Transformers are Efficient In-Context Estimators for Wireless Communication.
CoRR(2023)
摘要
Pre-trained transformers can perform in-context learning, where they adapt to a new task using only a small number of prompts without any explicit model optimization. Inspired by this attribute, we propose a novel approach, called in-context estimation, for the canonical communication problem of estimating transmitted symbols from received symbols. A communication channel is essentially a noisy function that maps transmitted symbols to received symbols, and this function can be represented by an unknown parameter whose statistics depend on an (also unknown) latent context. Conventional approaches ignore this hierarchical structure and simply attempt to use known transmissions, called pilots, to perform a least-squares estimate of the channel parameter, which is then used to estimate successive, unknown transmitted symbols. We make the basic connection that transformers show excellent contextual sequence completion with a few prompts, and so they should be able to implicitly determine the latent context from pilot symbols to perform end-to-end in-context estimation of transmitted symbols. Furthermore, the transformer should use information efficiently, i.e., it should utilize any pilots received to attain the best possible symbol estimates. Through extensive simulations, we show that in-context estimation not only significantly outperforms standard approaches, but also achieves the same performance as an estimator with perfect knowledge of the latent context within a few context examples. Thus, we make a strong case that transformers are efficient in-context estimators in the communication setting.
更多查看译文
关键词
wireless
AI 理解论文
溯源树
样例
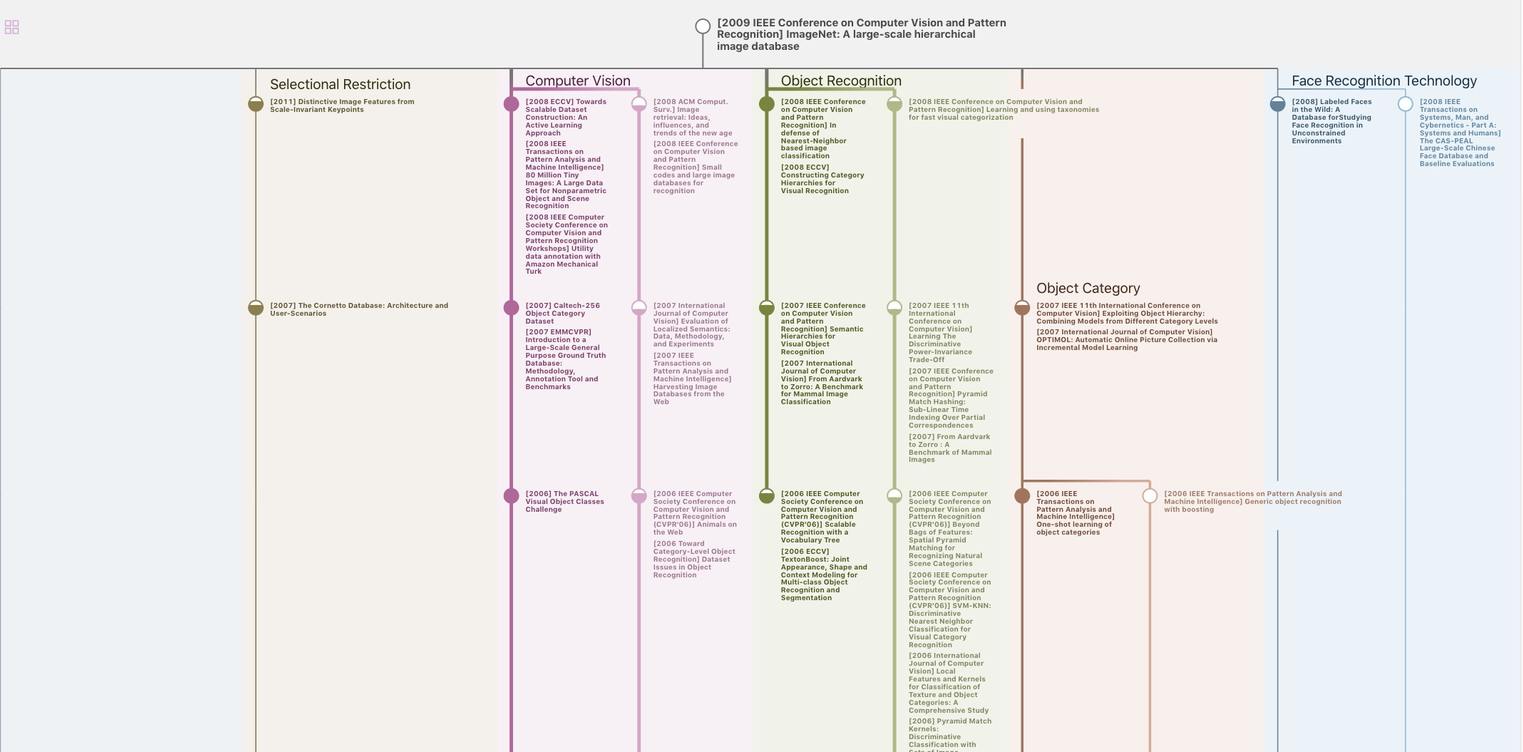
生成溯源树,研究论文发展脉络
Chat Paper
正在生成论文摘要