MOON: A Subspace-Based Multi-Branch Network for Object Detection in Remotely Sensed Images
REMOTE SENSING(2023)
摘要
The effectiveness of training-based object detection heavily depends on the amount of sample data. But in the field of remote sensing, the amount of sample data is difficult to meet the needs of network training due to the non-cooperative imaging modes and complex imaging conditions. Moreover, the imbalance of the sample data between different categories may lead to the long-tail problem during the training. Given that similar sensors, data acquisition approaches, and data structures could make the targets in different categories possess certain similarities, those categories can be modeled together within a subspace rather than the entire space to leverage the amounts of sample data in different subspaces. To this end, a subspace-dividing strategy and a subspace-based multi-branch network is proposed for object detection in remotely sensed images. Specifically, a combination index is defined to depict this kind of similarity, a generalized category consisting of similar categories is proposed to represent the subspace, and a new subspace-based loss function is devised to address the relationship between targets in one subspace and across different subspaces to integrate the sample data from similar categories within a subspace and to balance the amounts of sample data between different subspaces. Furthermore, a subspace-based multi-branch network is constructed to ensure the subspace-aware regression. Experiments on the DOTA and HRSC2016 datasets demonstrated the superiority of our proposed method.
更多查看译文
关键词
object detection,long-tail problem,generalized category,subspace-based multi-branch network,remotely sensed image
AI 理解论文
溯源树
样例
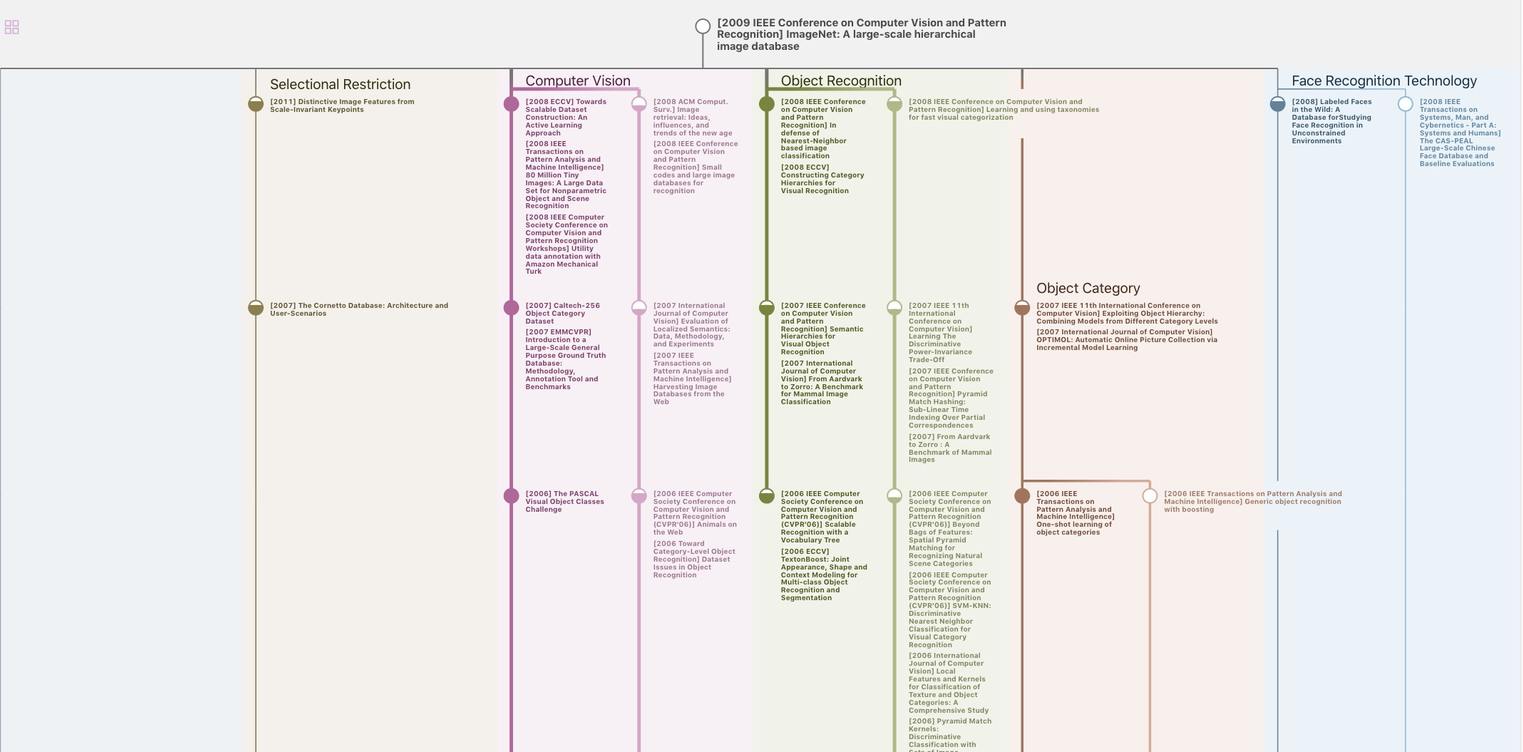
生成溯源树,研究论文发展脉络
Chat Paper
正在生成论文摘要