WELID: A Weighted Ensemble Learning Method for Network Intrusion Detection.
ISCC(2023)
摘要
The requirements for intrusion detection technology are getting higher and higher, with the rapid expansion of network applications. There have been many studies on intrusion detection, however, the accuracy of these models is not high enough and time-consuming, making them unavailable. In this paper, we propose a novel weighted ensemble learning method for network intrusion detection (WELID). Firstly, data preprocessing and feature selection algorithms are used to filter out some redundant and unrelated features. Next, anomaly detection is performed on the dataset using different base classifiers, and a layered ten-fold cross-validation method is used to prevent program overfitting. Then, the best classifiers are selected for the use of a multi-classifier fusion algorithm based on probability-weighted voting. We compare the proposed model with lots of efficient classifiers and state-of-the-art models for intrusion detection. The results show that the proposed model is superior to these models in terms of accuracy and time consumption.
更多查看译文
关键词
weighted ensemble learning method,detection
AI 理解论文
溯源树
样例
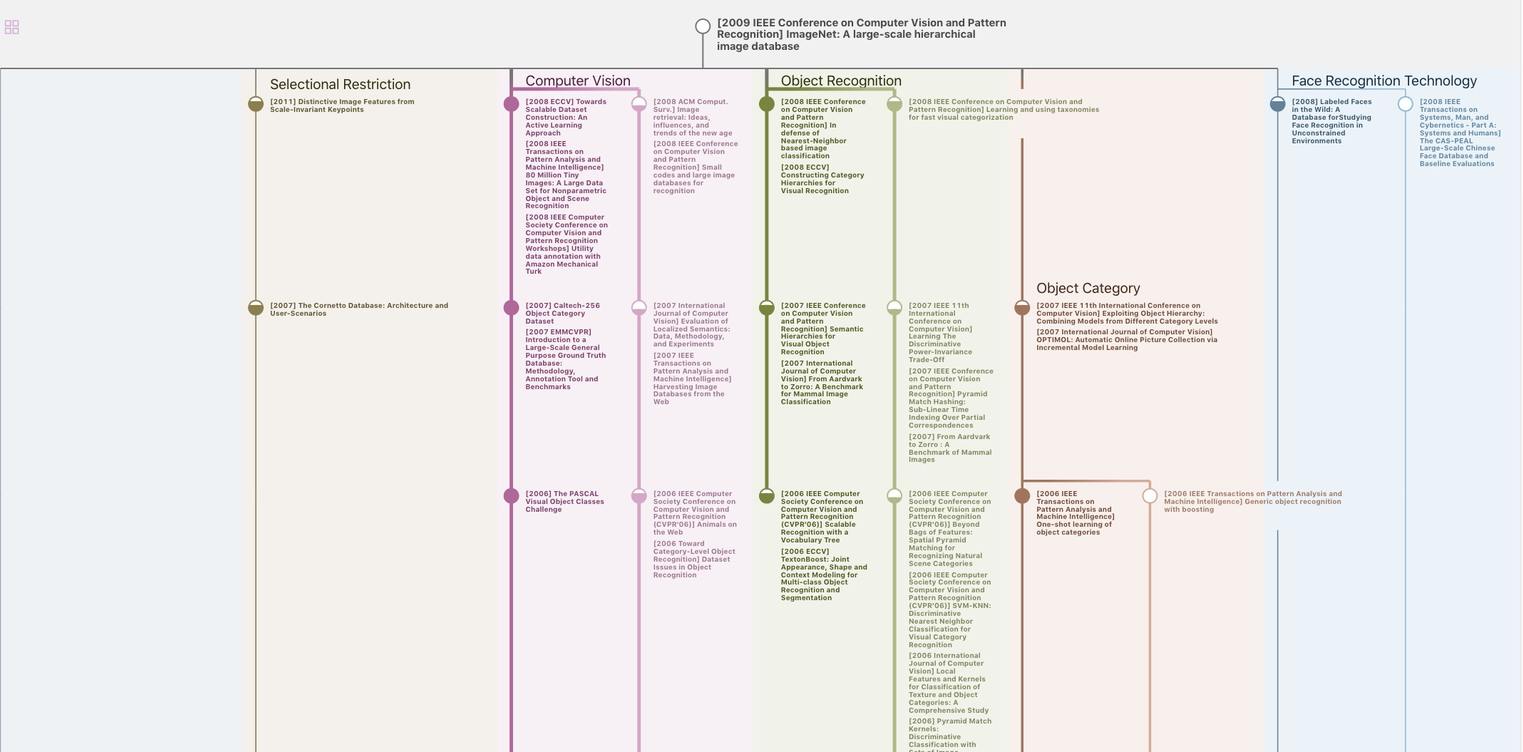
生成溯源树,研究论文发展脉络
Chat Paper
正在生成论文摘要