compression GRACE [1] for semantic segmentation, depth estimation, and classification, respectively. Our results show N-epitomizer’s strong potential as the first semantic offloading system to guarantee end-to-end latency even under highly varying cellular networks.
更多查看译文
关键词
DNN-aware codec,Compressive transmission,Offloading,Semantic communication
AI 理解论文
溯源树
样例
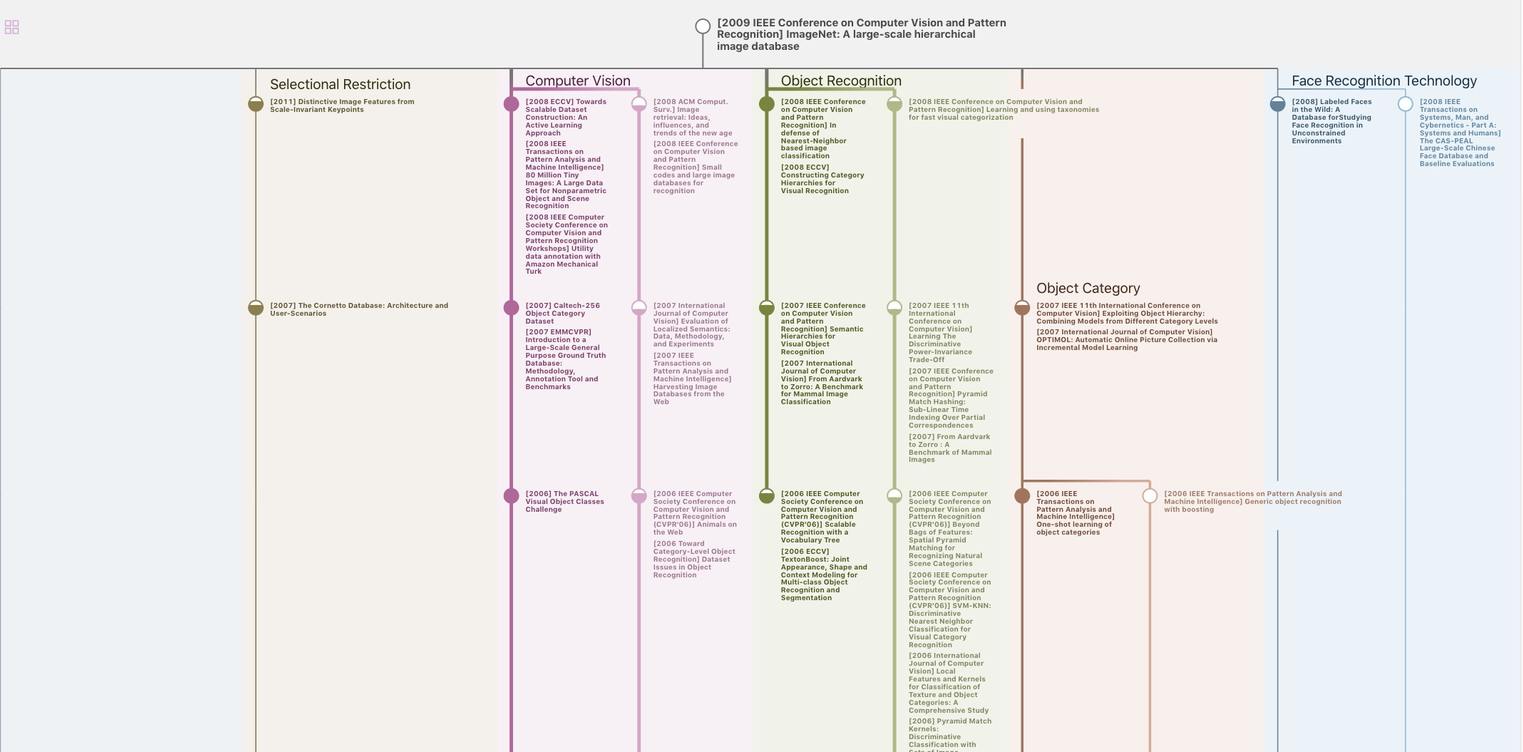
生成溯源树,研究论文发展脉络
Chat Paper
正在生成论文摘要