Clustered Distributed Learning Exploiting Node Centrality and Residual Energy (CINE) in WSNs.
Network(2023)
摘要
With the explosion of big data, the implementation of distributed machine learning mechanisms in wireless sensor networks (WSNs) is becoming required for reducing the number of data traveling throughout the network and for identifying anomalies promptly and reliably. In WSNs, the above need has to be considered along with the limited energy and processing resources available at the nodes. In this paper, we tackle the resulting complex problem by designing a multi-criteria protocol CINE that stands for “Clustered distributed learnIng exploiting Node centrality and residual Energy” for distributed learning in WSNs. More specifically, considering the energy and processing capabilities of nodes, we design a scheme that assumes that nodes are partitioned in clusters and selects a central node in each cluster, called cluster head (CH), that executes the training of the machine learning (ML) model for all the other nodes in the cluster, called cluster members (CMs). In fact, CMs are responsible for executing the inference only. Since the CH role requires the consumption of more resources, the proposed scheme rotates the CH role among all nodes in the cluster. The protocol has been simulated and tested using real environmental data sets.
更多查看译文
关键词
residual energy,cine,learning
AI 理解论文
溯源树
样例
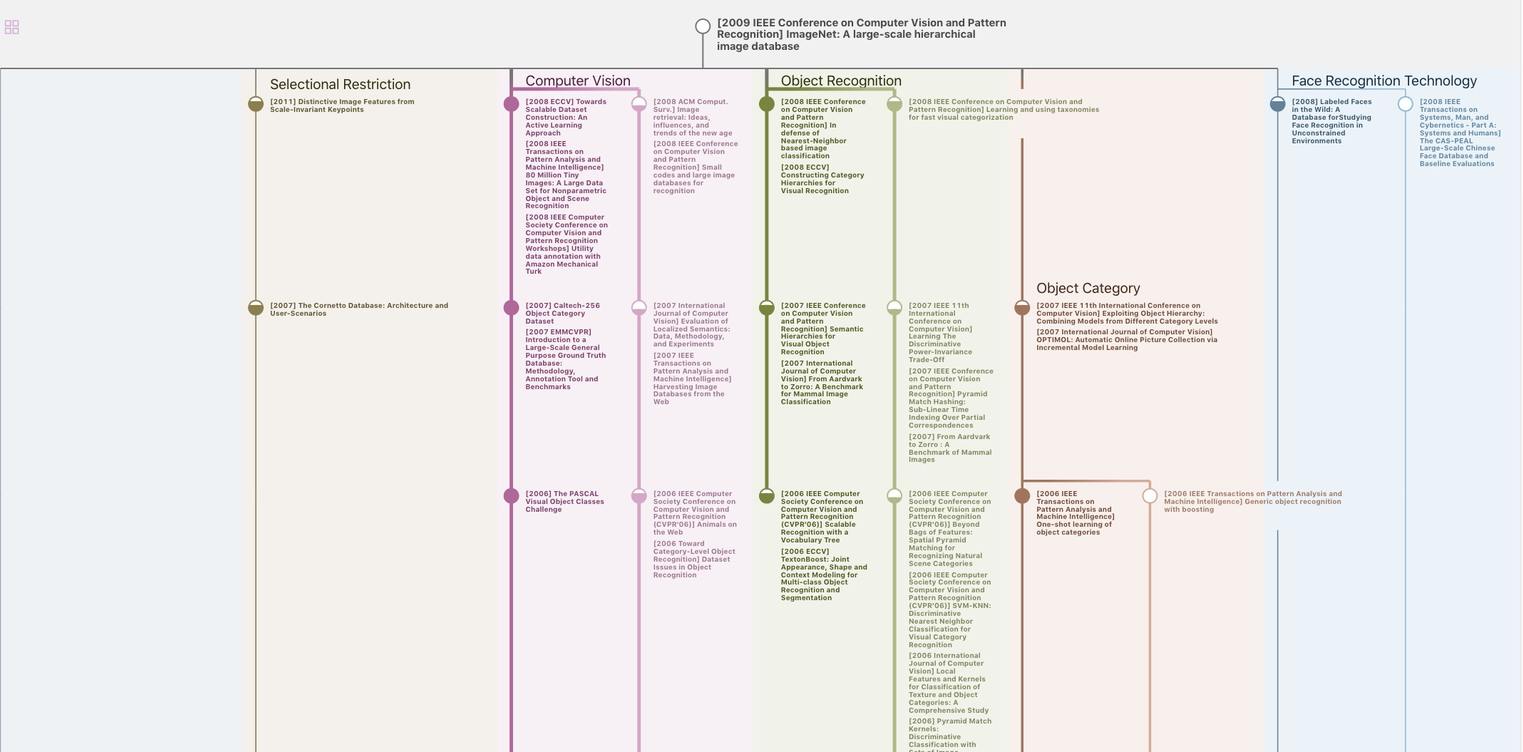
生成溯源树,研究论文发展脉络
Chat Paper
正在生成论文摘要