MINDE: Mutual Information Neural Diffusion Estimation
ICLR 2024(2023)
摘要
In this work we present a new method for the estimation of Mutual Information (MI) between random variables. Our approach is based on an original interpretation of the Girsanov theorem, which allows us to use score-based diffusion models to estimate the Kullback Leibler divergence between two densities as a difference between their score functions. As a by-product, our method also enables the estimation of the entropy of random variables. Armed with such building blocks, we present a general recipe to measure MI, which unfolds in two directions: one uses conditional diffusion process, whereas the other uses joint diffusion processes that allow simultaneous modelling of two random variables. Our results, which derive from a thorough experimental protocol over all the variants of our approach, indicate that our method is more accurate than the main alternatives from the literature, especially for challenging distributions. Furthermore, our methods pass MI self-consistency tests, including data processing and additivity under independence, which instead are a pain-point of existing methods.
更多查看译文
关键词
mutual information,score matching,diffusion models
AI 理解论文
溯源树
样例
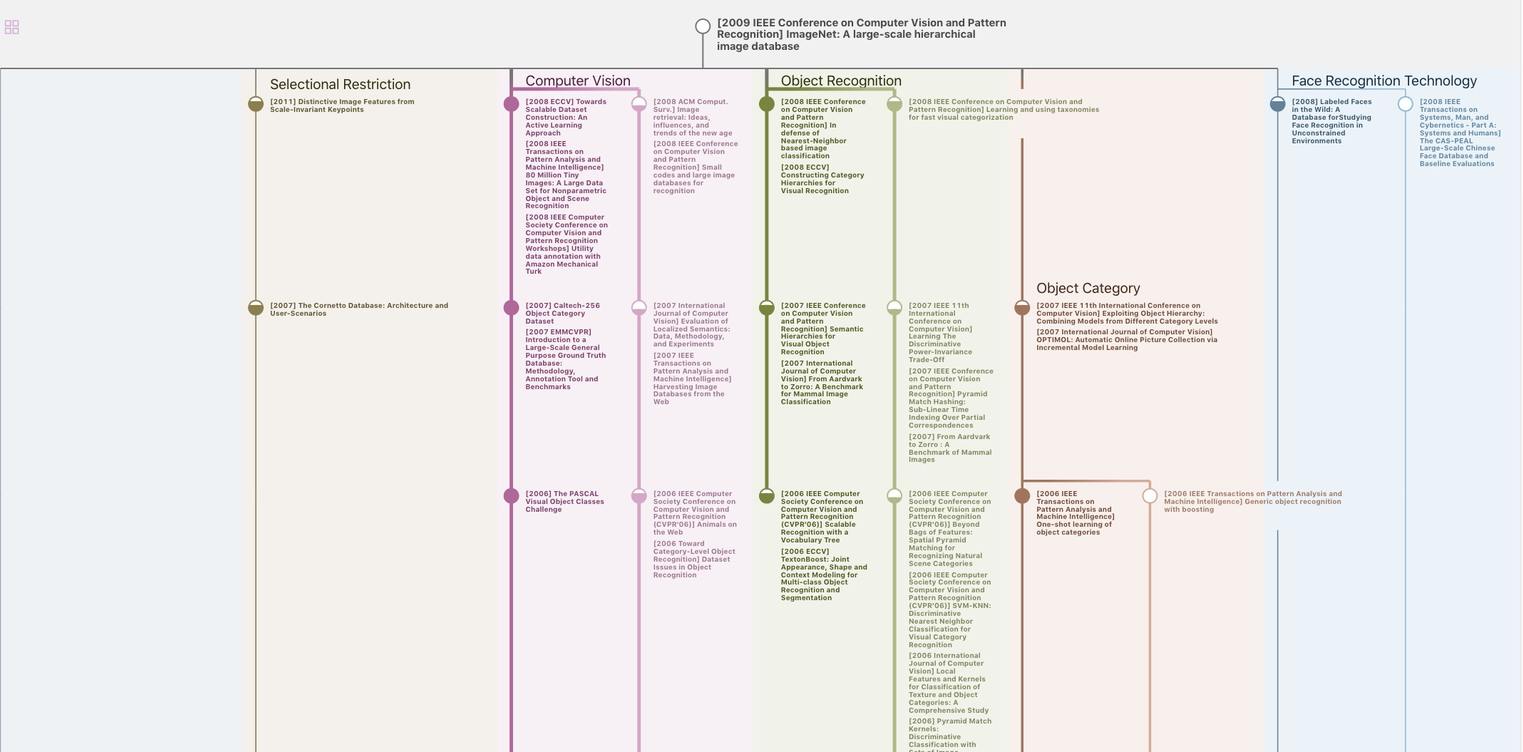
生成溯源树,研究论文发展脉络
Chat Paper
正在生成论文摘要