Florets for Chiplets: Data Flow-aware High-Performance and Energy-efficient Network-on-Interposer for CNN Inference Tasks
ACM Transactions on Embedded Computing Systems(2023)
摘要
Recent advances in 2.5D chiplet platforms provide a new avenue for compact scale-out implementations of emerging compute- and data-intensive applications including machine learning. Network-on-Interposer (NoI) enables integration of multiple chiplets on a 2.5D system. While these manycore platforms can deliver high computational throughput and energy efficiency by running multiple specialized tasks concurrently, conventional NoI architectures have a limited computational throughput due to their inherent multi-hop topologies. In this paper, we propose Floret, a novel NoI architecture based on space-filling curves (SFCs). The Floret architecture leverages suitable task mapping, exploits the data flow pattern, and optimizes the inter-chiplet data exchange to extract high performance for multiple types of convolutional neural network (CNN) inference tasks running concurrently. We demonstrate that the Floret architecture reduces the latency and energy up to 58% and 64%, respectively, compared to state-of-the-art NoI architectures while executing datacenter-scale workloads involving multiple CNN tasks simultaneously. Floret achieves high performance and significant energy savings with much lower fabrication cost by exploiting the data-flow awareness of the CNN inference tasks.
更多查看译文
关键词
chiplets,flow-aware,high-performance,energy-efficient,network-on-interposer
AI 理解论文
溯源树
样例
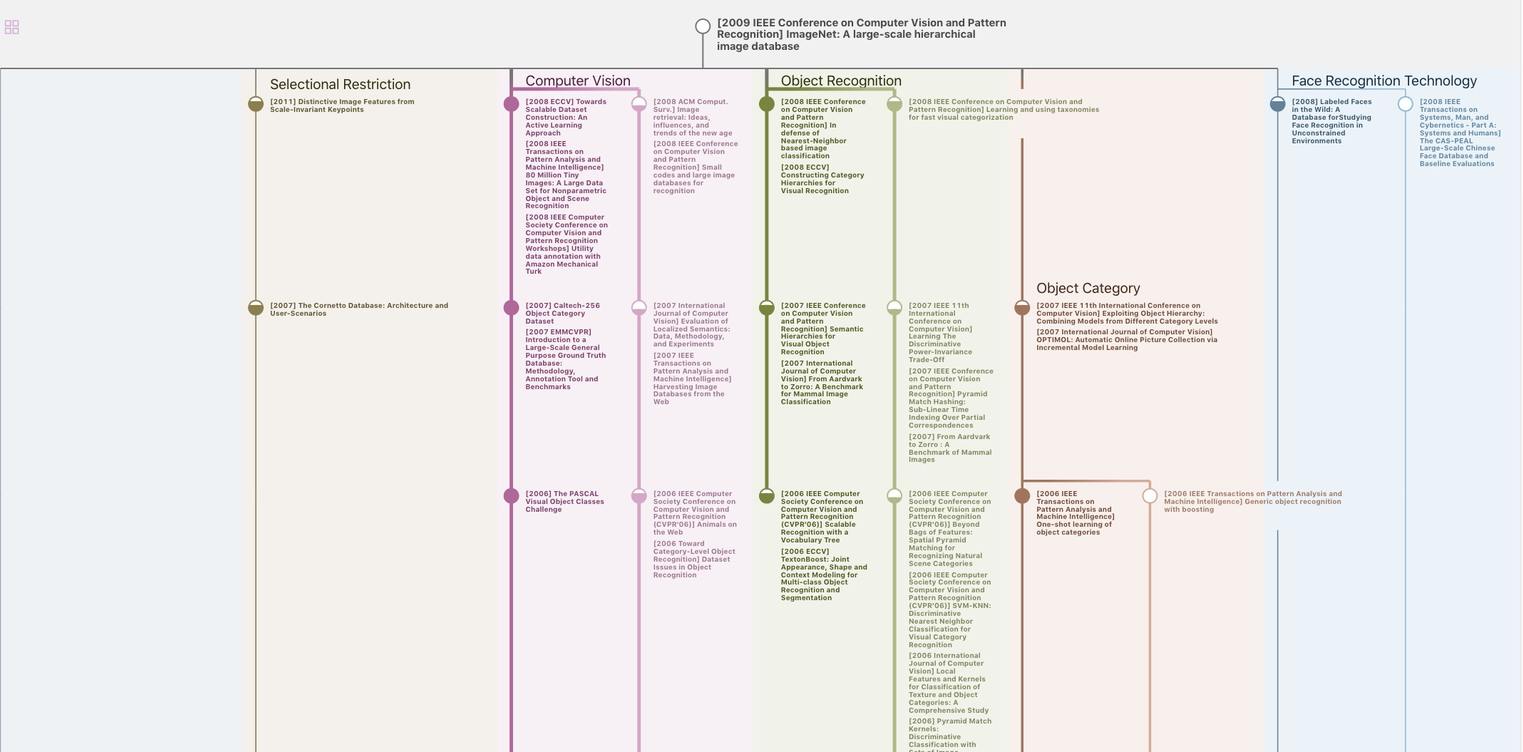
生成溯源树,研究论文发展脉络
Chat Paper
正在生成论文摘要