Stronger Coreset Bounds for Kernel Density Estimators via Chaining
CoRR(2023)
摘要
We apply the discrepancy method and a chaining approach to give improved bounds on the coreset complexity of a wide class of kernel functions. Our results give randomized polynomial time algorithms to produce coresets of size $O\big(\frac{\sqrt{d}}{\varepsilon}\sqrt{\log\log \frac{1}{\varepsilon}}\big)$ for the Gaussian and Laplacian kernels in the case that the data set is uniformly bounded, an improvement that was not possible with previous techniques. We also obtain coresets of size $O\big(\frac{1}{\varepsilon}\sqrt{\log\log \frac{1}{\varepsilon}}\big)$ for the Laplacian kernel for $d$ constant. Finally, we give the best known bounds of $O\big(\frac{\sqrt{d}}{\varepsilon}\sqrt{\log(2\max\{1,\alpha\})}\big)$ on the coreset complexity of the exponential, Hellinger, and JS Kernels, where $1/\alpha$ is the bandwidth parameter of the kernel.
更多查看译文
关键词
kernel density estimators,stronger coreset bounds
AI 理解论文
溯源树
样例
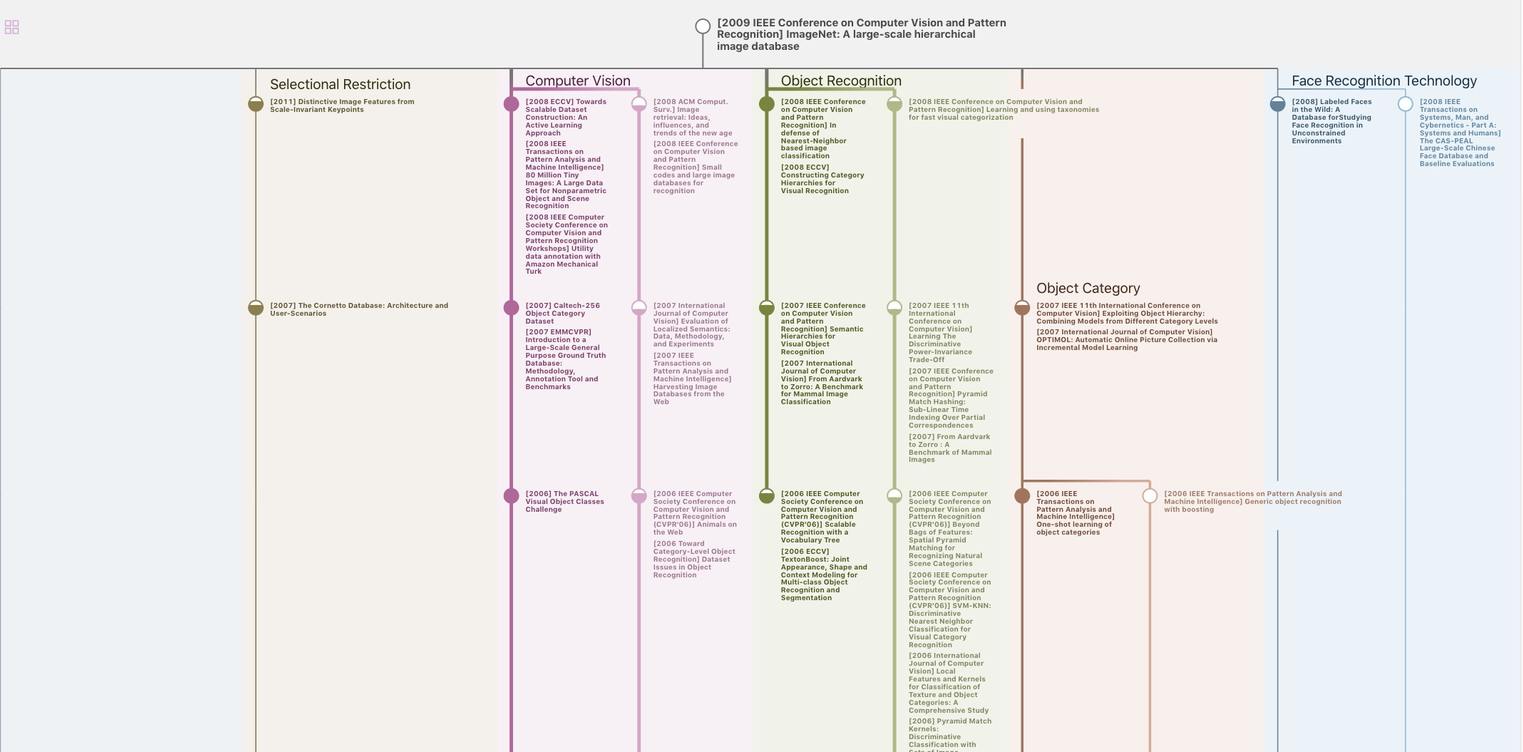
生成溯源树,研究论文发展脉络
Chat Paper
正在生成论文摘要