Revisiting the Efficiency-Accuracy Tradeoff in Adapting Transformer Models via Adversarial Fine-Tuning
ECAI 2023(2023)
摘要
Adversarial fine-tuning (i.e., training on adversarial perturbed inputs) has demonstrated promising results in improving the accuracy of natural language understanding tasks. However, the improved accuracy does not come for free but is accompanied by a significantly prolonged training time, limiting their applicability to larger and more complex models. This work revisits the efficiency-accuracy trade-off in adversarial fine-tuning by systematically analyzing if adversarial fine-tuning methods, in conjunction with several efficiency optimizations, are suitable for adapting pre-trained Transformer models for natural language understanding tasks. Our results show that multiple design choices are crucial in determining the efficiency-accuracy trade-off, and we introduce a method, ScaLA, that achieves better accuracy-vs-speed trade-off than prior methods. We show in experiments that our proposed method attains up to 14.7× adaptation speedups on BERT, RoBERTa, and T5, while achieving comparable accuracy to existing methods.
更多查看译文
关键词
adapting transformer models,efficiency-accuracy,fine-tuning
AI 理解论文
溯源树
样例
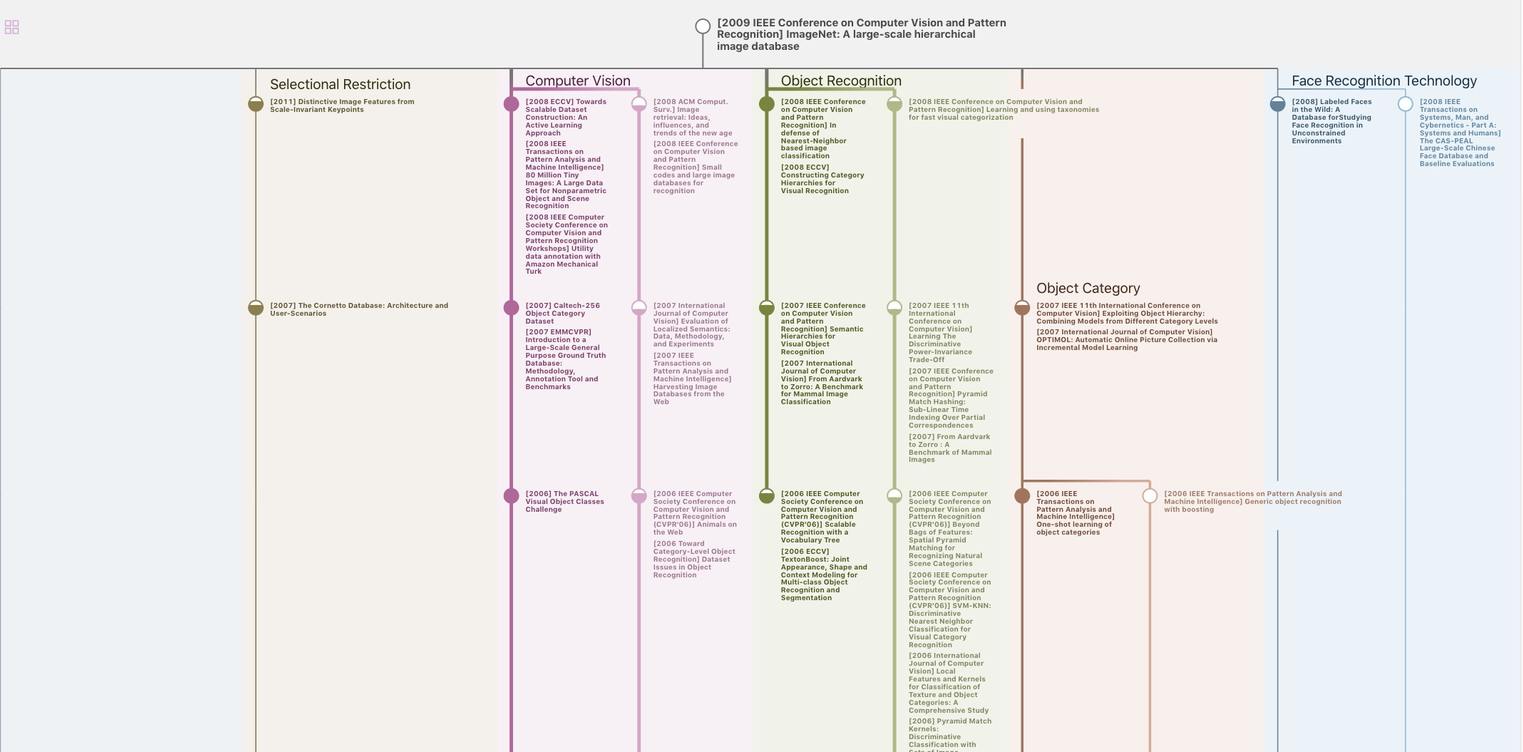
生成溯源树,研究论文发展脉络
Chat Paper
正在生成论文摘要