Assessing the Impact of a Supervised Classification Filter on Flow-based Hybrid Network Anomaly Detection
CoRR(2023)
摘要
Constant evolution and the emergence of new cyberattacks require the development of advanced techniques for defense. This paper aims to measure the impact of a supervised filter (classifier) in network anomaly detection. We perform our experiments by employing a hybrid anomaly detection approach in network flow data. For this purpose, we extended a state-of-the-art autoencoder-based anomaly detection method by prepending a binary classifier acting as a prefilter for the anomaly detector. The method was evaluated on the publicly available real-world dataset UGR'16. Our empirical results indicate that the hybrid approach does offer a higher detection rate of known attacks than a standalone anomaly detector while still retaining the ability to detect zero-day attacks. Employing a supervised binary prefilter has increased the AUC metric by over 11%, detecting 30% more attacks while keeping the number of false positives approximately the same.
更多查看译文
关键词
hybrid network anomaly detection,anomaly detection,supervised classification filter,flow-based
AI 理解论文
溯源树
样例
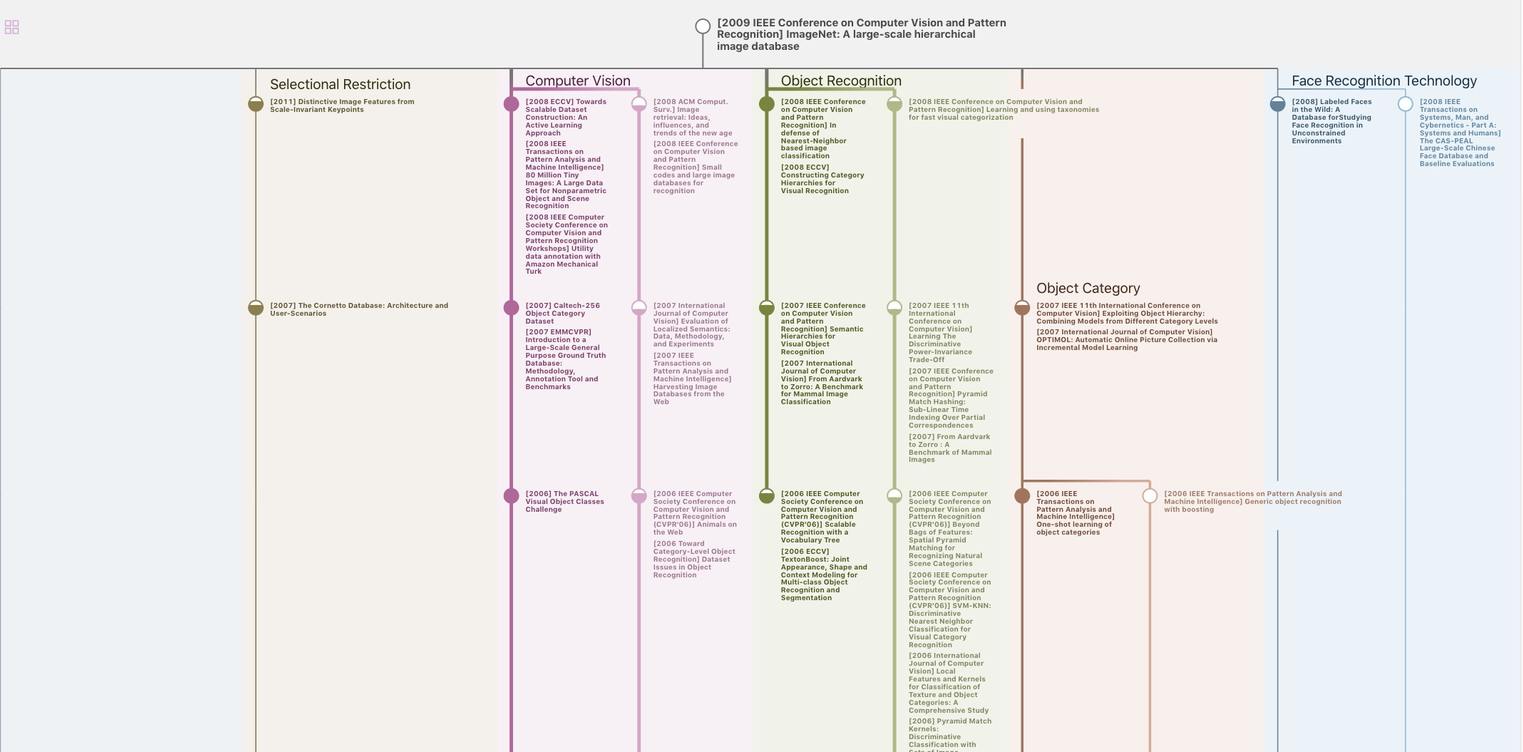
生成溯源树,研究论文发展脉络
Chat Paper
正在生成论文摘要