ReRAM-based graph attention network with node-centric edge searching and hamming similarity.
DAC(2023)
摘要
The graph attention network (GAT) has demonstrated its advantages via local attention mechanism but suffered from low energy and latency efficiency when implemented on conventional von-Neumann hardware. This work proposes and experimentally demonstrates an algorithm-hardware co-designed GAT that runs efficiently and reliably in ReRAM-based hard-ware. The neighborhood information is retrieved from trained node embeddings stored on crossbars in a single time step, and attention is implemented by efficient hashing and hamming similarity for higher robustness. Our scaled simulation based on the experimentally-validated model shows only 0.9% accuracy loss with over 35,500x energy improvement on the Cora dataset compared with GPU, and 1.1% accuracy improvement with 2x energy improvement compared with state-of-the-art ReRAM-based GNN accelerator.
更多查看译文
关键词
graph, graph attention network, sparse, ReRAM, memristor, crossbar array
AI 理解论文
溯源树
样例
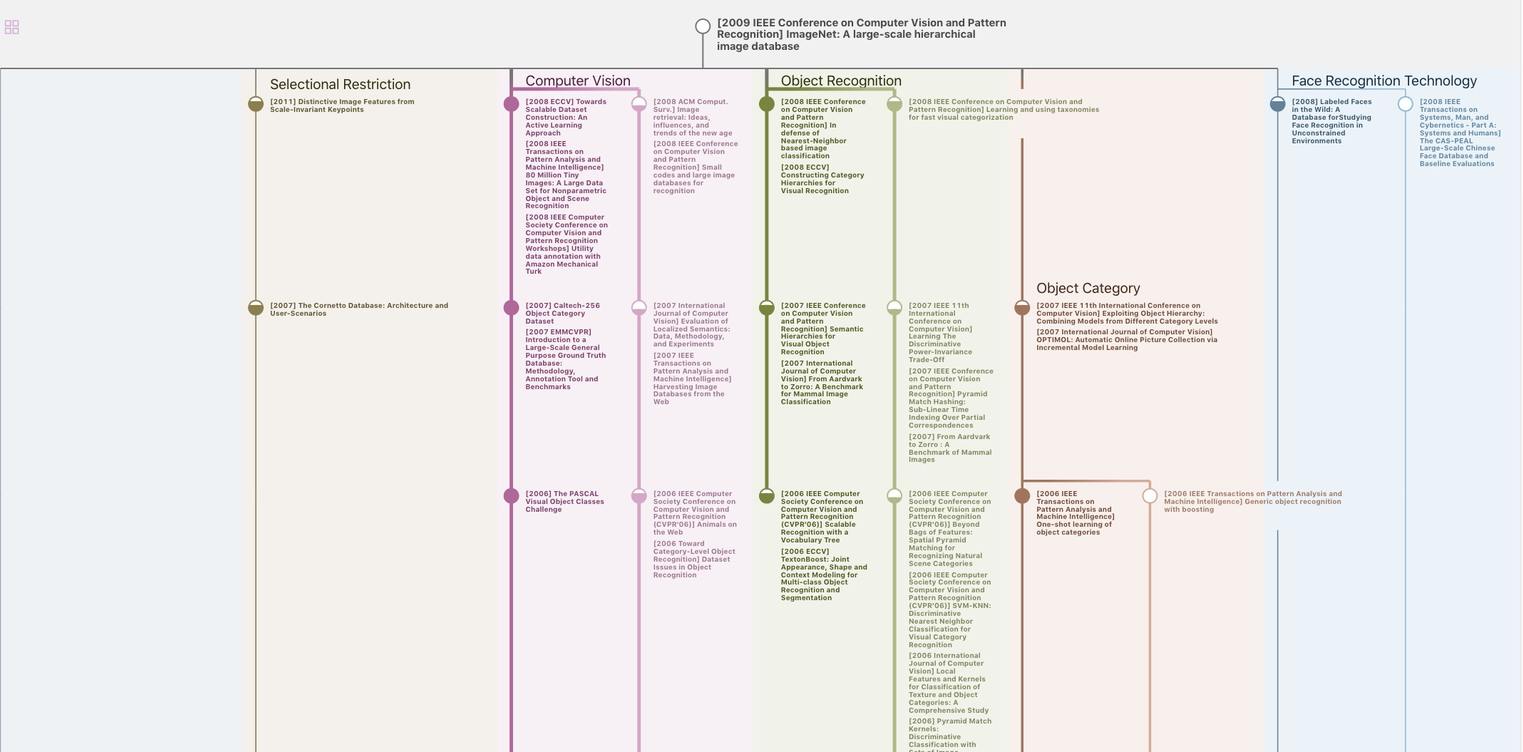
生成溯源树,研究论文发展脉络
Chat Paper
正在生成论文摘要