The Expressive Power of Low-Rank Adaptation
ICLR 2024(2023)
摘要
Low-Rank Adaptation (LoRA), a parameter-efficient fine-tuning method that leverages low-rank adaptation of weight matrices, has emerged as a prevalent technique for fine-tuning pre-trained models such as large language models and diffusion models. Despite its huge success in practice, the theoretical underpinnings of LoRA have largely remained unexplored. This paper takes the first step to bridge this gap by theoretically analyzing the expressive power of LoRA. We prove that, for fully connected neural networks, LoRA can adapt any model $f$ to accurately represent any smaller target model $\overline{f}$ if LoRA-rank $\geq(\text{width of }f) \times \frac{\text{depth of }\overline{f}}{\text{depth of }f}$. We also quantify the approximation error when LoRA-rank is lower than the threshold. For Transformer networks, we show any model can be adapted to a target model of the same size with rank-$(\frac{\text{embedding size}}{2})$ LoRA adapters.
更多查看译文
关键词
LoRA,expressive power,parameter-efficient fine-tuning,adaptation,neural networks,transformer
AI 理解论文
溯源树
样例
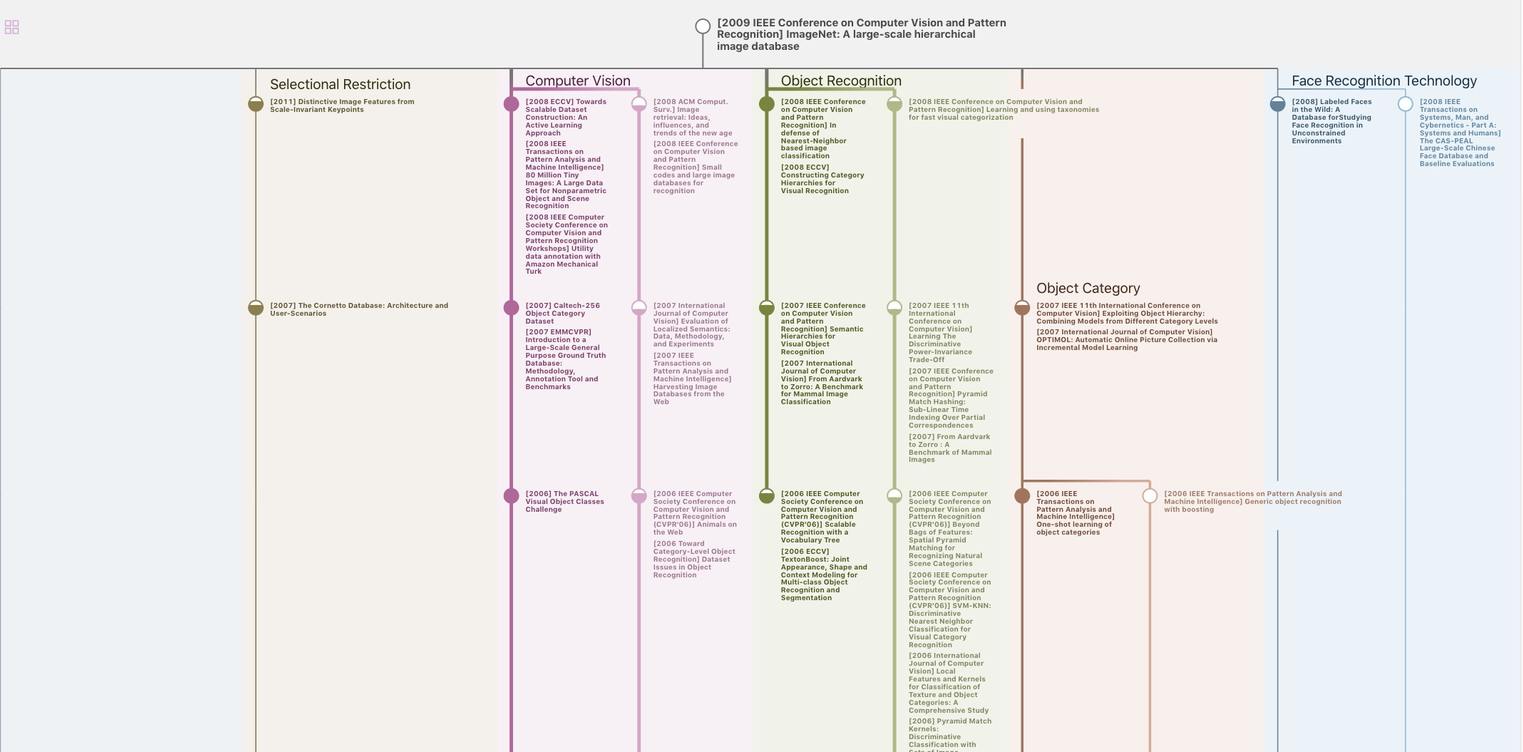
生成溯源树,研究论文发展脉络
Chat Paper
正在生成论文摘要