Multi-Modal Meta-Transfer Fusion Network for Few-Shot 3D Model Classification
International Journal of Computer Vision(2024)
摘要
Nowadays, driven by the increasing concern on 3D techniques, resulting in the large-scale 3D data, 3D model classification has attracted enormous attention from both research and industry communities. Most of the current methods highly depend on sufficient labeled 3D models, which substantially restricts their scalability to novel classes with few annotated training data since it can increase the chance of overfitting. Besides, they only leverage single-modal information (either point cloud or multi-view information), and few works integrate these complementary information for 3D model representation. To overcome these problems, we propose a multi-modal meta-transfer fusion network (M ^3 TF), the key of which is to perform few-shot multi-modal representation for 3D model classification. Specifically, we first convert the original 3D data into both multi-view and point cloud modalities, and pre-train individual encoding networks on a large-scale dataset to obtain the optimal initial parameters, which is beneficial to few-shot learning tasks. Then, to enable the network to adjust to few-shot learning tasks, we update the parameters in Scaling and Shifting operation ( SS ), multi-modal representation fusion (MMRF) and the 3D model classifier to obtain optimal initialization parameters. Since the large-scale training parameters in feature extractors will increase the chance of overfitting, we freeze the feature extractor and introduce a SS operation to adjust its weights. Specifically, SS can reduce the number of training parameters up to 20
更多查看译文
关键词
3D model classification,Multi-modal,Multi-view,Point cloud
AI 理解论文
溯源树
样例
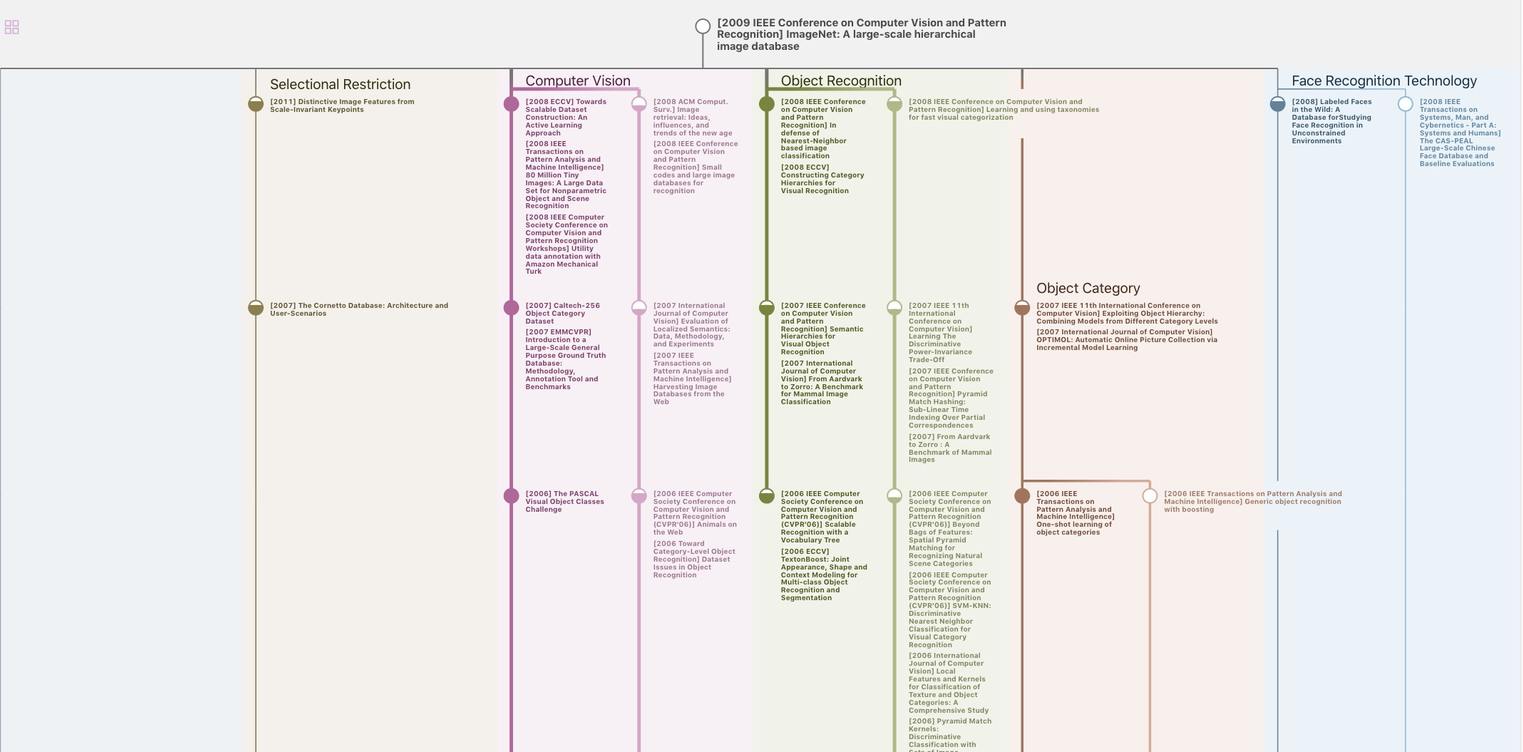
生成溯源树,研究论文发展脉络
Chat Paper
正在生成论文摘要