RaftFed: A Lightweight Federated Learning Framework for Vehicular Crowd Intelligence
CoRR(2023)
摘要
Vehicular crowd intelligence (VCI) is an emerging research field. Facilitated by state-of-the-art vehicular ad-hoc networks and artificial intelligence, various VCI applications come to place, e.g., collaborative sensing, positioning, and mapping. The collaborative property of VCI applications generally requires data to be shared among participants, thus forming network-wide intelligence. How to fulfill this process without compromising data privacy remains a challenging issue. Although federated learning (FL) is a promising tool to solve the problem, adapting conventional FL frameworks to VCI is nontrivial. First, the centralized model aggregation is unreliable in VCI because of the existence of stragglers with unfavorable channel conditions. Second, existing FL schemes are vulnerable to Non-IID data, which is intensified by the data heterogeneity in VCI. This paper proposes a novel federated learning framework called RaftFed to facilitate privacy-preserving VCI. The experimental results show that RaftFed performs better than baselines regarding communication overhead, model accuracy, and model convergence.
更多查看译文
关键词
lightweight federated learning framework,vehicular crowd
AI 理解论文
溯源树
样例
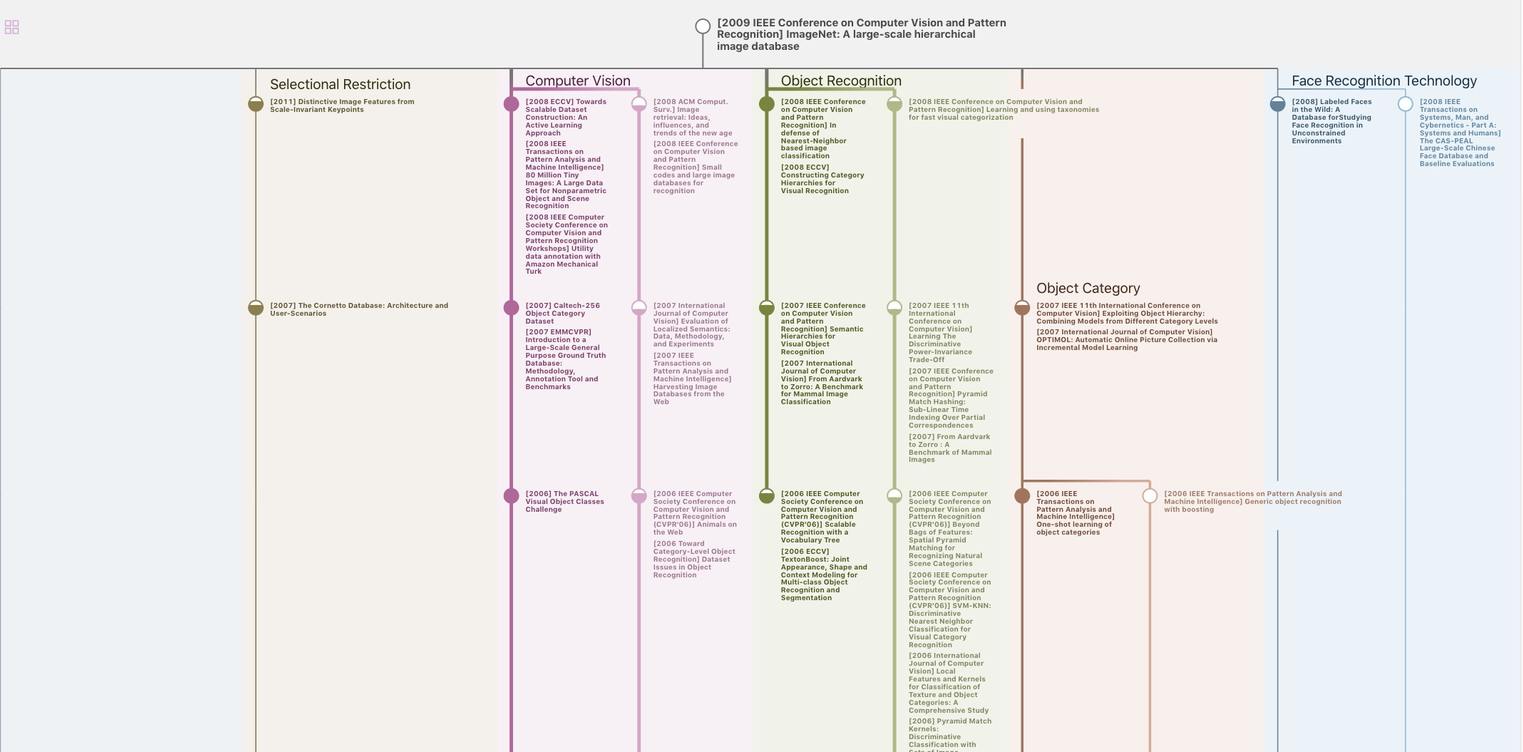
生成溯源树,研究论文发展脉络
Chat Paper
正在生成论文摘要