Deep Reinforcement Learning-Based SFC Deployment Scheme for 6G IoT Scenario.
ISCC(2023)
摘要
To meet the extremely low latency requirements of 6G Internet of Things (IoT) services, 6G network should be able to intelligently allocate the network resources. Based on Mobile edge computing (MEC) and network function virtualization (NFV), the 6G NFV/MEC-enabled IoT architecture will be a viable architecture to enable flexible and efficient resource allocation. The architecture will enable the deployment of service function chains (SFCs) in NFV-enabled network edge nodes. However, due to the heterogeneous and dynamic nature of 6G IoT, it is a challenge to deploy SFCs rationally. Therefore, this paper proposes a knowledge-assisted deep reinforcement learning (KADRL) based SFC deployment scheme. The scheme achieves flexible and efficient resource allocation by deploying SFCs at appropriate edge nodes for the requirements of 6G IoT services. Simulation results demonstrate that KADRL can achieve better convergence performance and can meet the requirements of delay-sensitive IoT services.
更多查看译文
关键词
6G,IoT,SFC deployment,Deep reinforcement learning
AI 理解论文
溯源树
样例
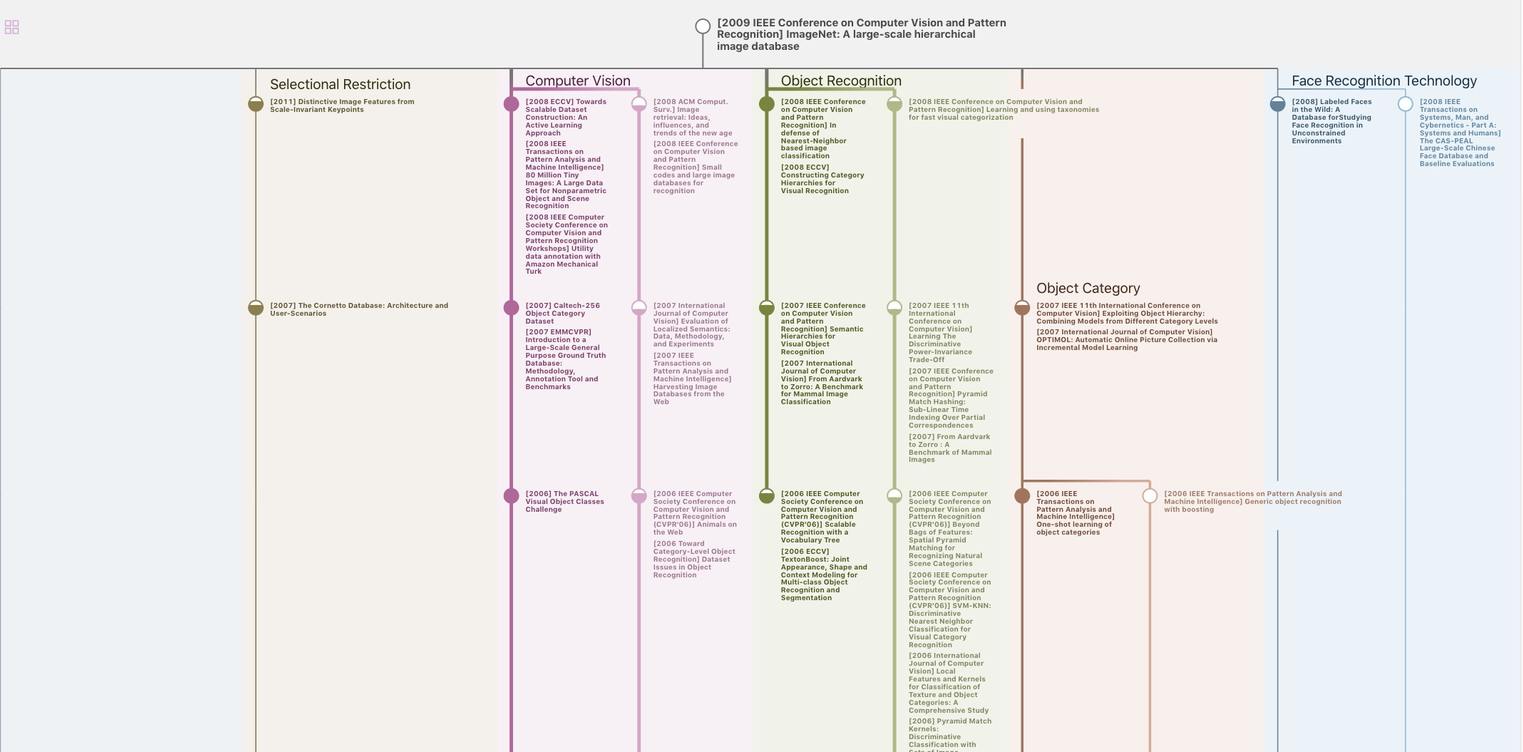
生成溯源树,研究论文发展脉络
Chat Paper
正在生成论文摘要