Self-Training with High-Dimensional Markers for Cell Instance Segmentation.
ISBI(2023)
摘要
Cellular segmentation is a fundamental prerequisite to many biological analyses. With the development of multiplexed imaging technologies, the need for accurately segmenting individual cells has significantly increased in recent years. However, current deep learning methods cannot deal with staining markers in an arbitrary order or different numbers. Moreover, acquiring pixel-level annotation is incredibly time-consuming in high-dimensional images. To tackle these issues, we incorporate pathology knowledge into our model and present a novel self-training framework. Concretely, we apply a serial attention mechanism and pooling operation to compress the multi-channel image during the training process. Afterward, the nuclei information guides the self-training in the pseudo-label stage. Experiments demonstrate our method is superior to the existing methods in both qualitative and quantitative results.
更多查看译文
关键词
Cell segmentation, deep learning, self-training, highly-multiplexed imaging, CODEX
AI 理解论文
溯源树
样例
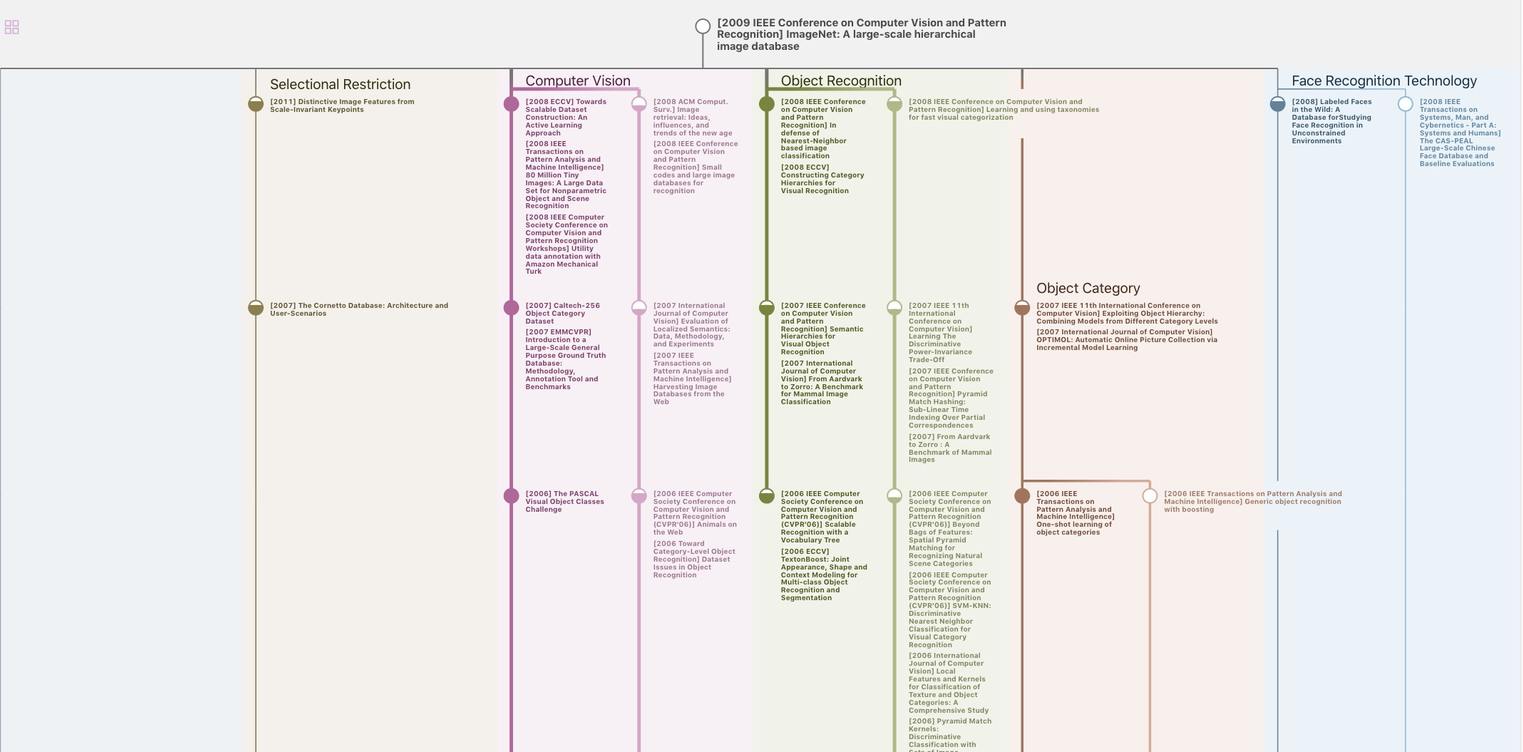
生成溯源树,研究论文发展脉络
Chat Paper
正在生成论文摘要