FEAT: Towards Fast Environment-Adaptive Task Offloading and Power Allocation in MEC.
INFOCOM(2023)
摘要
Mobile edge computing (MEC) has been proposed to provide mobile devices with both satisfactory computing resources and latency. Key issues in MEC include task offloading and power allocation (TOPA), for which deep reinforcement learning (DRL) is becoming a popular methodology. However, most DRL-based TOPA approaches are typically developed in a certain environment, lacking the adaptability to unseen environments. Motivated by this, this paper proposes a Fast Environment-Adaptive TOPA (FEAT) approach that could adapt to unseen environments with little fine-tuning. Specifically, we first split MEC states into the internal state and environmental state. Then, based on these two types of states, we develop two main components of FEAT — a group of internal state-dependent TOPA meta-policies and an environmental state-embedded steerer. Meta-policies learn TOPA skills within the internal state space (allowing reusing meta-policies in different environments), while the steerer learns to choose appropriate meta-policies according to embedded environmental states. When encountering an unseen environment with the same internal state space, FEAT only needs to fine-tune the steerer using the newly embedded environmental state with few internal state explorations. Extensive experimental results on simulation and testbed demonstrate that FEAT outperforms the state-of-the-art by more than 16.4% in terms of fine-tuning speeds.
更多查看译文
关键词
Mobile Edge Computing,Task Offloading,Power Allocation,Environment Adaptation
AI 理解论文
溯源树
样例
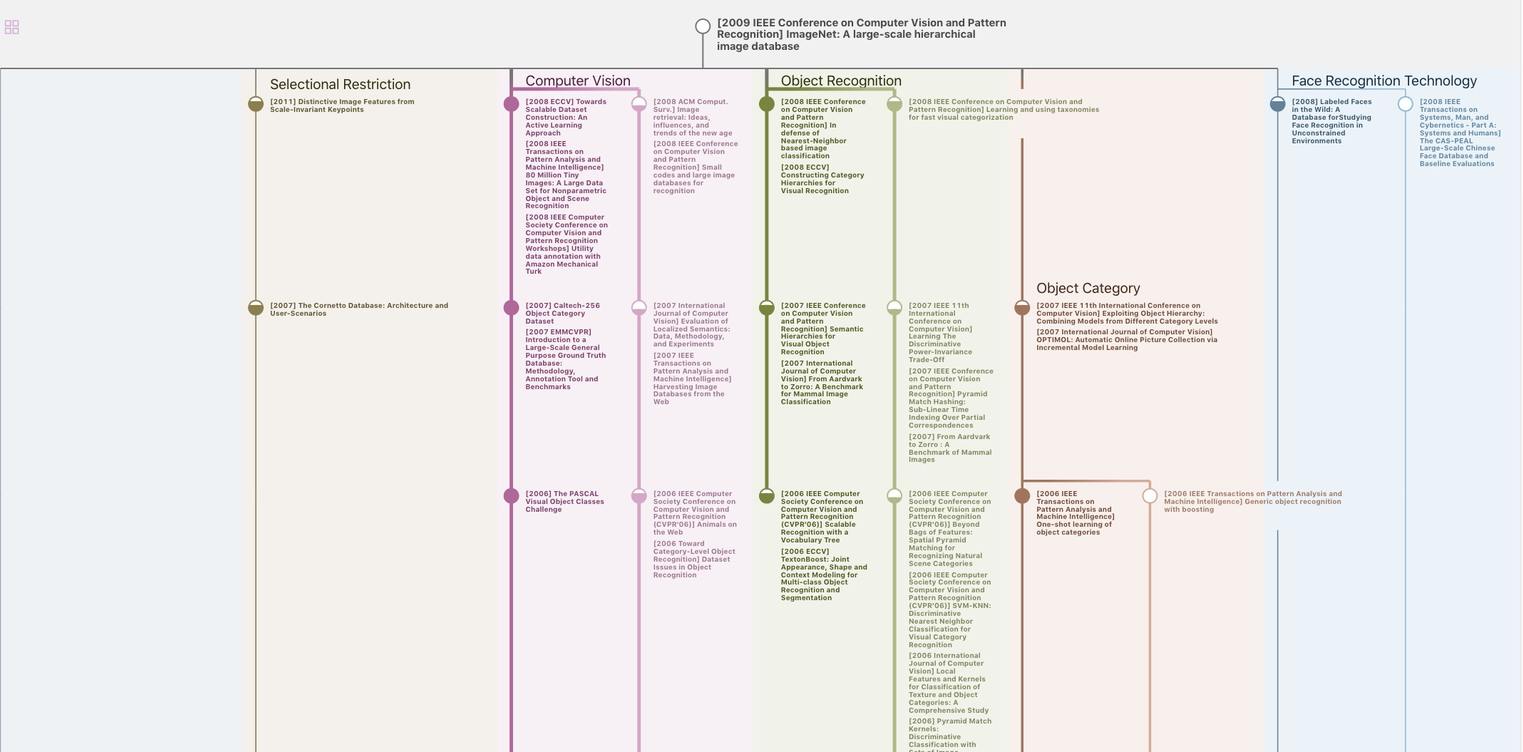
生成溯源树,研究论文发展脉络
Chat Paper
正在生成论文摘要