THUNDER: Named Entity Recognition using a Teacher-student Model with Dual Classifiers for Strong and Weak Supervisions
ECAI 2023(2023)
摘要
Strong and weak supervisions have complementary characteristics. However, utilizing both supervisions for named entity recognition (NER) has not been extensively studied. Moreover, the existing works address only incomplete annotations and neglects inaccurate annotations during NER model training. To effectively utilize weak labels, we introduce an auxiliary classifier that learns from weak labels. Furthermore, we adopt the teacher-student framework to handle both incomplete and inaccurate weak labels. A teacher model is first trained using both strongly and weakly supervised data, and next generates pseudo labels to replace weak labels. Then, the student model is trained so that the main classifier learns from both strong labels and confident pseudo labels while the auxiliary classifier learns from less confident pseudo labels. We also incorporate data augmentation through ChatGPT to generate additional annotated sentences to improve model performance and generalization capabilities. The experimental results with different weak supervisions demonstrate that our proposed method surpasses existing techniques.
更多查看译文
AI 理解论文
溯源树
样例
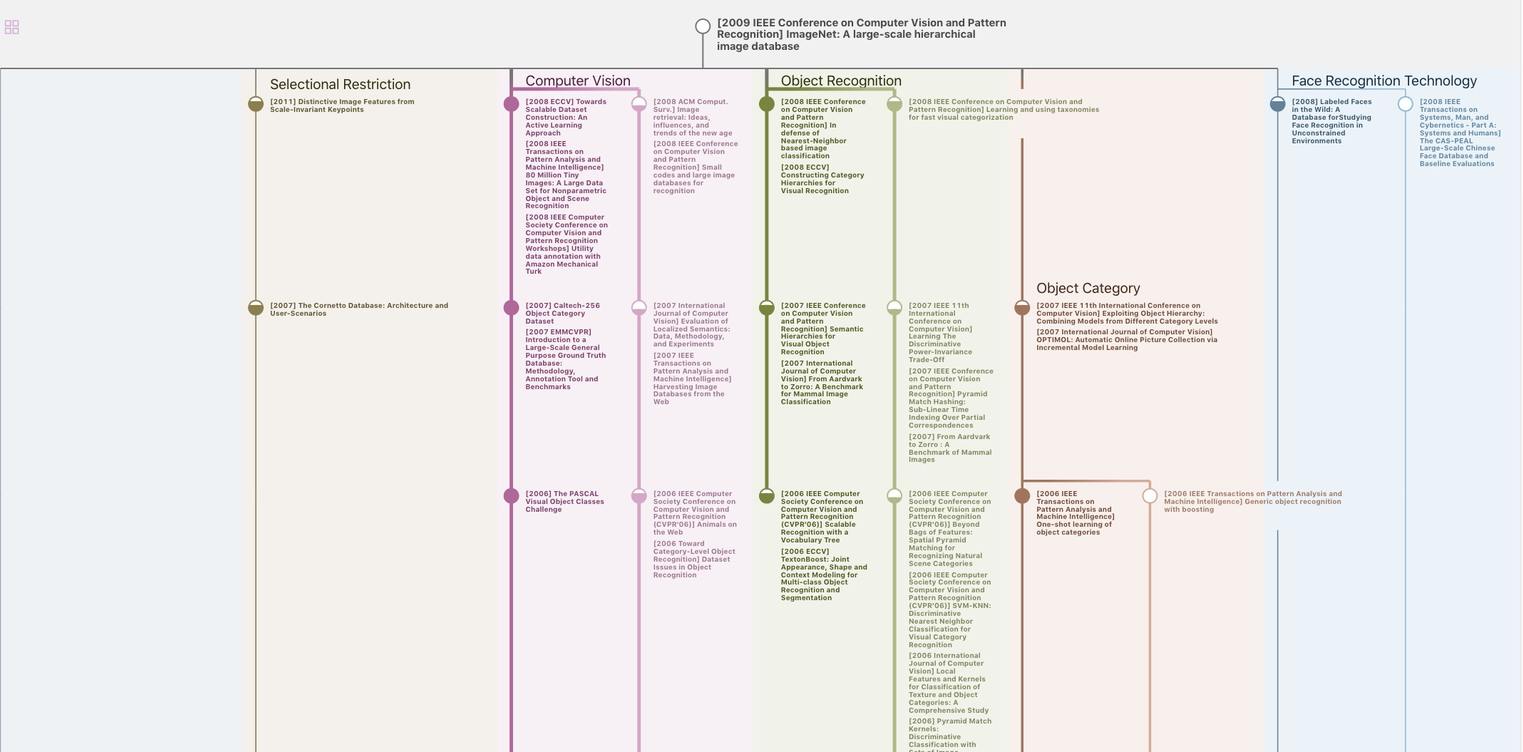
生成溯源树,研究论文发展脉络
Chat Paper
正在生成论文摘要