Digital Twin-Empowered Resource Allocation for 6G-Enabled Massive IoT
2023 IEEE INTERNATIONAL CONFERENCE ON COMMUNICATIONS WORKSHOPS, ICC WORKSHOPS(2023)
摘要
6G technology is expected to lead to an unpredictable increase of Internet of Things (IoT) devices. The need for maintaining continuous connectivity of these devices has in turn led to re-thinking of the traditional design of wireless networks. In particular, the integration of 6G and Digital Twin (DT) is expected to reshape the network management as it offers powerful features in design, development and optimization processes. DT is a digital representation of physical entities, which are designed around a two-way information flow. Therefore, this technology not only collects data, and employs intelligent learning methods by performing complex computations, but it also can send feedback to improve system performance for 6G-enabled massive IoT. However, deploying such a technology requires addressing complex challenges such as limited resources, seamless connectivity and lack of trust between end users and network edge. To address these challenges, we formulate the resource allocation problem including edge computation and service migration in 6G-enabled massive IoT. The contributions are threefold: First, our DT-empowered architecture is proposed that uses the realtime and historical data from end users to find the best allocation at a user. Second, it studies the impact of trust relationship between computing entities to prevent the unauthorized accesses and provides an authentication procedure. Third, it describes a Multi-Agent Reinforcement Learning (MARL) algorithm that consists of cooperative agents and aims to find the best resource allocation strategy by minimizing task processing latency. We validate the proposed DT-empowered architecture to show the reduced processing latency compared to traditional benchmark methods.
更多查看译文
AI 理解论文
溯源树
样例
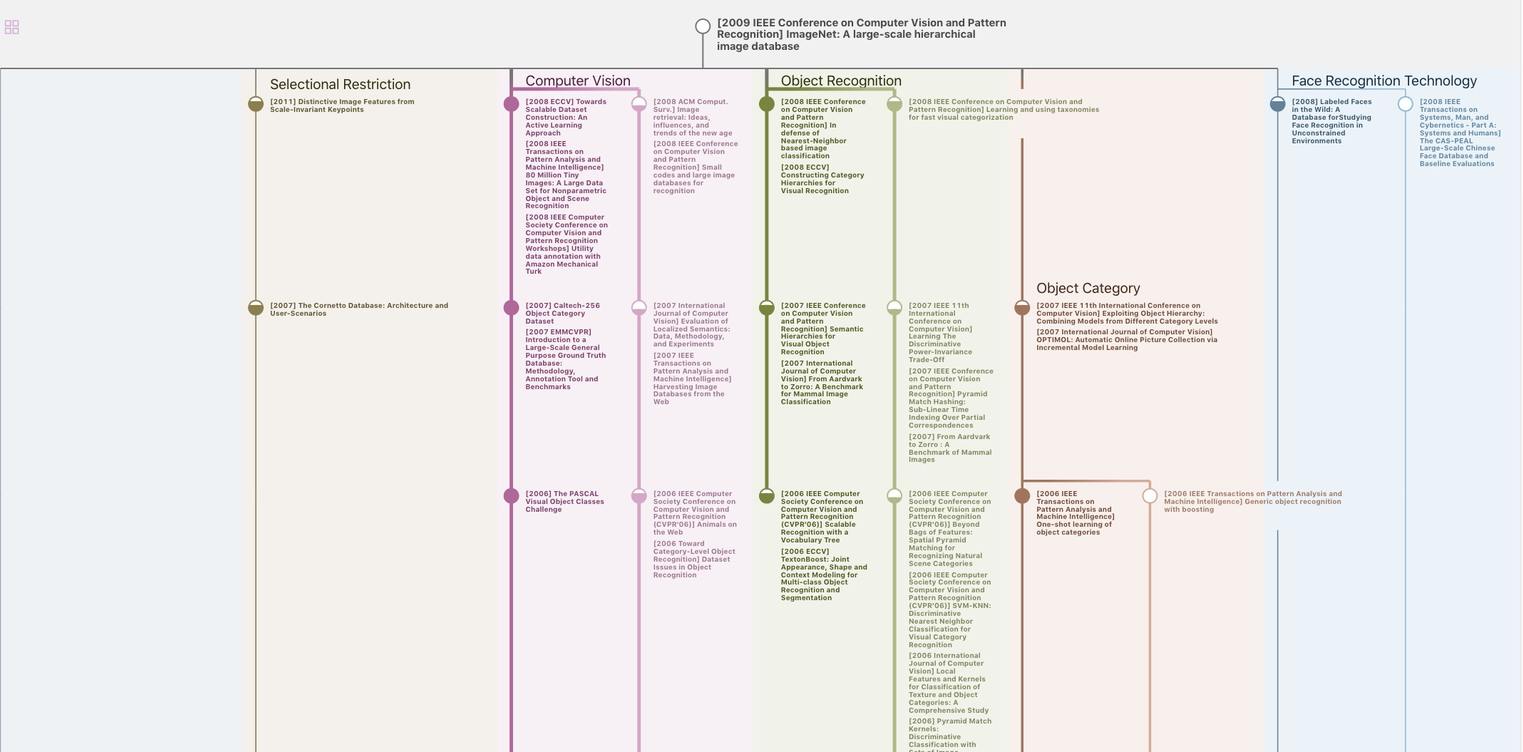
生成溯源树,研究论文发展脉络
Chat Paper
正在生成论文摘要